Programme
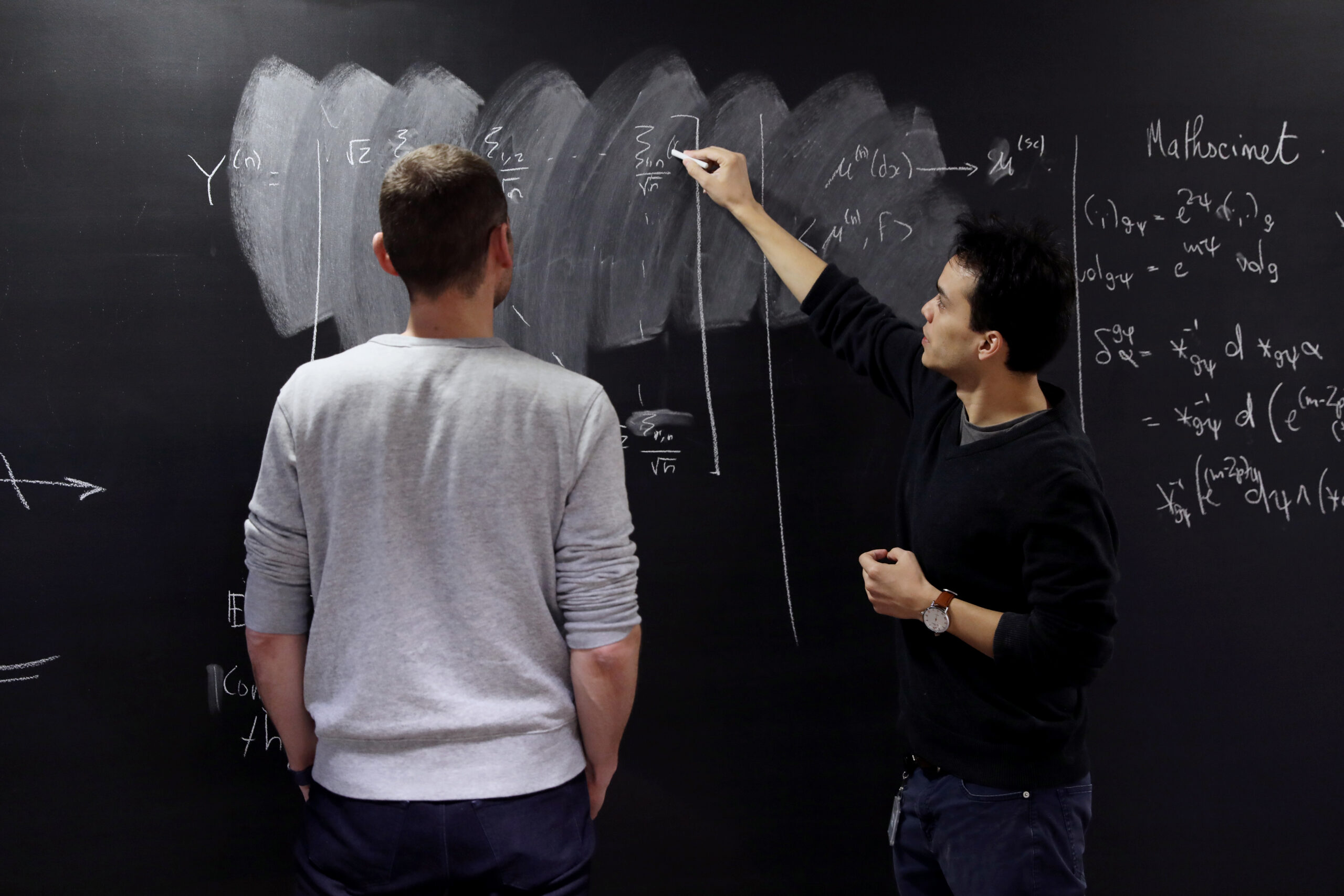
The Financial Mathematics track of the Master in Mathematics is a two-year programme taught in English. To boost their future career, the track includes internships and allows students to write their Master thesis in a professional setting during an internship. New students are requested to take the Refresher Courses scheduled before the start of the first semester.
Academic Contents
Course offer for Financial Mathematics, Semestre 1 (2024-2025 Winter)
-
Details
- Course title: Discrete-time stochastic processes
- Number of ECTS: 6
- Course code: F1_MA_MAT_FM-1
- Module(s): Financial Mathematics 1
- Language: EN
- Mandatory: Yes
-
Objectives
Introduction to basic concepts of modern probability theory
-
Course learning outcomes
On successful completion of the course, the student should be able to:
- Understand and use concepts of modern probability theory (e.g., filtrations, martingales, stopping times)
- Apply the notion of martingale to model random evolutions
- Know and apply classical martingale convergence theorems
- Describe and manipulate basic properties of Brownian motion
-
Description
Radon-Nikodym Theorem, conditional expectations, martingales, stopping times, optional stopping theorems, Doob’s inequalities, martingale convergence theorems, martingale central limit theorem, Brownian motion. -
Assessment
Exam modalities for the first session
Combined Assessment (40% Homeworks, 60% Written In-person Final Exam).
Exam modalities for the retake exam
Combined Assessment (40% Homeworks, 60% Written In-person Final Exam).
Absence Plan
In the case of justified absence in the final exam a replacement exam during the same semester will be offered. -
Note
- Rick Durrett: Probability Theory and Examples
- Yuval Peres, Peter Morters: Brownian Motion
-
Details
- Course title: Functional Analysis
- Number of ECTS: 6
- Course code: F1_MA_MAT_MMCS2-2
- Module(s): Financial Mathematics 1
- Language: EN
- Mandatory: No
-
Objectives
The aim of the course is to provide students with efficient tools to study linear operators between infinite dimensional vector spaces. In particular, this class will deal with normed vector spaces, Banach and Hilbert spaces, with bounded linear operators on normed vector spaces, fundamental principles such as Fourier analysis, Lebesgue integral, Han-Banach Theorem, Uniform Boundedness Principle or Closed Graph Theorem, and with spectral theory of compact (self-adjoint) linear operators.
-
Course learning outcomes
Students will acquire a solid understanding of functional analysis, its fundamental results and basic techniques. In particular, students will understand applications to measure theory, Fourier theory, and the spectral theorem for (unbounded) operators. Students will know the relevance of a theorem, its underlying motivation and a precise idea of its proof. Hopefully, students will demonstrate capacity for mathematical reasoning through analyzing, proving and explaining concepts from functional analysis. -
Description
Functional analysis aims to study infinite-dimensional spaces of functions and features of linear operators on these spaces. Though, from the point of view of Mathematics, functional analysis has its own interest, it plays a crucial role in many related areas, in particular in Physics, Engineering or Finance. Roughly speaking, most “real-life problems” involve non-linear partial differential equations with infinite-dimensional solution spaces of functions (or distributions). This course provides Master students with the basic tools and the fundamental results to develop skills to solve such problems. -
Assessment
Exam modality for the first session- Written test on week of October 28, duration 1 hour, 30% of the final grade,
- Final written exam (scheduled by the administration), 60% of the final grade,
- Written homework (problems to be determined), 10% of the final grade.
Exam modality for the retake exam
Retake exam (scheduled by the administration) will be in written form.
Absence
A make-up will be possible only for an unavoidable reason supported by a proof. -
Note
Note / Literature / Bibliography
Introductory Functional Analysis with Applications, by Erwin Kreyszig.
Functional Analysis, Spectral Theory and Applications, by Manfred Einsiedler and Thomas Ward.
An Introduction to Fourier Analysis, by Russell L. Herman
-
Details
- Course title: Riemannian Geometry
- Number of ECTS: 8
- Course code: F1_MA_MAT_GM-2
- Module(s): Financial Mathematics 1
- Language: EN
- Mandatory: No
-
Course learning outcomes
On successful completion of the course, the student should be able to:
- compute with tensors, such as metrics and curvature, in coordinates or coordinate-free
- demonstrate detailed knowledge of the exponential map, including criteria for completeness, conjugate points, and Jacobi fields
- understand multiple interpretations of curvature, for example, as the obstruction to a local parallel framing, or how it influences the spreading of geodesics via the Jacobi equation
-
Description
This foundational Master-level course is centered around the concepts of connection on a manifold and curvature. These are investigated in further depth in the setting of Riemannian or Lorentzian metrics, where the geometry of geodesics and its relation to curvature are studied.
-
Assessment
Oral or written exam -
Note
Literatur- M. P. do Carmo, Riemannian Geometry, Birkhäuser (1992)
- B. O’Neill, Semi-Riemannian Geometry with applications to Relativity, Elsevier (1983)
- John M. Lee, Introduction to Smooth Manifolds (2nd edition), Springer (2012)
-
Details
- Course title: Stochastic Analysis
- Number of ECTS: 6
- Course code: F1_MA_MAT_FM-2
- Module(s): Financial Mathematics 1
- Language: EN
- Mandatory: Yes
-
Objectives
Introduction to basic concepts of Stochastic Analysis.
-
Description
Continuous martingales, stochastic integration, quadratic variation, Itô calculus, theorem of Girsanov, stochastic differential equations, Markov property of solutions, connection of stochastic differential equations and partial differential equations, martingale representation theorems, chaotic expansions, Feynman-Kac formula. -
Assessment
Written exam -
Note
- I. Karatzas, S. Shreve: Brownian motion and stochastic calculus. 2nd edition. Springer, 1991
- B. Oksendal: Stochastic differential equations. Springer, 2003
- D. Revuz, M. Yor: Continuous Martingales and Brownian Motion. Springer Grundl., 1999
-
Details
- Course title: Partial Differential Equations I
- Number of ECTS: 7
- Course code: F1_MA_MAT_GM-3
- Module(s): Financial Mathematics 1
- Language: EN
- Mandatory: No
-
Objectives
The goal of the course it to get acquainted with Partial differential equations (PDE) as a powerful tool for modeling problems in science, providing functional analytic techniques in order to deal with PDE.
-
Course learning outcomes
On successful completion of the course the student should be able to:
- Apply methods of Fourier Analysis to the discussion of constant coefficient differential equations
- Work freely with the classical formulas in dealing with boundary value problems for the Laplace equation
- Prove acquaintance with the basic properties of harmonic functions (maximum principle, mean value property) and solutions of the wave equation (Huygens property)
- Solve Cauchy problems for the heat and the wave equations
- Give a pedagogic talk for peers on a related topic
-
Description
Fourier transform, the classical equations, spectral theory of unbounded operators, distributions, fundamental solutions. -
Assessment
Written exam -
Note
Literatur- Rudin: Functional analysis
- Jost: Postmodern analysis
- Folland: Introduction to partial differential equations.
- Reed-Simon: Methods of mathematical physics I-IV
-
Details
- Course title: Probabilistic Models in Finance
- Number of ECTS: 6
- Course code: F1_MA_MAT_FM-3
- Module(s): Financial Mathematics 1
- Language: EN
- Mandatory: Yes
-
Objectives
Introductory course to basic concepts of Mathematical Finance, also suitable for students who are not going to choose their specialization in Finance. The goal is to deepen the knowledge of modern probability theory by studying applications of general interest in an actual field of applied mathematics.
-
Course learning outcomes
On successful completion of the course, the student should be able to:
- Derive and apply formulas for option pricing and hedging strategies
- Carry out calculations based on arbitrage arguments
- Calculate the price of European call and put options using the Cox, Ross and Rubinstein model
- Apply the techniques of Snell envelopes to evaluate American options
- Derive the classical Black-Scholes formulas as limiting case of a sequence of CRR markets
-
Description
Discrete financial markets, the notion of arbitrage, discrete martingale theory, martingale transforms, complete markets, the fundamental theorem of asset pricing, European and American options, hedging strategies, optimal stopping, Snell envelopes, the model of Cox, Ross and Rubinstein. -
Assessment
Written exam -
Note
Literatur- D. Lamberton, B. Lapeyre: Introduction au calcul stochastique appliqué à la finance. Ellipses, 1997
- S. E. Shreve: Stochastic calculus for finance. I: The binomial asset pricing model. Springer Finance, 2004
- H. Föllmer, A. Schied: Stochastic finance. An introduction in discrete time. 2nd ed., de Gruyter, 2004
- F. Delbaen, W. Schachermayer: The mathematics of arbitrage. Springer Finance, 2006
-
Details
- Course title: Scientific Python
- Number of ECTS: 1
- Course code: F1_MA_MAT_MMCS2-4
- Module(s): Financial Mathematics 1
- Language: EN
- Mandatory: No
-
Objectives
- Introduction
- Getting started
- Basics of Python
- Array computations with numpy
- Array computations with numpy (cont.)
- Plotting with matplotlib
- Tabular data manipulation with pandas
- Tabular data manipulation with pandas (cont.)
- Writing good quality and robust Python code
-
Course learning outcomes
First sessionYou should feel comfortable writing basic scientific programs in Python, and be able to participate fully in future courses that require an element of programming.
Retake exam
Retake exam not possible, course must be retaken. -
Description
This course covers the basics of scientific programming with Python. It is aimed at people who have done some programming before, perhaps on an undergraduate course, but need a refresher before starting their Masters or Doctoral degrees at the University.
https://jhale.github.io/scientific-python/ -
Assessment
A coursework will be distributed at the end of the class. To pass the course and receive the ECTS credits you must complete the coursework.
-
Details
- Course title: Introduction to Graph Theory
- Number of ECTS: 3
- Course code: MA_DS-8
- Module(s): Financial Mathematics 1
- Language: EN
- Mandatory: No
-
Objectives
On successful completion of the course the students should be able to
- understand the relevance of the topics covered in the course for their applications,
- master the proofs of the main results of the course,
- solve problems using the toolkit developed in the course
- be autonomous in learning in the field of Graph Theory.
-
Description
Through a presentation of selected topics, the course aims to be an introduction to graph theory, its applications and its algorithmic aspects. It is designed as a self-contained course and focused on problems pertaining to Data Science.
Possible topics for the course include, but are not limited to
- Graphs and digraphs, degree and the degree sequence algorithm
- Connectedness, distance, shortest paths and connected components algorithms
- Graph matching problems and algorithms
- Elements of algebraic graph theory and PageRank algorithm
- Graph traversal algorithms
- Trees and applications
- Minimum spanning tree algorithms
- Network flow, min cut – max flow theorem and Ford–Fulkerson algorithm
- Centrality and betweness measures
- Cluster analysis
- Random Graphs
-
Assessment
Written exam and homework, and possibly algorithm implementation project during the semester.
First session
Retake exam
Writen exam -
Note
Note / Literature / Bibliography- R. Diestel, Graph Theory, Springer, 2017
- D. Jungnickel, Graphs, Networks and algorithms, Springer 2017
-
Details
- Course title: Mathematical Modelling
- Number of ECTS: 5
- Course code: F1_MA_MAT_MMCS2-3
- Module(s): Financial Mathematics 1
- Language: EN
- Mandatory: No
-
Course learning outcomes
By the end of the course, students are expected to:
- Understand the principles of mathematical modeling and its applications, including agent-based methods, in diverse fields.
- Demonstrate proficiency in selecting appropriate mathematical techniques, including agent-based modeling, for specific problems.
- Develop the ability to translate real-world scenarios into mathematical equations, agent-based models, and simulations.
- Gain proficiency in using computational tools and programming languages, including agent-based modeling platforms, for simulations and analysis.
- Apply critical thinking to interpret and validate results from mathematical models and agent-based simulations in practical contexts.
- Collaborate effectively in group projects involving mathematical modeling and agent-based simulations.
- Communicate complex mathematical concepts, agent-based modeling principles, and findings to both technical and non-technical audiences.
-
Description
This graduate-level course offers an in-depth exploration of mathematical modeling and computational techniques, including agent-based methods, applied to real-world problems across various disciplines. Through a blend of theoretical foundations and practical applications, students will develop the skills necessary to formulate, analyze, and solve complex problems using mathematical approaches, agent-based modeling, and computational tools.
Course Topics
1. Introduction to Mathematical Modeling and Agent-Based Methods
- Understanding the modeling process and the role of agent-based methods.
- Types of models: deterministic, probabilistic, agent-based.
2. Differential Equations, Systems, and Agent-Based Simulations
- Ordinary differential equations (ODEs), partial differential equations (PDEs), and their role in agent-based models.
- Implementing agent-based simulations using Python, NetLogo, or other platforms.
3. Numerical Methods, Simulations, and Agent-Based Modeling
- Finite difference methods, finite element methods for agent-based simulations.
- Hands-on experience with agent-based modeling software.
4 Optimization Techniques for Agent-Based Models
- Incorporating optimization into agent-based models.
- Applications in social sciences, economics, and ecology.
5. Statistical Models, Data Analysis, and Agent-Based Approaches
- Probability distributions, statistical inference for agent-based models.
- Analyzing agent-based simulation results and validating against data.
6. Agent-Based Models in Complex Systems
- Exploring emergent behaviors and self-organization in complex systems.
- Applications in urban planning, traffic flow, and network dynamics.
7. Case Studies and Applications of Agent-Based Modeling
- Modeling epidemics, social dynamics, market behavior.
- Simulating ecological interactions, ecosystem dynamics.
8. Sensitivity Analysis, Validation, and Interpretation
- Assessing agent-based model sensitivity and robustness.
- Validating agent-based model outcomes against real-world data.
9. Project-Based Learning with Agent-Based Modeling
- Collaborative projects involving real-world scenarios and agent-based simulations.
- Developing, implementing, and analyzing agent-based models.
10. Presentation and Communication of Agent-Based Results
- Effectively communicating complex mathematical concepts and agent-based findings.
- Presenting agent-based simulation results to technical and non-technical audiences.
-
Assessment
Assessment will be based on a combination of individual and group projects, quizzes, assignments, presentations, and a final examination. Emphasis will be placed on practical application, agent-based modeling, and the ability to integrate theoretical knowledge into real-world contexts. -
Note
Will be given to the students in class.
-
Details
- Course title: Numerical Analysis
- Number of ECTS: 6
- Course code: F1_MA_MAT_MMCS2-5
- Module(s): Financial Mathematics 1
- Language: EN
- Mandatory: No
-
Objectives
-
Course learning outcomes
At the end of the course, a student will be able
- to understand the main methods, the rigorous mathematical analysis and algorithms developed in numerical analysis
- to master their concrete implementation on a computer
- to formulate some basic physics or engineering applications in view of their treatment by a numerical method and its computer solution.
-
Description
The main results about the theory of numerical methods will be explained. During the course, the student will implement algorithms in Python to propose some concrete, robust and efficient scientific computing solutions to concrete problems. The course content is the following:
- Notion of error in numerical analysis
- Polynomial interpolation and approximation
- Numerical derivation and integration
- Numerical solution of Ordinary Differential Equations
- Direct and iterative methods for solving linear systems
- Nonlinear equations in one and several variables
- Project presentation + Complementary topics (optimization, eigenvalues problems)
-
Assessment
The evaluation will be based on continuous assessment (weekly programming exercises – 50 % of the grade) and a project presentation with a report (50 % of the grade).
-
Note
- X. Antoine, Numerical Analysis, course at the University of Luxembourg
- Ascher, Uri M and Greif, Chen, A First Course in Numerical Methods, SIAM, 2011.
- Heath, Michael T, Scientific computing: an introductory survey, revised second edition SIAM, 2018.
-
Details
- Course title: Student project 1
- Number of ECTS: 4
- Course code: F1_MA_MAT_GM-7
- Module(s): Financial Mathematics 1
- Language: EN
- Mandatory: No
-
Course learning outcomes
On successful completion of the project the student should be able to:
- analyse complex tasks
- propose solution strategies,
- break up a longer project into subsequent steps,
- apply a variety of methods in one project,
- present a task and its solution in a scientific way.
-
Description
The student project consists of project work that is carried out under the supervision of a professor or a postdoc. The work is either individual or group work. Group work needs the explicit approval of the Study Director.
At the beginning of the project, supervisor and student(s) define tasks to be carried out by the student(s), corresponding to the volume of 100 working hours (4 ECTS). The student(s) need to notify the Study Director of the project and the tasks at the latest on 15 October.
The project outcome is a pdf document written by the student. Additional outcomes (such as computer code, images, videos) can be asked for.
The required outcome has to be handed in on Moodle at the latest on 31 December.
-
Assessment
The students are marked for their project work.
In the case of a retake exam, a new project has to be done. The retake can be done with other supervisors.
-
Note
Note / Literature / Bibliography
Depends on the project.
-
Details
- Course title: Student seminar 1
- Number of ECTS: 1
- Course code: F1_MA_MAT_GM-8
- Module(s): Financial Mathematics 1
- Language: EN
- Mandatory: No
-
Course learning outcomes
On successful completion of the Student Seminar 1, the students should be able to:
- Acquire good insight into a field by means of individual work
- Deliver a mathematical lecture on a topic of their choice
- Share their knowledge with others
-
Description
Every participant chooses a supervisor among the academic staff of the Department of Mathematics or among the instructors of the Master in Mathematics and, jointly with the supervisor, a topic for a talk.
The audience of the talk consists at least of the participants of the Student Seminar 1, the supervisor and one other academic staff member of the Department of Mathematics (e.g. the supervisor of another talk). The duration of the talk is 40 minutes (time for questions included). -
Assessment
The evaluation is based on the quality of the talk delivered by the student -
Note
Note / Literature / Bibliography
Depends on the subject and is communicated by the chosen supervisor
Course offer for Financial Mathematics, Semestre 2 (2024-2025 Summer)
-
Details
- Course title: Advanced Stochastic Models and Financial Applications
- Number of ECTS: 5
- Course code: F1_MA_MAT_FM-4
- Module(s): Financial Mathematics 2
- Language: EN
- Mandatory: Yes
-
Objectives
- Learning the concept of stable convergence
- Reviewing the theory of semimartingales
- Proving the law of large numbers for power variation of semimartingales
- Showing the stable central limit theorem for power variation of semimartingales
- Discuss statistical applications
-
Description
This course gives an introduction to statistics of high frequency data. The main focus is on power variation statistics of semimartingales, and in particular on the law of large numbers and a stable limit theorem. -
Assessment
Written exam at the end of the course -
Note
Literatur- Jean Jacod and Philip Protter “Discretization of Processes”, Springer 2012.
-
Details
- Course title: Algebraic Topology
- Number of ECTS: 8
- Course code: F1_MA_MAT_GM-12
- Module(s): Financial Mathematics 2
- Language: EN
- Mandatory: No
-
Course learning outcomes
On successful completion of the course, the student should be able to:
- Explain the main definitions and results of Algebraic Topology;
- Comment on new concepts;
- Apply the new techniques and solve problems;
- Structure the acquired abilities and summarize essential aspects adopting a higher standpoint;
- Give a talk for peers or students on a related topic and write scientific texts or lecture notes, observing modern standards in scientific writing, in Didactics and in Pedagogy;
- Provide evidence for the mastery of the Mathematical Method.
-
Description
Topology is a field of mathematics that deals with the fundamental properties of spaces. In the topological world, two spaces are considered identical if they can be continuously transformed into one another or more generally, if they are related by what topologists call homotopy equivalence. For example, a sphere and a pyramid are identical, as are a donut and a coffee cup… A main goal is therefore to classify spaces up to homotopy equivalence.
Algebraic topology uses algebraic methods to solve this and other topological problems. An important method is the search for algebraic homotopy invariants. In particular, we try to assign an algebraic object such as a group to each topological space and to prove that this group is invariant if we replace the space with a homotopy equivalent space. One of the proofs of the fundamental theorem of algebra uses an algebraic invariant.
The main homotopy invariants we discuss are the ‘singular homology functor’ and the ‘homotopy functor’. An amazing result called the Hurewicz isomorphism, shows that the first singular homology group and the (abelianized) first homotopy group of a space are two variants of the same information. Van Kampen’s theorem allows us to practice our understanding of category theory and to compute homotopy groups of larger spaces from those of smaller spaces from which they are constructed. The final chapter will highlight the relationship between the coverings of a space and subgroups of its first homotopy group.
The objective is to allow the student to familiarize himself with a very active field of mathematics, with broad applications throughout science. Beyond this goal, special emphasizes will be put on the Mathematical Method, i.e., the optimal technique to learn and apply mathematics. This method is the most important of the objectives of any study program in mathematics.
Fundamental concepts of homological algebra
Chain complexes, chain maps, chain homotopies, homology functor, connecting homomorphism, Künneth isomorphism, simplicial and singular homology, homotopy invariance, examples and applications.Homotopy groups
Algebra and topology (revision), first homotopy group, Hurewicz isomorphism, homotopy functor, homotopy invariance, typical examples and applications.Van Kampen theorem
Covering space theory
Free product with amalgamation, Van Kampen-Seifert theorem (basic, general and groupoid version), examples and applications (in particular: first homotopy group of a product and of a coproduct).
Coverings of spaces, lifting property, coverings of groupoids, orbit categories, classifications and constructions of coverings, connections with the preceding chapters, examples and applications. -
Assessment
Written exam -
Note
Literatur- Allen Hatcher, Algebraic Topology, Cambridge University Press, 2002 – Mathematics – 544 pages, ISBN-13: 978-0521795401
- Peter May, A concise course in Algebraic Topology, University of Chicago Press, 1999 – Mathematics – 243 pages, ISBN-13: 978-0226511832
- Charles Weibel, An introduction to homological algebra, Cambridge studies in advanced mathematics 38, 1997, ISBN: 0-521-55987-1
- Saunders Mac Lane, Categories for the Working Mathematician, Graduate Texts in Mathematics, 5(2), Springer, 1978, ISBN: 978-0-387-98403-2
-
Details
- Course title: Numerical Linear Algebra
- Number of ECTS: 5
- Course code: F1_MA_MAT_MMCS2-6
- Module(s): Financial Mathematics 2
- Language: EN
- Mandatory: No
-
Description
Topics : matrices, linear systems, eigenvalues/eigenvectors.
The course is devided into three parts.- Part one is devoted to “reminders” about matrix theory: matrix norms, triangularization, diagonalization, spectral decomposition, perturbation theory, complexity of some matrix operations
- Part two is concerned with the numerical analysis of several algorithms
- Solving linear systems
- Gaussian elimination, LU and Cholesky methods,…
- The conjugate gradient method
- The least squares problem
- Normal equations, QR and Householder algorithms,…
- The eignevalues problem
- Power methods, Jacobi, Givens–Householde, Lanczos methods,…
- Solving linear systems
- Part three is devide to computer sessions to program some of the previous numerical methods. Programming language : Python.
-
Assessment
- written final exam (50%)
- project (50%) carried out by groups of 2 to more students
-
Note
NoteCourse
Part 1 (draft version)
Exercises
Exercices 1 (Preliminaries (linear algebra))
Exercices 2 (Norms, condition number)
Computer Sessions
Exercices 1 Gram-Scmidt process
-
Details
- Course title: Continuous Time Models in Mathematical Finance
- Number of ECTS: 8
- Course code: F1_MA_MAT_FM-5
- Module(s): Financial Mathematics 2
- Language: EN
- Mandatory: Yes
-
Objectives
Introduction to continuous time models in mathematical finance (Black-Scholes model).
-
Description
Arbitrage, risk-neutral measures, option pricing, hedging, Black-Scholes-Merton equation, call-put parity, connections with partial differential equations, forwards and futures, american options, exotic options, change of numéraire, Garman-Kohlhagen formula, term-structure models, Vasicek model, Heath-Jarrow-Morton model, forward LIBOR model. -
Assessment
Written exam
-
Note
Literatur- M. Baxter, A. Rennie: Financial calculus. An introduction to derivative pricing. CUP, 1996
- F. E. Benth: Option Theory with Stochastic Analysis. Springer, 2004
- R. J. Elliot, P. E. Kopp: Mathematics of Financial Markets. 2nd ed., Springer Finance, 2004
- J. C. Hull: Options, futures, and other derivatives. 6th ed., Prentice-Hall, 2005
- S. E. Shreve: Stochastic calculus for finance. II: Continuous-time models. Springer, 2004
- J. Michael Steele: Stochastic Calculus and Financial Mathematics. Springer, 2001
-
Details
- Course title: Mathematical Statistics II
- Number of ECTS: 5
- Course code: MA_DS-35
- Module(s): Financial Mathematics 2
- Language: EN
- Mandatory: No
-
Course learning outcomes
In parametric models, a successful student should be is able to establish the main properties (consistency, asymptotic normality, etc) of the most classical estimators, provide (possibly asymptotic) confidence regions and tests between two hypotheses. -
Description
The main problems of mathematical statistics: estimation, testing, the notion of risk. Construction of confidence intervals and tests. False discovery rate. The empirical measure and its applications: the moment method, estimation by empirical quantiles, the Kolmokorov-Smirnov Test. The maximum likelihood estimator. Exponential families in one dimension. Introduction to the Bayes paradigm and elements of decision theory. -
Assessment
First session
A partial written exam and a final written exam.
Retake exam
Oral exam -
Note
Literatur
For probability theory: Real Analysis and Probability, R.M. Dudley- For statistics: Mathematical Statistics, P. Bickel and K. Doksum
-
Details
- Course title: Numerical solution of partial differential equations and applications
- Number of ECTS: 6
- Course code: F1_MA_MAT_MMCS2-7
- Module(s): Financial Mathematics 2
- Language: EN
- Mandatory: No
-
Objectives
This course is an introduction to the numerical solution of partial differential equations (PDEs). It contains a theoretical part setting the mathematical foundations necessary for some important numerical methods used to obtain solutions to some classical PDEs, in particular the finite element method and the finite difference method.
The theoretical part of the course is supported by the development of a one-dimensional Galerkin finite element code for the Poisson problem and a one-dimensional finite difference code for a scalar hyperbolic transport problem.
We largely follow the reference [Qua09] which is available via the National Library’s Website as well as on some less official websites. In particular, we cover the contents of [Qua09, Chapters 1, 2, 3, 4, and 14]. -
Description
A brief survey of partial differential equations
This chapter briefly introduces the notion of linear PDEs and their classification into elliptic, parabolic and hyperbolic equations. We mention some classical examples, mainly issued from physics and engineering, such as the transport equation, the Laplace equation, the heat equation and the wave equation.Elements of functional analysis
We introduce the main notions and theoretical results of functional analysis that are extensively used in the numerical analysis of partial differential equations. We consider the Riesz representation theorem regarding representation of continuous linear forms on a Hilbert space, and we survey the notions of bilinear form, of continuous injection of a Hilbert space into another, the notion of derivative in the sense of Fréchet, some elements of the theory of distributions, the basic properties of the Lebesgue and Sobolev spaces, and the notion of adjoint operator.Elliptic equations
We illustrate boundary-value problems for elliptic equations (in one and several dimensions), present their variational reformulations, treat the boundary conditions and analyze their well-posedness. Several examples of physical interest are introduced, in particular the Poisson equation, starting with the one-dimensional case, for various boundary conditions. We consider some variational formulations of these problems, and then turn to the boundary-value problems associated to the Poisson equation in the two-dimensional case. We establish that under some regularity condition the weak formulation is equivalent to the strong one. For general elliptic problems, the Lax-Milgram theorem ensures that the weak formulation is well-posed.The Galerkin finite element method for elliptic problems
We formulate Galerkin’s method for the numerical discretization of elliptic boundaryvalue problems and analyze its existence, uniqueness, stability and convergence features in an abstract functional setting. We then introduce the Galerkin finite elements method, first in one dimension, and then in several dimensions.The Galerkin finite element method – a numerical example
This section introduces students to the development of a one-dimensional Galerkin finite element solver for the Poisson problem using Python. We focus on building the solver within a provided Jupyter notebook, providing hands-on experience with the computational and algorithmic aspects of the finite element method.Finite differences for hyperbolic equations
Finite differences – a numerical example
The aim of this final chapter is to study classical finite differences methods for approximating solutions of first-order hyperbolic equations. We start with exposing some hyperbolic equations starting with the scalar transport problem in one dimension which we analyze by the method of characteristics. We also establish an a priori estimate by the energy method. We then turn to systems of linear hyperbolic equations in one dimension and give the example of the wave equation. Then we introduce the finite difference method, together with its variants: the forward/centered Euler scheme, the Lax-Friedrichs scheme, the Lax-Wendroff scheme, in the case of the scalar transport problem, before to move to more general cases, for which we analyze the consistency, stability, convergence, dissipation and dispersion properties of the finite difference methods.
This section introduces students to the development of a one-dimensional finite difference solver for a hyperbolic transport problem using Python. We focus on building the solver within a provided Jupyter notebook, providing hands-on experience with the computational and algorithmic aspects of the finite difference method. -
Assessment
First session
Assessment is via coursework (30%) and final examination (70%). The due date for the coursework will be set at a later time.
Retake policy
Failure on the coursework can be compensated through the examination, and vice versa, as long as the final mark for the course is greater than or equal to 10.
In the event that the final mark is less than 10:- The student may request a retake the examination once.
- The student may not resubmit the coursework.
-
Note
Reference
[Qua09] Alfio Quarteroni. Numerical models for differential problems. Vol. 2. Springer, 2009.
-
Details
- Course title: Sustainable Scientific Software
- Number of ECTS: 3
- Course code: F1_MA_MAT_MMCS2-8
- Module(s): Financial Mathematics 2
- Language: EN
- Mandatory: No
-
Objectives
During this course you will learn foundational skills that will enable you to
develop numerical and scientific software that is sustainable and reproducible.
-
Course learning outcomes
Students should be able to:- Understand the motivation for writing scientific software to a high standard.
- Work effectively as a team to write consistent and clean code, and share that code using tools, e.g. version control.
- Understand appropriate methodologies for improving code quality, robustness, and performance, e.g. linting, writing unit tests, static analysis tools, debuggers, performance profilers.
- Effectively plan a small piece of software, including the choice of and combination of different programming paradigms.
- Reflect on the tradeoffs in terms of time to write vs overall quality. and the long term sustainability implications.
-
Description
- Definitions of sustainability in a software context
- Introduction to the Unix shell with bash
- Introduction to version control with git
- Clean code, style guidelines and working in a team
- Tools for improving code.
- Writing documentation
- Test driven development
- Containers
- Continuous integration
-
Assessment
Two courseworks are set during the semester with equal weight towards the final mark.
-
Note
Note / Literature
https://jhale.github.io/sustainable-scientific-software/ for further links.
-
Details
- Course title: Partial Differential Equations II
- Number of ECTS: 8
- Course code: F1_MA_MAT_GM-15
- Module(s): Financial Mathematics 2
- Language: EN
- Mandatory: No
-
Objectives
Learning tools in order to deal with PDE, understanding the interplay between local and global problems and techniques.
-
Description
Distributions as generalized functions continued, Sobolev spaces, elliptic regularity, elliptic operators on compact manifolds, some non-linear equations. -
Assessment
Written exam -
Note
Literatur- Jost: Postmodern analysis
- Folland: Introduction to partial differential equations
- Reed-Simon: Methods of mathematical physics I-IV
- Aubin: Nonlinear analysis on manifolds
-
Details
- Course title: Advanced Graph Theory
- Number of ECTS: 6
- Course code: F1_MA_MAT_GM-16
- Module(s): Financial Mathematics 2
- Language: EN
- Mandatory: No
-
Objectives
-
Course learning outcomes
On successful completion of the course, the student should be able to
- Illustrate the main results and concepts with well-chosen examples
- Master the proofs and techniques of the theory
- Solve exercises related to the topics covered in class
- Give an overview of the course content, focusing on his/her own taste and favorite topics
- Communicate his/her own pleasure in solving mathematical problem
-
Description
Graphs are structures that are ubiquitous in Mathematics and its applications. Through a presentation of selected topics, the course aims to be an introduction to certain modern aspects of graph theory.
Some basic knowledge of the concept of graphs is a prerequisite for this course.
According to time and taste, topics covered will be chosen among (and are not limited to) the following ones:- Matching, covering and packing problems
- Edge and vertex colorings
- Graph polynomials
- Ramsey theory for graphs
- Infinite Graph Theory
-
Assessment
Oral exam or written exam according to number of participants
-
Note
Literatur- Reinhard Diestel. Graph Theory, 5th edition. Graduate texts in mathematics 173, Springer, 2017
-
Details
- Course title: High Dimensional Statistics
- Number of ECTS: 5
- Course code: MA_DS-11
- Module(s): Financial Mathematics 2
- Language: EN
- Mandatory: No
-
Objectives
The goal of the course is a detailed introduction to modern techniques in high dimensional estimation of linear models and covariance matrices. After successful completion the student should be able to establish the main properties of Lasso and related estimators in the setting of linear regression models, asymptotic results for large random matrices and non-asymptotic properties for high dimensional estimators of covariance matrices.
-
Description
- High dimensional regression model
- Parameter estimation under constraints: Lasso and relates estimation procedures
- Variable selection
- Introduction to principal component analysis
- Basic elements of random matrix theory: semi-circle law, Marcenko-Pastur distribution, Tracy-Widom distribution
- Estimation of high dimensional covariance and precision matrices; hard and soft thresholding
-
Assessment
There will be a written exam at the end of the course. -
Note
Bibliography- P. Bühlmann and S. van de Geer „Statistics for High-Dimensional Data“
- C. Giraud “Introduction to High-Dimensional Statistics”
- G.W. Anderson, A. Guoinnet and O. Zeitouni „An Introduction to Random Matrices“
-
Details
- Course title: Riemann Surfaces
- Number of ECTS: 8
- Course code: F1_MA_MAT_GM-13
- Module(s): Financial Mathematics 2
- Language: EN
- Mandatory: No
-
Course learning outcomes
At the end of the course the students should have a basic understanding of what Riemann surfaces are and how they can be studied from different points of view, especially the complex and topological one. They should be able to understand and solve simple problems involving the material from the course. -
Description
A primary goal of various kinds of geometric theories is to describe shapes of arbitrary dimensions from a mathematical point of view. One way to do so is to build such shapes out of pieces of a euclidean space R^n, obtaining smooth manifolds. In some cases, smooth manifolds can be covered instead with pieces of a complex affine space C^n: when the complex dimension is 1, such a manifold is a Riemann surface (its real dimension will be 2).
From a topological point of view, we will be looking simply at a sphere, a doughnut, or a surface with more “holes”; however, the mere existence of a complex structure provides the foundations for an extremely rich theory. Riemann surfaces lie at the crossroads of many fields of research of modern mathematics: topology, complex and algebraic geometry, number theory and harmonic analysis, among others.
The course will cover the basic notions of the theory, starting from the definition of a complex manifold, and move on to the classification of Riemann surfaces via the study of their divisors. This will allow the students to acquire familiarity with some ubiquitous tools from complex geometry, such as the Riemann-Roch theorem.
We will put some focus on examples along the way. If time allows, we will see how some constructions from the complex analytic theory can be reinterpreted in the language of modern algebraic geometry, bridging the gap between the two worlds, and we will touch some more advanced topics that could inspire the students’ further work.
-
Assessment
Weekly exercise will be provided and the students will be asked to present some of them orally. This will provide for continuous evaluation that will make up for 1/3 of the grade. The remaining 2/3 will be given by the result of a final oral exam. -
Note
Literatur- Rick Miranda, Algebraic Curves and Riemann Surfaces.
- Martin Schlichenmaier, An Introduction to Riemann Surfaces, Algebraic Curves and Moduli Spaces.
- Oleksandr Iena, Riemann surfaces. Lecture Notes. Winter Semester 2015/2016.
The above texts are available on a-z.lu. More references will be provided during the course.
-
Details
- Course title: Student Project 2
- Number of ECTS: 4
- Course code: F1_MA_MAT_GM-18
- Module(s): Financial Mathematics 2
- Language: EN
- Mandatory: No
-
Course learning outcomes
On successful completion of the Student Project 2, the student should be able to:
- analyse complex tasks,
- propose solution strategies,
- break up a longer project into subsequent steps,
- apply a variety of methods in one project,
- present a task and its solution in a scientific way.
-
Description
The student project consists of project work that is carried out under the supervision of a professor or a postdoc. The work is either individual or group work. Group work needs the explicit approval of the Study Director. At the beginning of the project, supervisor and student(s) define tasks to be carried out by the student(s), corresponding to the volume of 100 working hours (4 ECTS). The student(s) need to notify the Study Director of the project and the tasks at the latest on 15 March. The project outcome is a pdf document written by the student. Additional outcomes (such as computer code, images, videos) can be asked for. The required outcome has to be handed in on Moodle at the latest on 31 May. The project file will undergo an automatic plagiarism check. -
Assessment
The students are marked for their project work. In the case of a retake exam, a new project has to be done. The retake can be done with other supervisors.
-
Details
- Course title: Student seminar 2
- Number of ECTS: 2
- Course code: F1_MA_MAT_GM-19
- Module(s): Financial Mathematics 2
- Language: EN
- Mandatory: No
-
Course learning outcomes
On successful completion of the Student Seminar 2, the students should be able to:
- Fully benefit from seminar talks
- Acquire good insight into a field by means of individual work
- Give themselves lectures on specific topics
- Share their knowledge with others
-
Description
Every participant chooses a supervisor among the academic staff of the Department of Mathematics and, jointly with the supervisor, a topic for a talk.
The audience of the talk consists at least of the participants of the seminar, the supervisor and one other academic staff member of the Department of Mathematics (e.g. the supervisor of another talk). The duration of the talk is 75 minutes (time for questions not included).
A typewritten version of this (these) lecture(s) is requested.
The students’ seminar talks will take place during April and May. The exact timetable depends on the number of registered students and is made during the semester.
The supervisor together with the course responsible marks the students’ performance and the written text. -
Assessment
Continuous evaluation. The mark is based on the talk and the written text delivered by the student.
If a student fails, a new talk and a new text can be delivered in the following semester. A supervisor change is allowed.
Course offer for Financial Mathematics, Semestre 3 (2024-2025 Winter)
-
Details
- Course title: Lie Algebras and Lie Groups
- Number of ECTS: 6
- Course code: F1_MA_MAT_GM-20
- Module(s): Financial Mathematics 3
- Language: EN
- Mandatory: No
-
Objectives
The purpose of this course is to give an introduction into the theory of finite dimensional Lie groups and Lie algebras, assuming some basic knowledge of differentiable manifolds.
-
Course learning outcomes
On successful completion of the course, the student should be able to:
- Expound the mathematical foundation behind symmetries of solid bodies, dynamics of mechanical systems, and geometric structures in nature.
- Explain the deep interrelations between Lie groups and Lie algebras, as well as the technical tools behinds these interrelations.
- Simplify mathematical problems admitting symmetry Lie groups actions to problems admitting symmetry actions of their Lie algebras.
- Master applications to the theory of manifolds and representation theory, which in turn have applications in physics, engineering and mechanics.
-
Description
The Lie algebra of a Lie group, the exponential map, the adjoint representation, actions of Lie groups and Lie algebras on manifolds, the universal enveloping algebra, basics of the representation theory. -
Assessment
First session
End of course assessment -
Note
Note / Literature / Bibliography- “Lie groups and Lie algebras” by Eckhard Meinrenken, 83 pages (free to download)
- “Prerequisites from Differential Geometry” by Sergei Merkulov (free to download)
-
Details
- Course title: American Options: Optimal stopping theory and numerical methods
- Number of ECTS: 5
- Course code: F1_MA_MAT_FM-7
- Module(s): Financial Mathematics 3
- Language: EN
- Mandatory: Yes
-
Objectives
The lectures provide an introduction to American options and optimal stopping problems (theoretical foundation and numerical implementation).
-
Course learning outcomes
On successful completion of the course, the student should be able to:
- Explain pricing and hedging of ‘Vanilla’ American options in a multi-asset Black-Scholes model using bi- or multinomial trees
- Extend multinomial tree methods to the pricing and hedging of American options in local volatility or stochastic volatility models (CEV, SABR, Heston…)
- Implement efficiently a regression method ‘à la Longstaff-Schwarz’ for solving multi-dimensional optimal stopping problems like the pricing and the hedging of multi-asset American options or energy derivatives like swing options (take-or-pay gas con-tracts)
- Implement an optimal quantization based numerical scheme to price and hedge multi-asset American style derivatives
- Analyze these numerical methods and their respective ranges of efficiency, especial-ly compared to PDE methods
-
Description
- Discrete time optimal stopping theory: Snell envelope, Rogers’ dual representation, optimal stopping times
- Backward dynamical programming principle
- American options pricing and hedging in a complete market, with and without div-idends
- Bermuda options (discrete time)
- Swing options on energy markets
- Numerical methods: variational inequality (PDE method), regression methods (Longstaff-Schwarz), Quantization methods with a priori error bounds
- Continuous time optimal stopping theory and applications to complete markets
- Réduites, variational inequalities, free boundary
-
Assessment
First session
Written or oral exam (depending on the number of enrolled students)
Retake exam
Written or oral exam (depending on the number of enrolled students) -
Note
Note / Literature / Bibliography
Support
provided in class
Literature- L. Carassus, G. Pagès (2015). Finance de marché, Modèles mathématiques à temps discret, Vuibert, 400p.
- V. Bally, G. Pagès, J. Printems (2005). A quantization tree method for pricing and hedging multidimensional American options, Mathematical Finance, 15(1):119-168.
- É. Clément, P. Protter, D. Lamberton (2002). An analysis of a least squares regression method for American option pricing, Finance Stochastics, 6(2):449-47
- N. El Karoui (1981). Les aspects probabilistes du contrôle stochastique, Lecture Notes in Mathematics 876, 72-238, Springer Verlag.
- D. Lamberton, B. Lapeyre (1996). Introduction to stochastic calculus applied to fi-nance. Chapman Hall, London, 185 pp. ISBN: 0-412-71800-6.
- F.A. Longstaff, E.S. Schwarz (2001). Valuing American options by simulation: a simple least-squares approach, Review of Financial Studies, 14:113-148.
- J. Neveu (1964). Bases mathématiques du calcul des Probabilités, Masson, Paris; Eng-lish translation Mathematical Foundations of the Calculus of Probability (1965), Holden Day, San Francisco.
- A. N. Shyriaev (2008). Optimal stopping rules. Translated from the 1976 Russian sec-ond edition by A. B. Aries. Reprint of the 1978 translation. Stochastic Modeling and Applied Probability, 8, Springer-Verlag, Berlin, 217 pp.
-
Details
- Course title: Combinatorial Geometry
- Number of ECTS: 6
- Course code: F1_MA_MAT_GM-21
- Module(s): Financial Mathematics 3
- Language: EN
- Mandatory: No
-
Objectives
Beyond learning exciting material, the course is designed to explore and experience the process of mathematical research.
-
Course learning outcomes
The course requires minimal prerequisites (some linear algebra, Euclidean geometry and basic topology) but aims to explore results that are at the limit of current known understanding. In particular, we’ll discuss some open problems and try to illustrate the process of modern research. The subjects are chosen so that they can be treated with a hands-on approach, and this approach and experience are as important for this course as the actual content. -
Description
The course will take the form of a topics course, presenting a selection of themes from combinatorial aspects of geometry. Topics may include cube complexes, convex sets in R^n, combinatorial ways of exploring surfaces, topics in graph theory…
-
Assessment
First session
3 short in-class tests, worth together a total of 20%; final written exam worth 80%.
Retake exam100% final written exam, no kept grade.
Absence plan: the final grade will be worked out as follows:- Case 1: Student attends all three short tests. The best two out of the three scores will count for 10% each, and the final exam will count for 80%.
- Case 2: Student attends two short tests. The two scores will count for 10% each and the final exam will count for 80%.
- Case 3: Student attends one short test. The test score will count for 10% and the final exam will count for 90%.
- Case 4: Student attends no short test. The final exam will count for 100%.
-
Note
Note / Literature / Bibliography
Varied. (A mix of lecture notes and articles.)
-
Details
- Course title: Numerical methods in Finance
- Number of ECTS: 5
- Course code: F1_MA_MAT_FM-8
- Module(s): Financial Mathematics 3
- Language: EN
- Mandatory: Yes
-
Objectives
The objective of this course is to present numerical methods currently used in finance, especially in option pricing and portfolio optimization, and to introduce basic skill programming to tackle problems encountered in mathematical modelling of finance.
-
Course learning outcomes
On successful completion of the course, the student should be able to:
- Explain and apply relevant numerical methods currently used in finance.
- Carry out calculations based on Monte Carlo methods, especially in option pricing and portfolio optimization.
-
Description
First, we will introduce mathematical problems occurring in finance, mainly in option pricing and portfolio optimization in financial markets following stochastic models. These formulations call upon partial differential equations, conditional expectation, optimal time, dynamic programming and control. Next, we shall describe methods to approach numerical simulations of these objects. Finally, in practical sessions, we will use recent software and Python programming to illustrate efficiency of these methods.
The course will be organized in three parts:
- PDE methods for option pricing and numerical methods in stochastic control: 15h (A. Sulem)
- Monte Carlo methods (tree method, regression, machine learning) : 8h (Ludovic Goudenège)
- Applied sessions with computer using the computational finance software “Premia” (www.premia.fr) and Python programming: 7h (Ludovic Goudenège)
-
Assessment
First session
Written exam with possibly an applied project
Retake exam
Written exam with possibly an applied project -
Note
Note / LiteratureD. Lamberton and B. Lapeyre. Introduction to Stochastic Calculus Applied to Finance. Chapman and Hall, 2nd edition, 2007.
- B. Lapeyre, A. Sulem and D.Talay. Simulation of Financial Models: Mathematical Foundations and Applications, to appear. Cambridge University Press.
- B. Jourdain. Probabilités et statistique. Ellipes, 2009.
- T. M. Mitchell. Machine learning. WCB/McGraw-Hill, 1997.
- G. Pagès. Introduction to numerical methods in probability in finance. 3em cycle Hanoi. Vietnam, 2007.
-
Details
- Course title: Gaussian processes and applications
- Number of ECTS: 5
- Course code: F1_MA_MAT_FM-9
- Module(s): Financial Mathematics 3
- Language: EN
- Mandatory: No
-
Objectives
The objective is to learn the basic concepts and techniques associated with Gaussian processes, that are omnipresent in modelling random phenomena (finance, physics, machine learning, statistics, etc.)
-
Course learning outcomes
On successful completion of the course, the student should be able to:
- Explain the language, basic concepts and techniques associated with Gaussian variables, vectors, and processes
- Identify, analyse, and prove relevant properties of models based on a Gaussian structure
- Solve exercises involving a Gaussian structure
-
Description
- Gaussian random variables (characteristic function, CLT, stability properties, Stein’s lemma)
- Gaussian random vectors (definition, characteristic function, existence, uniqueness in law, multivariate CLT, density, Hermite polynomials)
- Gaussian random processes (definition, modifications, uniqueness in law, function of positive type, existence, Brownian motion, continuity)
- Fractional Brownian motion (definition, existence, Hölder regularity)
-
Assessment
Exam modalities for the first session
Written exam during the exam period (January/February).
Exam modalities for the retake exam
Written exam during the exam period July). -
Note
Note / Literature / Bibliography
Will be discussed in class
-
Details
- Course title: Advanced Econometrics
- Number of ECTS: 6
- Course code: QTECOFIN-2
- Module(s): Financial Mathematics 3
- Language: EN
- Mandatory: No
-
Objectives
This course is intended for students enrolled in the Master of Science program in Quantitative Economics and Finance.
Ph.D. students interested in learning this material are also welcome. The objective of this course is to familiarize students with microeconometric models and methods that are widely used in economics, finance, and social science research. We will focus on linear models. Nonlinear models are covered in a subsequent course.
On completion of the course unit successful students will be able to:
Have a good understanding of some widely used econometric models and techniques used by economists to answer policy related questions
Understand how these models are identified, estimated, and tested, how the asymptotic distributions of the various estimators and test statistics are obtained, and the fundamental assumptions underlying these results. This will not only enable the students to process and interpret empirical data and test whether they are in accordance with economic theory, but should also help them read, understand, and critically evaluate the econometrics articles in peer-reviewed journals encountered during the course of their own research.
-
Description
COURSE CONTENT
1. Basics.
_ What is a statistical model?
_ Interpreting and estimating models of causal relationships with observational data.
_ Exogenous and endogenous variables.
_ Reference: Chapter 1 and class-notes.
2. Statistics review.
_ Best linear predictors and best predictors.
_ Conditional expectations and their properties.
_ Basic asymptotic theory.
_ Applications of asymptotic theory to estimation and testing.
_ Reference: Chapters 2, 3 and class-notes.
3. Single equation linear models.
_ Ordinary least squares.
_ Consistency and asymptotic normality of OLS estimators.
_ Estimating heteroscedasticity-robust standard errors.
_ Problems associated with endogenous regressors.
_ Prevalence of endogenous regressors in econometric models.
_ The instrumental variables (IV) solution to endogenous regressors.
_ Reference: Chapter 4 and class-notes.
4. IV estimation of single equation models.
_ The two-stage least squares (2SLS) estimator and its properties.
_ The control function estimator and its properties.
_ Testing for endogeneity.
_ Relationship between 2SLS and control function estimators.
_ Reference: Chapters 5, 6 and class-notes.
5. Generated regressors and instruments.
_ OLS with generated regressors.
_ 2SLS with generated instruments.
_ Effect of generated regressors and instruments on the properties of estimators.
_ Specification testing in linear models with generated regressors.
_ Reference: Chapter 6 and class-notes.
6. Generalized method of moments (GMM)
_ Econometric models defined in terms of moment conditions.
_ Under-identified, just-identified and over-identified moment-condition models.
_ Estimation of just- and over-identified moment-condition models.
_ Asymptotic properties of GMM estimators.
_ Two-step optimal GMM estimators.
_ GMM based hypothesis testing.
_ GMM based specification testing.
_ Reference: Chapter 14 and class-notes.
7. Systems of equations with exogenous regressors.
_ Seemingly unrelated regressions (SUR).
_ Optimal GMM estimation of SUR models.
_ Imposing cross-equation restrictions.
_ Reference: Chapter 7 and class-notes.
8. Systems of equations with endogenous regressors.
_ GMM estimation of SUR with varying instruments.
_ GMM versus 3SLS.
_ Reference: Chapter 8 and class-notes.
9. Linear simultaneous equations.
_ Classical supply and demand models.
_ Natural instruments: identification via exclusion restrictions.
_ Identification via cross-equation and covariance restrictions.
_ Reference: Chapter 9 and class-notes.
10. Linear panel data models.
_ Linear panel data models without unobserved effects.
_ Linear panel data models with unobserved effects.
_ Estimating fixed effects models under strict exogeneity.
_ The random effects estimator.
_ Testing the random effects hypothesis.
_ Correlated random effects: Hausman-Taylor type models.
_ Dynamic panel data models and sequential exogeneity._ Reference: Chapters 10, 11 and class-notes.
-
Assessment
- 60% of the course grade is based on the final exam, which is closed book and closed notes. The final exam is comprehensive, i.e., it includes all material covered in the class, the homeworks, and the TA sessions
- 40% of the course grade is based on 5 homework assignments. The completed homeworks have to be handwritten and handed in individually
-
Note
Bibliography:
The required text for this course is
1. Econometric analysis of cross section and panel data, 2nd ed., J. M. Wooldridge (2010).
All chapter references in the syllabus are to this book.Students interested in additional reading may consider the following references:
“Econometrics,” B. Hansen, 2022.
“Econometric theory and methods,” J. G. MacKinnon and R. Davidson, 2009.
“Mostly harmless econometrics: An empiricist’s companion,” J. D. Angrist and J. S. Pischke, 2008.
“Microeconometrics: Methods and applications,” A. C. Cameron and P. K. Trivedi, 2005. Also by the same authors, “Microeconometrics using STATA,” 2009.
“Panel data econometrics,” M. Arellano, 2003.
“Econometric analysis,” W. H. Greene, 2003.
“Econometrics,” F. Hayashi, 2000.
“An introduction to classical econometric theory,” P. Ruud, 2000.
“Statistics and econometric models,” vol. 1 and 2, C. Gourieroux and A. Monfort, 1995.
“A course in econometrics,” A. S. Goldberger, 1991.
“Large sample estimation and hypothesis testing,” W. K. Newey and D. McFadden, in Handbook of Econometrics, vol. 4, 1994.
“Advanced econometrics,” T. Amemiya, 1985.
“Handbooks of econometrics,” vol. 1 – vol. 6B, 1983 – 2007.
-
Details
- Course title: Machine Learning – Reading course
- Number of ECTS: 5
- Course code: F1_MA_MAT_MMCS2-12
- Module(s): Financial Mathematics 3
- Language: EN
- Mandatory: No
-
Details
- Course title: Data Science
- Number of ECTS: 5
- Course code: F1_MA_MAT_GM-24
- Module(s): Financial Mathematics 3
- Language: EN
- Mandatory: No
-
Objectives
The successful candidate will understand the basic theoretical concepts of data-centric aspects and will be able to work on data-centric problems. The aim is to continue the work done in the project within the framework of a Master’s thesis.
-
Course learning outcomes
On successful completion of the course the student should be able to:
- Explain and apply basic theoretical concepts on selected aspects of data processing.
- Develop appropriate solutions for data-centered problems.
- Consolidation of the acquired competences in the subject area through a Master’s thesis.
-
Description
In this course, the term ‘data’ is seen centric and we will look at data from different perspectives. We will discuss selected aspects of Data Preparation and Preprocessing, Data Statistics, Data Security, Data Privacy, Data Management, Big and Small Data, Data Retrieval, Data Visualization, and Data Analytics. -
Assessment
First session
50% written assignment or oral interview + 50% practical.
Retake exam
Those who do not pass the course have the opportunity to retake the exam in the summer term. -
Note
Note / Literature / Bibliography- Elmasri, Navathe: Fundamentals of Database Systems. Pearson Addison Wesley. 2006.
- Han, Kamber: Data Mining – Concepts and Techniques. Morgan Kaufmann. 2011.
- Manning, Raghavan, Schütze: Introduction to Information Retrieval. Cambridge University Press.
Ware: Information Visualization. Morgan Kaufmann. 2012. - Witten, Kamber: Data Mining: Practical Machine Learning Tools and Techniques. Morgan Kaufmann.
- Aggarwal, Yu: Privacy-Preserving Data Mining – Models and Algorithms. Springer. 2008.
- Marz: Big Data: Principles and best practices of scalable realtime data systems. Manning. 2015.
as well as different articles, reports, and journals contributions.
-
Details
- Course title: Student Project 3
- Number of ECTS: 4
- Course code: F1_MA_MAT_GM-25
- Module(s): Financial Mathematics 3
- Language: EN
- Mandatory: No
-
Course learning outcomes
On successful completion of the Student Project 1 (or 3) the student should be able to:
- analyse complex tasks,
- propose solution strategies,
- break up a longer project into subsequent steps,
- apply a variety of methods in one project,
- present a task and its solution in a scientific way.
-
Description
The ability to work on a project, either individually or as part of a team, is one of the key skills expected of a mathematician, in and outside academia. The Student Project 1 offers the student the opportunity to improve this skill by allowing them to work on a well-defined and well chosen project, either individually or as a team, under the guidance of a researcher.
The Student Project 3 consists of project work that is carried out under the supervision of a researcher.The work is either individual or group work.
At the beginning of the project, supervisor and student(s) define tasks to be carried out by the student(s), corresponding to the volume of 100 working hours (4 ECTS) for each student. The student(s) need to submit the planned topic to the course responsible for approval at the latest on 15 October.
The project outcome is a pdf document written by the student(s).
Additional outcomes (such as computer code, images, videos) can be asked for. The required outcome has to be handed in on Moodle at the latest on 31 December. The project file will undergo an automatic plagiarism and AI check.Timeline for the student
Beginning of semester
- Connect to the Moodle classroom
- Find a supervisor and a topic
- On or before 15 October, get approval from the course responsible of the project participants, the supervisor and the topic
During the semester
- Work on the project
- Seek feedback from your supervisor
End of the semester
- Hand in your project on or before 31 December on Moodle (for plagiarism and AI check)
-
Assessment
First session
The students are marked for their project work. In the case of a retake exam, a new project on the same or a different topic has to be done.
Retake exam
The retake can be done with a different supervisor.
-
Details
- Course title: Internship (in a company)
- Number of ECTS: 5
- Course code: F1_MA_MAT_MMCS2-13
- Module(s): Financial Mathematics 3
- Language: EN
- Mandatory: No
-
Details
- Course title: Nonparametric Statistics
- Number of ECTS: 5
- Course code: MA_DS-18
- Module(s): Financial Mathematics 3
- Language: EN
- Mandatory: No
-
Objectives
Upon completion a successful student should be able to
- Construct classical estimators of unknown probability distribution
- Derive asymptotic properties of empirical distribution functions
- Perform tests for unknown distribution functions
- Construct non-parametric estimators of the density
- Estimate components of a non-linear regression model
- Understand the concept of minimax estimation
-
Course learning outcomes
- Understanding the concepts of empirical distribution functions, kernel estimation and minimax theory
- Performing derivation of standard estimation and testing methods in nonparametric statistics
-
Description
- Estimation of probability measures
- Weak limit theorems for empirical measures
- Kolmogorov-Smirnov and Cramer-von Mises tests
- Estimation of the density
- Nadaraja-Watson estimator and general kernel estimators
- Non-linear regression models
- Minimax theory
-
Assessment
First session
Solving bi-weekly exercises (40%) and a final exam (60%) will build the overall grade.
Retake exam
Written exam.
-
Note
Literatur- A.B. Tsybakov (2009): “Introduction to Nonparametric Estimation”, Springer.
- A. Van der Vaart and J. Wellner (1996): “Weak Convergence and Empirical Processes”, Springer.
-
Details
- Course title: Numerical Methods for Variational Problems
- Number of ECTS: 5
- Course code: F1_MA_MAT_MMCS2-9
- Module(s): Financial Mathematics 3
- Language:
- Mandatory: No
-
Course learning outcomes
At the end of the course students should have a thorough understanding of the mathematical underpinnings of the polynomial (p) finite element method and its implementation in Python code. If there is sufficient time students will also be exposed to advanced topics such as mixed finite element methods and preconditioning finite element linear systems.
-
Description
Please see https://jhale.github.io/finite-elements/ for a full syllabus. -
Assessment
First session
50% written examination.
50% coursework.
Retake examRetake exam possible if coursework component passed, otherwise course must be retaken.
Absence
Absence at final exam treated via procedure in Règlement des études. -
Note
Note / Literature / Bibliography
https://jhale.github.io/finite-elements/
The Mathematical Theory of Finite Element Methods, Brenner S.C. and Scott, L. R., Springer 2008. https://doi.org/10.1007/978-0-387-75934-0 (available digitally via the uni.lu library)
-
Details
- Course title: Bayesian Statistics
- Number of ECTS: 5
- Course code: MA_DS-22
- Module(s): Financial Mathematics 3
- Language: EN
- Mandatory: No
-
Objectives
Provide students with the necessary knowledge of Bayesian statistics, both from a theoretical as well as practical viewpoint.
-
Course learning outcomes
Handle of the concepts from Bayesian statistics, Bayesian inference, Bayesian modelling, practical handle of Bayesian techniques on Python. -
Description
This course will provide a thorough introduction and overview on the most important concepts from Bayesian inference, starting with the Bayesian philosophy in contrast to frequentist statistics. We will discuss various choices of prior distributions, Bayesian inference, Bayesian modelling, model checking and comparison (in particular introducing the concept of Bayes factor), and advanced computation. The latter aspect will especially be dealt with in the practicals, where Markov Chain Monte Carlo methods shall be treated. -
Assessment
First session
Written exam with open questions, both theory and exercises, in particular there will be computational exercises
Retake exam
Written exam with open questions, both theory and exercises, in particular there will be computational exercises -
Note
Note / Literature / Bibliography- Box, GEP and Tiao, GC (1992) Bayesian Inference in Statistical Analysis. John Wiley and Sons
- Gelman, A, Carlin, JB, Stern, HS, Dunson, DB, Vehtari, A and Rubin, DB (2013) Bayesian Data Analysis 3rd edition. Chapman Hall/CRC.
- Brooks, S, Gelman, A, Jones, GL, Meng, X-L (2011) Handbook of Markov Chain Monte Carlo. Chapman Hall/CRC
-
Details
- Course title: Continuous-Time Stochastic Calculus and Interest Rate Models
- Number of ECTS: 5
- Course code: F1_MA_MAT_FM-6
- Module(s): Financial Mathematics 3
- Language: EN
- Mandatory: Yes
-
Course learning outcomes
On successful completion of the course, the student should be able to:
- Calculate probabilities and expectations related to the semi-martingale models presented in the lectures
- Carry out calculations based on change of numéraire and no-arbitrage pricing
- Compute the prices of interest rate derivatives
- Apply stochastic volatility models to deal with implied volatility surfaces
-
Description
Basic Notions of Fixed Income Markets; Semimartingale Modeling; Stochastic Differential Equations; No-Arbitrage Pricing; Change of Numéraire; Short Rate Models; Heath-Jarrow-Morton Framework; Market Models; Stochastic Volatility.
-
Assessment
First session
Written exam
Retake exam
Written exam -
Note
Note / Literature / Bibliography- D. Brigo and F. Mercurio (2006) Interest Rate Models : Theory and Practice. Springer Verlag
- Damir Filipovic (2009). Term-structure models. A graduate course. Springer Verlag.
Course offer for Financial Mathematics, Semestre 4 (2024-2025 Summer)
-
Details
- Course title: Master Thesis
- Number of ECTS: 20
- Course code: F1_MA_MAT_FM-10
- Module(s): Master Thesis
- Language: FR
- Mandatory: No
-
Details
- Course title: Master Thesis with internship
- Number of ECTS: 30
- Course code: F1_MA_MAT_FM-11
- Module(s): Master Thesis with internship
- Language: FR
- Mandatory: No
-
Details
- Course title: Reading Course
- Number of ECTS: 5
- Course code: F1_MA_MAT_GM-30
- Module(s): Financial Mathematics 4
- Language: EN
- Mandatory: No
-
Description
This is an individual reading course about the background and complementary knowledge for a Master thesis. -
Assessment
The assessment is based on the performance in the meetings with the instructor(s).
-
Details
- Course title: Extended Reading Course
- Number of ECTS: 10
- Course code: F1_MA_MAT_GM-31
- Module(s): Financial Mathematics 4
- Language: EN
- Mandatory: No
-
Description
This is an individual extended reading course about the background and complementary knowledge for a Master thesis. -
Assessment
The assessment is based on the performance in the meetings with the instructor(s).
-
Details
- Course title: Complex Geometry
- Number of ECTS: 5
- Course code: F1_MA_MAT_GM-28
- Module(s): Financial Mathematics 4
- Language: EN
- Mandatory: No
-
Course learning outcomes
On successful completion of the course the student should be able to:
- Demonstrate proper understanding of the notion of complex manifold;
- Identify the most important examples;
- Master the main techniques of the theory;
- Prove good knowledge of the key theorems;
- Apply the new techniques to geometric problems;
- Give a pedagogic talk for peers on a related topic;
- Write clear and concise lecture notes, including appropriate exercises and applications
-
Description
The aim of the lecture course is to give an introduction to complex geometry. In particular the course will treat many examples. After the course the students should understand Hermitian vector bundles and the basics of Kahler manifolds and the Hodge decomposition. The students should be acquainted with the basic theorems and examples.
-
Assessment
First session
Oral examination (a presentation) and 1-2 homework sets -
Note
Literature- R.O. Wells: Differen9al Analysis on Complex Manifolds (main text);
- S.S. Chern: Complex Manifolds without Potential Theory;
- Griffiths Harris: Principles of Algebraic Geometry;
- F. Warner: Foundations of Differentiable Manifolds;
- D. Huybrechts: Complex Geometry;
- Lecture notes Martin SCHLICHENMAIER, available on the Moodle for download.
-
Details
- Course title: Optimisation for Computer Science
- Number of ECTS: 5
- Course code: MICS2-17
- Module(s): Financial Mathematics 4
- Language:
- Mandatory: No
-
Objectives
Different problems have different nature. In terms of complexity some problems are called intractable and can not be solved by classical computers. But there are also many other aspects of the nature of optimisation problems such as linearity, convexity, continuity, dynamicity, randomness that may lead the choice of different optimisation techniques
-
Course learning outcomes
* Characterize problems
* Identify the key concepts related to optimisation techniques
* Use optimization frameworks
* Implement optimization algorithms
* Validate optimization algorithms and results
* Validate approaches for solving optimization problems
-
Description
This lecture confront the students to real instances of such problems. They are first asked to model the problem and next proposed solutions include exact methods, relaxations, approximations, heuristics and meta-heuristics. And these practical study cases are supported by the theoretical lectures on Problem Solving (1st semester) -
Assessment
Project: 100%