Audacity
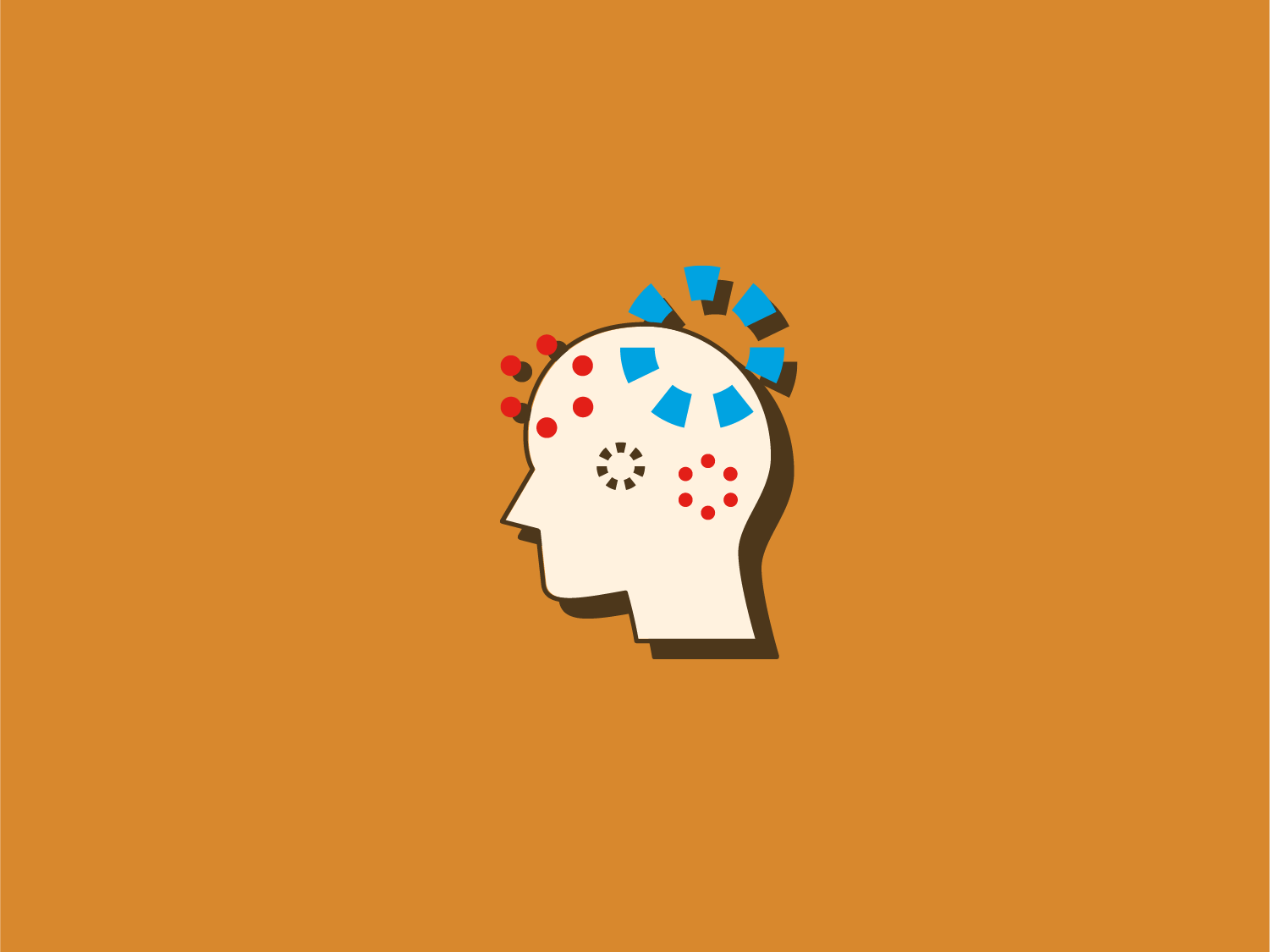
Audacious high-risk projects and new ideas.
2024
By Prof. Christophe LEY (FSTM) and Prof. Robert KUMSTA (FHSE)
Across the health sciences, a key goal is to prevent the onset of physical or mental health problems. The identification of pre-disease states, which could inform early intervention measures, rests on reliable prognostic biological and behavioural markers. Biobehavioral mechanisms and pathways eventually leading to dysfunctional trajectories are complex and multifactorial, so that single-point measures of isolated systems are bound to have low prognostic validity. Thus, the dynamic nature of multiple bio-behavioral systems and their interplay have to be taken into account using advanced statistical methods to achieve meaningful prediction. Our PERFORM project aims to develop such new statistical methods for multi-modal, continuous and densely collected data. Importantly, method development will occur in a quasi-experimental context where causal relationships between risk and outcome are partially known, and hypotheses exist about the biobehavioral mediators.
The project focuses on two main case studies: biological and psychological markers in relation to i) injury risk and fatigue in athletic populations and ii) mental health in at-risk populations. In both studies, individuals will be monitored accounting for psychological components while incorporating physiological and endocrine markers through continuous monitoring using wearable technologies. By analysing data such as cortisol levels, lactate levels, heart rate variability, and subjective mental health data, we aim to develop predictive models that can improve the efficacy of interventions and enhance recovery strategies.
The development of a robust prediction model will be at the heart of the project. The aim is to both accurately predict momentary stress levels as well as fatigue and injury risk, respectively, and to identify the main driving factors. We plan to achieve this by adapting statistically enhanced learning (SEL), a combination of flexible statistical modelling and machine learning, to the present context. SEL is tailor-made to combine multiple data sources and, in particular, allows integrating temporal dynamics into machine learning algorithms by means of well-chosen statistical estimators. In order to identify those factors that actually affect our outcomes, we will combine the resulting models with interpretable machine learning techniques to help mitigate the black-box nature of machine learning.
This interdisciplinary initiative integrates fields such as biopsychology, sports science, medicine, data science and mathematics to develop new SEL approaches in prediction of dysfunctional mental and physical states. These will enable the comprehensive analysis of multi-modal data, providing insights into the physiological and psychological processes underlying stress-associated mental health problems and fatigue and recovery. The project represents a bold step forward in personalised health monitoring and intervention.
By Prof. Numa BERTOLA (FSTM) and Prof. Jan LAGERWALL (FSTM)
The current management of existing civil infrastructure, such as bridges, relies mostly on subjective visual inspection. Such unreliable inspection leads to dramatic collapses, such as the Morandi bridge in Genoa in 2018, but also many early replacements with significant associated costs that could have been avoided. Data-driven structural-safety assessment tools, relying on sensing technologies, have been actively developed by the structural-engineering community. Nonetheless, such techniques, given sensors currently available, are also unreliable as they usually monitor the bridge only at single points, meaning that they can easily miss damage developing a few centimeters away of the sensor devices. Indeed, the crack development in concrete can be extremely localized, and structure failure can occur with little warning on the global bridge behavior.
It is thus crucial to develop new sensing technologies that are able to spatially monitor the concrete surface, which is exactly the aim of the BriCE project. This multidisciplinary project will leverage the recent progresses in materials science of the co-PI using Cholesteric Liquid Crystal Elastomers (CLCEs) as sensor sheet across concrete bridge surfaces. CLCEs have the ability to shift color with deformation, allowing easy detection and tracking of cracks in the concrete surface underneath.
By combining this new material, with an adequate camera-based tracking system, machine learning tools and advanced concrete-behavior modelling (as spear-headed by the PI), it is possible to develop a radically new method for tracking structural damage on concrete bridges. The convergence of materials physics, chemistry, computational tools and bridge monitoring allows the development of this new sensing solution. This new sensing approach presents the promising advantages of being scalable, inexpensive, durable, and providing a versatile solution for concrete bridge structural health monitoring. The audacious BriCE project can trigger a paradigm shift in infrastructure management by providing the needed sensing solution for safer and more economical decision making on bridge safety evaluation.
Assist. Prof Numa Joy BERTOLA
FSTMAssistant professor in Civil Engineering with a focus on concrete structures
By Prof. Anja LEIST (FHSE) and Dr. Patrick MAY (LCSB)
Dementia, an incurable neurodegenerative disease marked by memory loss, is increasingly significant in aging populations. Despite advances in understanding genetic and lifestyle risks, key challenges remain. First, we cannot yet reliably predict individual dementia risk, as current genetic and biomarker tests capture only a subset of cases. This is due to fragmented approaches that neglect holistic assessments combining clinical, lifestyle, and genetic factors. Second, the biological pathways driven by genetic and modifiable risk factors are not fully understood, partly because of a lack of reliable biomarkers for these pathways. However, recent large-scale proteome studies have shown that proteomic signatures can predict complex traits, capturing environmental factors beyond genetics.
PRECIDEM aims to tackle these challenges by developing comprehensive prediction models that integrate clinical, lifestyle, and proteomic biomarkers, alongside new insights from genetics, including lifestyle-GWAS data. The project will also use causal testing methodologies like Mendelian randomization to explore the interaction between genetics and environmental factors in dementia development. To achieve this, PRECIDEM will utilize three large datasets—UK Biobank, the Dutch Lifelines cohort, and Generation Scotland—for knowledge synthesis and validation. By combining expertise in dementia risk reduction, genetics, and proteomics, PRECIDEM seeks to advance precision dementia prediction and prevention. Ultimately, the project will help identify individuals at risk for dementia and enhance our understanding of its biological causes, paving the way for targeted preventive interventions.
By Prof. Harlan KOFF (FHSE) and Dr. Rachel RECKINGER (FHSE)
Mainstream industrialized markets have widespread unsustainable social, economic, political, and environmental implications (De Schutter, 2017), which permeate food systems worldwide. These systems are typically governed through regulation of the parts that deal directly ‘with’ food, i.e. inputsupply, agricultural production, technology, etc. The parts of food systems that revolve ‘around’ food,
such as trade, finance, education, etc. are often less regulated and not seen as fundamental parts of these systems (Reckinger et al., 2022).
This usually reinforces policy siloes and above all, maintains the unsustainable characteristics of global food systems that undermine distributive justice, such as concentration of power, prioritization of economic profitability, exploitation of food workers and the further depletion of already inequitably accessed resources. These inequities have perpetuated a system characterized by long supply-chains, mass production and overabundance in some places while other regions are characterized by scarcity, malnutrition, hunger. These traits are the logical consequence of a regime where care for people (in terms of accessibility, affordability nutrition and cultural appropriateness of food) and for the planet (in terms of soil health, biodiversity preservation, water stewardship, etc.) are not central, undermining defining commitments in the 2030 Agenda for Sustainable Development. Similarly, production subsidies and supply chain supports contribute to accelerated climate change and unhealthy consumption in many parts of the world.
Food systems lack balance and scholarship acknowledges that this is caused by incoherent policy frameworks for sustainability. What’s more, the segmented focus on hunger without addressing underlining systemic inequalities focalizes political attention on food security, which pits the need for increased food production against localized, values-based sustainable practices.
This proposed project addresses these challenges through the implementation of a policy coherence for sustainable development (PCSD) approach. Framed within the transformative sustainable development that defines the Sustainable Development Goals (SDGs), this proposal responds to three inter-related research questions: 1) what properties characterize a(n) (un)sustainable food system?, 2) what mechanisms can be identified to promote transformative change in socio-ecologically complex food systems? and 3) what policy innovations are needed to attain socio-ecological sustainability in food systems?
By Prof. Alexander SKUPIN (LCSB) and Prof. Alexandre TKATCHENKO (FSTM)
Ion channels are at the core of neuronal dynamics and their malfunction is associated with diverse neurological disorders including epilepsy and migraine. Despite intensive research, our understanding how modifications of the molecular structure caused by alternative splicing or mutations affects the channels’ functionality is rather limited due to experimental limitations with direct implications for drug discovery.
The project will address this challenge by complementary computational approaches and molecular biology experiments synergizing between the Luxembourg Centre for Systems Biomedicine (LCSB) and the Department of Physics and Material Science (DPhyMS) by combining AlphaFold structure predictions, molecular docking, machine-learning assisted molecular dynamic simulations and validation experiments. Based on our recently preliminary work on the Nav1.1 channel investigating the effect of genetic variants in the encoding gene SCNA1A associated with epilepsy and migraine, we will perform protein structure prediction and molecular dynamic simulations of the Nav1.1 variants to characterize the dynamic consequences of the corresponding mutations and cross-validate these findings with electrophysiology data. For the validated Nav1.1 variant models, we will perform in silico drug discovery by molecular docking and subsequent molecular dynamic simulations including long-range van-der Waals interactions to restore normal Nav1.1 activity in a disease-associated genetic variant and thus in a structure-specific manner. Most promising candidates will be validated by in vitro electrophysiology experiments.
Overall, the interdisciplinary project will combine approaches from computational and molecular biology, methodologies from physical chemistry and computer science to establish an innovative computational pipeline for structure (and hence variant) specific drug identification for ion channels with a proof-of-concept application to Nav1.1 in the context of the epileptic syndromes and migraine in a translational manner. This approach will pave the way for systematic analyses and in silico drug screening for other ion channel-based neurological disorders like neurodegeneration and build synergies between departments at the University including the current development of the medical school.
Assoc. Prof Alexander SKUPIN
LCSBAssociate professor/Chief scientist 2 in Modelling of Biomedical Data
By Prof. Anne GRÜNEWALD (LCSB) and Prof. Stéphane BORDAS (FSTM)
In MITO-MANIAK, we will develop an adaptive Bayesian computational model of astrocyte-neuron metabolic coupling that can be used to predict mitochondrial activity at unprecedented speed. Our model could serve as a computational drug screener for Parkinson’s disease (PD).
PD is a debilitating movement disorder that currently lacks neuroprotective treatment options. About 10% of PD cases are due to mutations in proteins which primarily act in mitochondrial processes. Interestingly, latest research suggests that the mitophagy regulators PINK1/Parkin are also involved in neuronal mitochondrial metabolism, which is intertwined with astrocytic metabolism. However, from the (mostly descriptive) data available to date, no concrete metabolic rescue strategy for dopaminergic (DA) neurons has emerged.
While these new metabolic insights could be a major clue towards disease-modifying drugs, state-of-the-art investigations require enormous experimental efforts. A step change for PD drug screening would be brought by a computational tool able to identify key metabolic pathways and modulators. This would, in turn, enable the selection of optimal wet-lab experiments and thus accelerate the search for a PD cure.
In MITO-MANIAK, (i) we will use multi-omics data to identify metabolic pathways disrupted in iPSC-derived neurons/astrocytes from PINK1-/PRKN-PD patients. (ii) We will create Bayesian computational models that simulate the impact of PINK1/PRKN mutations on mitochondrial function in DA neurons and astrocytes. (iii) We will construct multi-compartment models that simulate metabolic interactions between neurons and astrocytes. (iv) Finally, we will use these simulations to test the effects of metabolic interventions on mitochondrial function in DA neurons and validate the impact of selected treatments in vitro.
With our interdisciplinary approach, we will generate a platform that will facilitate high-throughput testing of mitochondrial metabolic agents in a cost- and labour-effective manner. Ultimately, we will utilise this platform for the development of an urgently needed drug that can prevent DA neuron demise in PD.
2023
By Prof. Luc NIJS (FHSE), Prof. Inès CHIHI (FSTM) and Prof. Luis LEIVA (FSTM)
Unlike athletes, musicians receive little or no education regarding the most effective ways to prepare their bodies and minds for the high demands of performing music. The SAMUSE project addresses this shortcoming in training and educating musicians. It will lay the foundations of a research-based professionalization of instrumental music education by developing innovative quantitative methods informed by Artificial Intelligence (AI) modeling strategies to characterize different skill levels in music performance. Such characterization is fundamental to understanding the music skill acquisition continuum and to deploying this understanding for the design of adequate and effective learning paths.
To this end, the SAMUSE project will introduce an integrative (multi-sensing measurements) and development-oriented (multiple skill levels) approach to the study of skill acquisition in music performance. It will elaborate a solid theoretical and methodological framework to identify and define the psychophysiological parameters (“embodiment indicators”) that change over time in relation to the different skill acquisition levels. By conducting a focused cross-sectional study, the physical, cognitive, and emotional engagement of musicians will be measured at different skill levels. The project will contribute to novel measurement and analysis methods in music performance research, allowing a more advanced and sophisticated characterization of skill levels in music performance, among other project outcomes. In addition, we will focus on the development of wearable and flexible sensors, which is a noteworthy research area that has captured the interest of numerous researchers. These sensors have the potential to be seamlessly incorporated into clothing, gloves, and accessories. For music performance research, wearable and flexible sensors could be designed to capture a wide range of data, including gestures and physiological responses.
The project will support further research on the design and evaluation of novel educational approaches (e.g., through longitudinal intervention studies), and on the development of advanced digital monitoring applications to enhance healthy and effective performance skill acquisition. This will ultimately support several research applications, such as quality enhancement in music education, multimodal sensor fusion algorithms, and user models. From an engineering standpoint, the project’s objective is to create innovative, smart, wearable, and flexible sensors designed for data collection during music performance. These sensors would prioritize user comfort, ease of use, efficiency, and environmental friendliness.
Assist. Prof Ines CHIHI
FSTMAssistant professor in Electrical Measurement and Sensor Technology
By Dr. Marian VAN DER MEULEN (FHSE) and Prof. Jorge GONCALVES (LCSB)
Recent advances in machine learning (ML) and artificial intelligence (AI) have made it possible to develop new methods for understanding brain function based on medical imaging. One very promising approach, which revolutionized the field of natural language processing by enabling tools like ChatGPT, is to use embeddings, which are low-dimensional representations of high-dimensional data, for example mapping text to a vector of numbers. Embeddings can be used to capture the relationships between different data modalities and feature dimensions, may it be spatial patterns, temporal dynamics, or even semantic correlations.
In this project, we will transfer the concept of embeddings to the domain of image analysis for studying brain function and cognition. By using embeddings, we create a lower-dimensional space which enables us to fuse different data modalities in this space, for examples MRI imaging of brains, blood-based markers such as proteins or cytokine levels, and results of psychological cognition testing – modalities that are originally quite “incompatible”. By projecting them to a common space using recent ML/AI techniques, novel joint analysis is enabled. To evaluate performance of the joint models and ensure that their “black box” approach is based on solid ground in the application domain, we will apply them first to the field of pain research.
This audacious project will yield outcomes in two domains: In the methodological / computational domain, we will develop techniques to efficiently apply joint embedding methods, as established in language processing, to other modalities revolving around medical imaging and cognitive science data. On an applied level in cognitive science, we will generate novel insights in the identification of multi-modal markers for cognitive/clinical states, in particular for pain. We will, for the first time, integrate cognitive and behavioral data with MR imaging information and blood-based markers in a large, joined analysis. This specific combination of data modalities is also highly relevant in neurodegenerative disorders like Alzheimer’s or Parkinson’s disease. As such, our focus on pain research provides an ideal testbed for opening audacious perspectives in analyzing cognitive processes reaching far beyond the current context.
2022
by Dr. Josh BERRYMAN (FSTM) and Prof. Michael HENEKA (LCSB)
Amyloid diseases in general have complex and often unknown etiologies, however inflammatory processes are a common feature of the large-scale killers, Alzheimer’s disease and Parkinson’s.
Inflammation is well-characterised as the typical direct cause of AA amyloidosis, and is likely to be a mediating process in the destructive interaction of SARS-CoV2 and amyloid disease. During inflammatory response, reactive oxygen and nitrogen species are produced which interact promiscuously with endogenous molecules, including aggregation-prone peptides. It is already known that inflammatory damage can strongly enhance aggregation and neurotoxicity of Amyloid Beta peptides (Aβ), however, typically for amyloid disease, the picture remains murky as the biochemistry of inflammation is complex, and protective effects have also been reported for similar oxidative inflammatory effects under different experimental conditions.
The purpose of this project is to examine the precise molecular and supramolecular changes driven by reactive species released during inflammation and quantitatively discriminate between harmful and beneficial inflammation-driven processes, tracing the thread all the way from atomistic inflammation-driven free radical chemistry up to amyloid disease in three-dimensional human tissue cultures and in mice. Interdisciplinary collaboration, such as the Institute for Advances Studies is designed to foster, is necessary to address this lengthscale-crossing feedback between biological processes at the atomic, molecular, supramolecular, cellular, and tissue levels which are typically studied piecemeal by very different communities with very different tools, leading to a lack of any complete and satisfying understanding. A quantitative multiscale description of these interactions will shed much-needed light on the etiology of neurodegenerative disease, and on its links to inflammatory conditions such as obesity and infection, which today are known of but very little understood. This project proposes to trace a complete and quantitative etiology leading from specific inflammatory stimulus to Alzheimer’s disease, which should provide valuable insight leading ultimately to new therapeutic approaches.
by Prof. Catherine JONES (FHSE) and Prof. Phillip DALE (FSTM)
To better understand social preferences, the Solar Living Lab develops camouflaged Photovoltaics (PV) and evaluates them using AR & city models.
Future renewable energy systems will noticeably change the appearance of our land and cityscapes. There will be a massive shift from predominantly invisible fossil fuel production infrastructures, which currently takes up ∼0.3% of land surface, to a photovoltaic (PV) and wind infrastructure accounting for ∼3 to 6% of land area in Luxembourg, Belgium and the Netherlands small to medium-sized countries. These land-use changes present new challenges and are often met with adverse public discourse, related to visual, social and environmental impacts entwined with the social-cultural values citizens attach to places. Our project will provide a framework for understanding how the renewable energy transition could be better accepted and what factors will improve the likelihood of acceptance.
Our hypothesis states that PV panels that fit with the look and feel of the local land/cityscapes will improve social acceptance, but this bring about challenges of: (1) how to develop coloured patterned PV panels that are camouflaged within their surroundings and yet maintain their energy yield ; (2) how to involve community stakeholders in the design of new energy environments within the preconditional boundaries required to generate a certain amount of energy in different neighbourhoods.
To test our hypothesis, we propose to investigate: (1) how to fabricate colour images with different resolutions using liquid crystals on PV panels with negligible performance loss ; (2) to evaluate the scaled-up results directly with stakeholders and citizens. We will build geospatial models for different options of PV installations at the neighbourhood scale, which will be evaluated via city models / augmented reality technology with citizens and stakeholders (researchers, energy sector representatives, housing and development decision makers, and local planners) within our SolarZukunft Living Lab.
Whilst using an embedded place-based theory with a social acceptance evaluation framework we will assess which PV scenarios are preferred and how they could be spatially distributed in a neighbourhood to reach a specific level of energy yield. From our results, we will be able to inform spatial planning processes on the visual and social impact of the renewable energy transition and support community visioning for the future.
Our research will provide scientists, decision-makers and citizens a head start on understanding the social, environmental and economic impacts of a distributed PV energy transition on our urban and rural landscapes.
If you would like to join our living lab please contact catherine.jones@uni.lu or phillip.dale@uni.lu.
by Prof. Rebecca TEFERLE (FSTM) and Prof. Ariane KÖNIG (FHSE)
Forest ecosystems can play important roles as habitats for diverse species, as drivers of bio-geo-chemical cycles including the water and nutrient cycles, they can promote water infiltration in soil and help the build-up of ground water bodies, and as such they can contribute also to the resilience of regional ecosystems against impacts of extreme weather events, such as droughts.
At the same time, individual trees can be vulnerable to predicted impacts of anthropogenic climate change, including old and new plant pests and diseases. The timely identification, mapping and characterization of trees has thus gained in importance for evidence-based and future-oriented management and policies. Traditional field-based inventory surveys are limited in scope and highly time-consuming and expensive to monitor the forests in the Grand Duchy of Luxembourg (GDL). A paradigm shift in forestry monitoring is called for, with forests needing to be understood as complex socio-ecological systems that require adaptive management and governance to make forests and forest management more resilient and sustainable. New technologies for remote sensing and citizen science promise innovative approaches that may be able to help respond to these new needs. The interdisciplinary project PIONEER explores the development of an innovative approach to produce timely, accurate and accessible evidence on forest ecosystems and their services by combining remote sensing and citizen science.
Firstly, PIONEER will investigate the use of endurance unmanned aerial vehicle (UAV) platforms, i.e., new systems that can cover up to 100 km2 per day, with light detection and ranging (LiDAR) and hyperspectral image (HSI) sensors to collect data on the forest canopy. The approach will rely on developing deep learning (DL) approaches for improving the extraction of known and new forest metrics. LiDAR data will be drawn upon to describe canopy architecture, encompassing the horizontal and vertical structure at scales of individual trees, while HSI data does so in describing physiological traits of individual trees from their spectral signature. The developed DL algorithms will extract 3D ITCs and integrate them with their spectral signatures for an efficient way to classify species, while providing a digital model for precision forestry to identify tree growth rates and health at a scale of individual trees, investigating spectral characteristics associated with early infestations, and providing the forest metrics that enhance monitoring and sustainability of forestry. A critical aspect for DL is the gathering of enough labeled bespoke training and validation data for successful and efficient classification, segmentation and identification models.
Secondly, a citizen science approach will be co-designed with experts, professionals, forest owners and citizen volunteers to allow the collection of complementary data from below the canopy that presents data on both the state of health of individual trees and estimated ecosystem services such as carbon sequestration and water infiltration in the ground. Furthermore, PIONEER will explore whether data for deep learning can be collected with citizen science. A citizen science tool set for participatory monitoring will help to enrich official data pools but also provides educational means that can be used by schools and in life-long learning offered as part of leisure activities to develop an appreciation of complexity and renegotiation of what is valued in the community.”
Both remote sensing and citizen science will be leveraged in tandem to develop a tree-centric approach to carbon density estimates, biodiversity, and forest diseases based on identifying individual tree crowns (ITCs) and species by utilizing the complementarity in these data sources. This combined approach promises to respond to new data needs for new official environmental accounting approaches considering ecosystems and ecosystem services as well as economic aspects that are being proposed at the level of the UN and discussed within the EU.
2021
by Prof. Jun PANG (FSTM) and Dr. Lasse SINKKONEN (FSTM)
Discovering structures of gene regulatory networks (GRNs) based on large-scale high throughput data is a fundamental research challenge in systems biology.
The inference of accurate GRNs from data is challenging not only because of the large number of components involved, but also their possible mutual interactions. The inferred network models can provide system-level understanding of the mechanism of cell transformations, which is crucial for the comprehension of the progression of complex diseases such as Parkinson’s disease (PD), and the affected cell types.
GENERIC takes an interdisciplinary approach towards GRN inference, integrating theories, techniques and tools from biology and computer science. It aims to devise data-driven deep learning models for inferring GRNs with high accuracy from single-cell gene expression data. In particular, with this proposed project we will be the first to develop novel GRN inference methods by exploiting neural relational inference (NRI) to discover latent interactions directly from data. This paves a new way for accurate and efficient reconstruction of comprehensive real-life GRNs. Our inference methods combine an iterative graph generation process and reinforcement learning into NRI in order to increase its efficiency and accuracy, and as well to incorporate prior biological knowledge of the studied GRN and well-established GRN structural properties.
The development of our methods will be driven and accompanied by application of the methods to single cell gene expression data from differentiation of human dopaminergic neurons (hDAns), a cell type with high biomedical relevance for example in PD. Moreover, the effectiveness and accuracy of the inferred GRNs will be validated in wet-lab using ChIP-seq and CRISPR-dCas9 inhibition experiments in hDAns derived from induced pluripotent stem cells (iPSCs). The newly identified networks will give rise to better control of hDAn differentiation.
by Prof. Stéphane BORDAS (FSTM) and Prof. Andrea BINSFELD (FHSE)
Our cultural heritage faces multiple threats. We are losing the story of where we came from. Be it through man-made or natural disasters, we may lose track of where the past is leading us. Archaeology and History, Art History, Data and Computational Sciences, and Geophysics (including Geology) are teaming up to build a new breed of forensics scientists who will “Reverse Engineer our Future”.
We set out to devise the new generation of image-guided multi-physics computational archaeology tools, which will enable us to digitally recover, record and preserve our cultural heritage. Uniquely, we will build advanced computer simulations, directly from state-of-the-art imaging and remote sensing data, to, in time, better understand the construction sequence, the role of artefacts and art pieces, and to imagine and simulate life as it took place in those locations. Through a fascinating case study in a post-conflict zone, namely Mosul, Iraq, we set out to demonstrate how recent advances in multi-physics-image-based modelling and simulation can support the virtual repatriation and visualisation of three-dimensional scenes, get to areas which humans cannot access and address research questions which could not have been answered before.
Computational Modelling and Simulation will help us, from incomplete data, to build the Earth’s Digital Archaeological Genome, reverse engineer our past to better understand the present day and (fore)see what our future holds. Our team of “archaeological geneticists” will become the new actors of “archaeology forensics” to shed new light on the crime scene of destroyed or threatened archaeological or cultural heritage sites. Keeping in mind the relatively short time frame of this project, our team will first focus on devising new smart adaptive point clouds to simulate the mechanics of archaeological sites with a controlled degree of accuracy and to exercise this idea on the actual site of Mosul, upon which our team has years of experience.
2020
by Prof. Silvia ALLEGREZZA (FDEF) and Dr. Patrick MAY (LCSB)
Within the last 15 years DNA sequencing and, in particular, Next Generation Sequencing (NGS) or Massive Parallel Sequencing (MPS) have revolutionised human genetics research. It is now possible to sequence a complete human genome inexpensively and more efficiently.
Importantly, the analysis of common Single Nucleotide Polymorphisms (SNPs) offers new opportunities for the so-called Forensic DNA Phenotyping (FDP), as well as forensic epigenetics. More concretely, FDP, exploiting predictive aspects of DNA, can draw inferences on concrete genetic aspects: a sample from the crime scene can offer information about appearance-related traits (hair, skin and eye colour or age), biogeographical ancestry, geographical origin, kinship, lineage (in general, relationships), behaviour or genetic diseases of the potential offender. Our interdisciplinary project intends to combine legal and genetics science to explore the future of FDP addressing the following research questions:
- What will be the predictive power of FDP in the coming years? How should forensics genealogy be integrated with FDP? How could the results of FDP be misunderstood in light of the involvement of non-genetic items in the establishment of certain traits?
- What are the next frontiers in terms of behavioural genetics? Can FDP predict more than external appearance? What is the impact of automated treatment of FDP or behavioural profiling? What is the potential impact on the current setting of criminal investigations?
- To what extent and under which conditions should FDP-related information be stored on databases? And what rules should govern interoperability of such databases?
- What is the role of private genetics companies in these fields? How can intellectual property rights be combined with judicial review and defence rights in criminal justice?
The innovative project will integrate academic Uni.lu fields of expertise together with law enforcement agencies as well as private actors in the field of genomics and NGS in an interdisciplinary project aimed at a long-term strategic field of research.
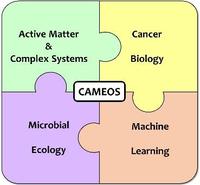
by Prof. Anupam SENGUPTA (FSTM) and Prof. Elisabeth LETELLIER (FSTM)
Notwithstanding the recent run of breakthroughs in the realms of microbiomes and cancers–two complex systems on their own–much remains unknown at their interface: The Cancer Microbiome.
A growing body of fundamental and translational research indicates that the associated microbiome influences–and potentially–regulates cancer progression, crucially impacting the choice and efficacy of diagnostic and therapeutic approaches. At the scale of a microbe, cancer is a spatially structured active soft material, either close to thermodynamic equilibrium, kBT (product of the Boltzmann constant, kB, and temperature, T) for solid tumours or out-of-equilibrium for most metastatic cells. The associated microbiome–an active matter system–elicits emergent geometry, order and topology that, crucially, regulate local biophysical properties. Taken together, the cancer microbiome is a coupled active complex system where emergent properties underpin oncogenic attributes and progression.
Yet, to date, such a framework remains unexplored, with bulk of existing studies focusing independently on one or the other. Little is known if and how biophysical emergence regulates the dynamics of cancer microbiome, leaving open a major chasm in our efforts to predict cancer progression, and innovatively harness this promising, yet unexplored functional parameter to advance diagnostic and therapeutic tools. Using a bottom up multi-scale experimental approach, CAMEOS pioneers a new line of cross-disciplinary research on cancer microbiome that lies at an exciting interface of physics, biology and machine learning (ML). By leveraging the synergy between the PI-s, and their upcoming collaborations within the UL (DCS, DPhyMS & LCSB), CAMEOS will discern the role of microbes–both direct and indirect–in shaping cancer phenotypes and micro-environments, in relation to microbial species and physiology. Focusing on colorectal cancer (CRC) as model system, CAMEOS will characterise the CRC and matrix physico-chemical properties, concomitantly with those of the associated microbes using on-chip and organoid- based platforms. A combination of advanced visualisation and biomolecular assays will capture the micro-scale dynamics, which using ML-based analysis tools, will reveal how microbes regulate CRC and matrix properties, ultimately feeding back into the dynamic microbial organisation and metabolism.
Crucially, through time-series data, CAMEOS will be in a unique position to generate seminal data that will link CRC-matrix transformations, in real time, with microbial distribution and physiology, revealing emergent feedbacks that underpin sessile-to-metastatic (benign-to-malignant) changes. This original, ambitious, cross-disciplinary endeavour will break new grounds by presenting a novel integrated mechanistic framework that will uncover the role of nonlinear interactions, feedback and emergence in shaping the fitness and resilience of cancer-microbe ecosystems, thereby heralding innovative approaches which will harness emergent properties for the next generation of cancer prevention and management.
by Prof. Luca RATTI (FDEF), Prof. Claus VÖGELE (FHSE) and Prof. Anna KORNADT (FHSE)
Prior to the onset of the Covid-19 pandemic, the debate on the future of work revolved around two concurrent narratives: the omnipresent automation of working environments and the emergence of new forms of work, facilitated by technology.
Research on workers’ fundamental rights and wellbeing, therefore, was mainly focused on digitalisation-driven concerns. With the start of the pandemic, such focus proved to be incomplete since reality turned towards unprecedented perspectives. Lockdown measures were introduced globally. In all European countries, essential workers were overexposed and most intellectual workers suspended or confined to homeworking. This initiated a radical change in the working paradigm: the matrix of work – namely the workplace – unexpectedly disappeared in its original features, leaving working people and employers alike without a stable anchor. Some policy makers are attempting to address such changes prematurely by suggesting the introduction of new laws (e.g. the legal right for a fixed number of home office days, as is the case in Germany). Actions are clearly called for, but should be based on empirical evidence.
The W@W Project aims to provide this evidence by addressing some crucial questions: How does the Covid-19 pandemic impact the concept and notion of workplace? How will telework be regulated? How will employees’ privacy at work be preserved? How will wages and productivity be measured? How will Covid-19 related changes to the work-environment and changes to its regulation affect employees’ health and well-being? How will the diversity of the workforce in terms of age and resources impact those relations? We will investigate these questions in six European countries (Luxembourg, Germany, France, Italy, Spain, and Sweden), through a legal analysis of the regulatory measures taken and their implications. This will be empirically complemented by data from a representative, longitudinal survey, which tackles the individual consequences of the measures in the different countries. In addition, we will conduct a case study with a Luxembourg-based industrial partner. We will integrate these findings and propose policy measures by introducing the concept of the “work-sphere”, which might replace the workplace as we know it.
The current pandemic continues to severely impact our way of life in all contexts and at all levels, individual societal and globally. It may be that once it is over, we will return to normal. Business as usual, regulations as usual, wellbeing as usual. Or maybe not. This project will help us to be prepared.
by Prof. Joana MENDES (FDEF) and Prof. Anna-Lena HÖGENAUER (FHSE)
The role of European Central Bank (ECB) in monetary policy and in European Economic and Monetary Union (EMU) law and politics has changed fundamentally in the past decade.
Just as during the sovereign debt crisis, in 2020 it is again at the forefront of economic responses that are essential to preserve the Eurozone, and, many claim, the EU itself. While it has so far been successful, the evolution of the ECB remains deeply problematic, both in terms of legality and legitimacy. It faces a conundrum of independence and democracy that remains unsolved. EU legal and political science scholarship has hitherto avoided addressing the problems that the ECB’s redistributive policies pose to democratic legitimacy in the EU, not least because of how they fundamentally constrain Member States’ economic policies.
EMULEG proposes to re-define the institutional framework of the EMU. It analyses whether the ECB’s independence is too high in light of the increasing polarisation of opinions on its policies and the growing importance of its decisions, and whether there are better alternatives that allow for a rebalancing of independence and democratic legitimacy. For this purpose, we will compare the legal framework of the independence of the ECB to three other central banks, as well as the institutional reality of accountability towards political institutions and the public. The aim is to formulate advice on possible reforms including both changes to the ECB’s legal framework and the institutional practice of central bank accountability in the EU, to find a better balance between effective policies in the pursuance of collective goals and democratic legitimacy.
Dimitrios is focusing on the adequacy of the independence apparatus of the European Central Bank (ECB) in light of the Bank’s adapted role during the last decade and the concomitant democratic legitimacy challenges. From a political science perspective, Dimitrios seeks to compare the model of the ECB with the models of other central banks with a view to identifying policy lessons. His research concentrates on the evolution of economic policy beliefs of central bank officials and other stakeholders and their impact on institutional arrangements. The study includes the methods of Organizational Discourse Analysis, Elite Interviewing and Comparative Analysis.
Nikolas is focusing mainly on the ECB’s role within the European Economic and Monetary Union (EMU) as established in the EU legal framework and as developed through the ECB’s institutional practices especially since the financial crisis erupted. Given that the ECB’s role within the EMU has raised issues of democratic legitimacy, this study will rethink this role, especially by comparing it to the role of other central banks. The research will end up with devising reform proposals to strengthen the democratic accountability of the ECB. These proposals will be linked with the formulation of advice on a redefinition of the ECB’s role within the EMU and on a possible redesign of the EMU’s institutional framework to address the democratic legitimacy concerns.
by Prof. Jan LAGERWALL (FSTM) and Prof. Holger VOOS (SnT)
With TRANSCEND we will create the foundation for an infrastructure that currently does not exist, yet is dearly needed to keep up with emerging trends in robotics and automation.
The long-term vision is to enable safe cohabitation of robots and humans in everyday contexts, from the intimacy of our homes to construction sites and busy cityscapes. At the heart are the remarkable optics of Cholesteric Spherical Reflectors (CSRs), a versatile new optical component invented in the team, exhibiting omnidirectional reflectivity in a narrow wavelength band with circular polarisation. This enables detection from any direction even in visually complex and dynamic environments, without false positives. By tuning the reflection band outside the visible spectrum, CSRs are undetectable by the human eye, hence aesthetically non-intrusive. We will coat surfaces with CSR-based fiducial markers—effectively invisible QR codes—that link the physical world to its digital representation, allowing Machine–to-Machine (M2M) and Environment-to-Machine (E2M) communication that is reliable, inexpensive and extremely energy efficient. Our technology will assist navigation and real-time trajectory optimisation for robots (individual or in swarms), robot-assisted construction and responsible deconstruction and recycling, supporting the circular economy.
With such broad scope and audacious ambitions, it is clear that TRANSCEND is exceptionally interdisciplinary. The project is feasible thanks to intimate collaboration of two PIs with highly complementary expertise, in materials physics (FSTM) and robotics (SnT/FSTM), supported by international experts in chemical engineering (TU Eindhoven, Netherlands) and construction of built environment (New Jersey Institute of Technology, USA). To ensure a lively interdisciplinary communication along with a vital understanding among the team members about the challenges faced by the others, two PhD students will be recruited simultaneously, forming the core of a coherent team throughout TRANSCEND. Both students will be co-supervised by both PIs, and both will do secondments to the international collaborators.
by Prof. Alexander SKUPIN (LCSB), Prof. Jacques KLEIN (SnT) and Prof. Massimiliano ESPOSITO (FSTM)
Epilepsy is the fourth most common neurological disorder characterised by recurrent seizures and a rather heterogeneous clinical appearance with 30% of patients not responding to available drug treatments.
In the IDAE project, we address this challenge by an interdisciplinary and translational approach combining biomedical expertise and deep phenotyping in zebrafish models for epilepsy with advanced data analysis methods and mechanistic metabolic modelling. In particular, we will use interpretable machine-learning methods to integrate available heterogeneous data sets of brain dynamics, brain composition and metabolic profiles of diverse genetic zebrafish models to reveal underlying disease mechanisms which will identify a mechanistic genotype-phenotype relation and pave the way for improved patient stratification. For this purpose, we will re-analyse local field recordings of zebrafish models and microscopy time course data and integrate these seizure characteristics with brain composition deciphered from single-cell RNA sequencing data. The brain dynamics will be subsequently linked to specific metabolic activity identified by metabolomics and characterised by metabolic networks, which will then be used to perform mechanistic metabolic modelling to identify potential biomarker signatures. Based on the deep phenotyping in zebrafish, we will eventually apply the developed stratification strategy to human EEG data and provide metabolic profile biomarker candidates to be validated in future translational approaches.
Overall, the project will develop new approaches for mechanistic data integration in biomedicine and apply them to heterogeneous data sets on epilepsy to facilitate improved patient stratification.
Assoc. Prof Alexander SKUPIN
LCSBAssociate professor/Chief scientist 2 in Modelling of Biomedical Data
2019
by Prof. Mark D. COLE (FDEF) and Prof. Yves LE TRAON (SnT)
Artificial Intelligence (AI) is reaching out into all sectors and the law is no exception. Machine learning methods are being discussed as potential substitutes for human interaction or decision or at least for creating results on which human decisions are based.
In the area of law and its application, this can start for example with police investigations, range from the use of analysis in the prosecution to the final judicial decision-making, or from a client-lawyer relationship to the decision about damages in a civil procedure. Although there is still a long way to go before human intervention in the field of law could be replaced, first use cases show already now that there is an inherent danger in using AI solutions to replace analysis of a specific situation and possible predictions derived from it when attaching legal consequences to such decisions: the data used may be biased or the way they are applied may have unintended consequences and therefore the results can be unfair, or – in terms of the law – unjust. The area of law in this context is only a possible example to illustrate the research project’s goal: firstly, testing machine learning (ML) applications, which are used to replace or as a basis of human decision-making, in order to identify whether it is technically possible to get adversarily unfair results, and, secondly, to then be able to conclude whether what is technically possible is overall desirable or not. If testing of existing AI methods and models used e.g. in the context of law as described above, show that decision outcomes are worse in light of the originally perceived goal (just/unjust) than if they are based on human intervention only, or if the outcome even contradicts legal or moral assumptions (e.g. fairness by being non-discriminatory), then a (technically) possible use of AI may still not be a favourable option.
In challenging the appropriateness of ML in the context of legal decision-making, but possibly also in other fields such as political decision-making, the ultimate goal is to identify in which cases and to what extent it is “safe” to rely increasingly on such solutions and where the limits are. The project shall not have the goal of programming a specific algorithm that can be used in the legal domain.
Nor is the idea to analyse completely a specific legal domain such as criminal law and identify the specific problems in that area. What is needed is to define concrete cases with which it can be proven that a problem with the ML exists and there is no perfect solution to fix it. In that regard, the project shall be exploratory in nature, by showing what type of testing is necessary to find out deficiencies of AI systems – in this regard it relies on computer science expertise – and what consequences this has from a legal perspective. The latter is twofold: on the one hand, the domain of law and the judicial decision-making process will be used as blueprint for an area in which – possibly with good reason – there is quite some scepticism about using ML. On the other hand, legal norms such as fundamental rights will be the scrutiny benchmark to decide whether the outcome of testing means an AI system is insufficient. With this, the project shall give the basis to then further systematically research specific domains, specific AI solutions or specific testing methodology.
In the meanwhile, another aspect of the project is to present interim findings to a general public via an open conference as there is an increasing awareness of “AI entering everyday life” whilst for many this is a fearful prospect – and thereby there is a need for science to contribute to the societal discussion in Luxembourg.
Prof Yves LE TRAON
SNTDirector of the Interdisciplinary Centre for Security, Reliability and Trust
by Prof. Anja LEIST (FHSE), Prof. Paul WILMES (LCSB) and Prof. Rejko KRÜGER (LCSB)
Dementia is one of the greatest scientific, medical and socioeconomic challenges of our times, as it affects more than 46 million people worldwide, with incidence numbers projected to double within the next 20 years.
There is no medical treatment to prevent, halt or reverse the progression of neurodegeneration underlying dementia. Educational gradients in the onset and progression of dementia on top of the contribution of innate cognitive abilities suggest a role of socioeconomic factors in the development of this syndrome. At the same time, recent evidence for dysregulation of the gut microbiome in patients with dementia indicates that the microbiome may play a role in modulating brain function linked to neurodegeneration and suggests the presence of a diet-microbiome-gut-brain axis. Interestingly, socioeconomic factors can also influence food choices, which in turn may impact the microbiome composition. However, the underlying mechanisms of how the microbiome might contribute to neurodegeneration remain unclear, and little is known on how the composition of the gut microbiome is modulated by environmental and socioeconomic factors.
The proposed Audacity project, MCI-BIOME, aims to further our knowledge of the relationship between socioeconomic factors, diet, and changes in the gut microbiome in relation to Mild Cognitive Impairment (MCI). MCI is considered a prodromal stage of dementia and represents a transition state between normal cognitive ageing and dementia. We focus on MCI as, at this stage, identification of individuals at highest risk to progress towards dementia for potential preventive interventions would be most relevant. The project relies on a unique interdisciplinary team composed of clinical experts in neurodegeneration, specialists in socioeconomic research, and specialists in high-resolution microbiome characterisation (integrated multi-omics) and big data analytics. Samples and data to be collected include stool samples of 60 MCI patients of the Programme Démence Prévention (pdp) and 60 healthy controls (in terms of cognition) as well as relevant information on demographic, socioeconomic, and diet-related variables. The main objectives of the project are:
- to develop a novel interdisciplinary framework at the interface between social sciences, neurology and microbial ecology to open new research horizons and to strengthen the University’s excellent research profile,
- to develop a multivariate microbiome biomarker model for MCI based on integrated multi-omic data by comparing MCI patients to a group of healthy controls,
- to explore how microbiome characteristics differ between sub-groups defined by socioeconomic factors including education and diet,
- to build a research infrastructure that will be vital for acquiring high-level follow-up funding for future projects including the analysis of progression of MCI in relation to microbiome shifts and the validation of the microbiome biomarker model in the large Luxembourg-based cohort of the National Centre for Excellence in Research on Parkinson Disease (NCER-PD) including individuals with and without MCI and dementia.
by Prof. Conchita D’AMBROSIO (FHSE) and Prof. Alexandre TKATCHENKO (FSTM)
We propose to bring together machine learning approaches, physics-inspired descriptors, and the economics of wellbeing to address questions broadly related to predicting life satisfaction and health of individuals in a data-driven manner.
In the context of improving individuals’ life, one is often faced with the question of ordering individual/societal parameters (i.e. health and wealth) in terms of their importance. Up to now, state-of-the-art approaches relied on linear regression with very low Pearson correlation coefficients (R^2=0.2-0.3). The main goal of this project is to apply modern nonlinear machine-learning techniques to data on individual and social wellbeing with the aim to:
- understand the structure of the data and signal/noise ratio of many existing datasets,
- go significantly beyond linear regression with kernel-based methods and neural networks to search for multi-property correlations,
- assess different descriptors of individuals and metric definitions in ‘individual spaces’. To our knowledge, such fundamental ‘first-principles’ approaches to data analysis and nonlinear regression are only now starting to be applied to data on individual and social wellbeing, hence our project is timely and of potentially substantial impact.
by Prof. Andreas FICKERS (C2DH), Prof. Emma SCHYMANSKI (LCSB) and Prof. Laurent PFISTER (LIST)
There is hard evidence that global change is having severe and persistent impacts on environmental systems – eventually compromising socio-economic development in many regions of the world.
Luxembourg is far from being sheltered from these threats. There is an pressing need for a better understanding of the involved processes, interactions and feedbacks, both via a better understanding of past evolutions and the development of new prediction tools. Here, we propose the “Luxemburg Time Machine” (LuxTIME) project for exploring radically new ways for analysing and interpreting factual evidence of the past. We intend to build an interdisciplinary framework for investigating “big data” of the past, inspired by the conceptual premises of the “European Time Machine” Flagship project (see point 2), While being a high-risk interdisciplinary project, we see multiple avenues for high reward. Within Luxembourg, LuxTIME has the potential to function as a “team building project” for the Team Luxembourg (LIST, LISER, LIH, University), fostering the energies and skills across two interdisciplinary centres at the University in cooperation with LIST – integrating historical, informatics, environmental and health expertise that can easily be expanded at later stages.
By building a digital dataset that will include information from three different fields and scientific perspectives, namely eco-hydrology, medicine and history, LuxTIME will use a local showcase (i.e. the industrialisation of Belval / Minette region) as a testbed for methodological and epistemological reflections on how to study the impact of environmental changes on the health of the local population in a long term perspective. In combining past evidence from eco-hydrological studies (informing on water and pollutant sources, flow paths and transit times; non-stationarity of hydrological systems, and topographic / geological transformations), medical records (describing disease patterns, mortality rates, social/psychological well-being) and bio-chemical data (based on imaging and mass-spectrometry techniques as well as digital forensics on teeth) and history (archival sources documenting economic, social, political and cultural changes), LuxTIME will eventually study the past in completely new ways.
By mixing “contextual information” based on archival evidence with “scientific evidence” derived from chemical, biological, or medical investigations, the project explores new ground in interpreting “big data of the past” in a truly interdisciplinary setting. The Belval-case is meant to critically test the analytical potential of a multi-layered research design which – this is the mid-term ambition – can be expanded into a national case study; that is the building of a real “Luxembourg Time Machine” including many different kinds of data from many different institutions (such as demographic, economic, climate and socio-economic data from LISER, STATEC etc.).
Prof Andreas FICKERS
C2DHDirector of the Luxembourg Centre for Contemporary and Digital HistoryProf Emma SCHYMANSKI
LCSBDeputy Director of LCSB, Full professor in Environmental Cheminformatics-
LIST
Prof. Dr. Laurent Pfister
LIST and Affil. Prof. at Uni.luHead of ENVISIONEnvironmental sensing and modelling