Thibault Simonetto combined applied research with a top-tier academic publication. He details the challenges he faced and the lessons he learned from this specific approach.
When Thibault Simonetto began his doctoral research with BGL BNP Paribas, he faced an intriguing challenge. The bank was already using artificial intelligence to streamline its operations, but there was a critical blind spot – no one knew how these AI systems would handle unexpected data. His solution would not only transform how we test AI systems but also earn recognition at NeurIPS, one of the world’s most prestigious AI conferences.
What was the core focus of your research?
“The project began four years ago through our partnership with BGL BNP Paribas. We identified a critical challenge: while the bank was already using AI models to automate its processes, we weren’t sure how robust these models were when faced with unfamiliar data. We developed a method to test these models against ‘evasion attacks’ – attempts to force incorrect decisions by introducing noise into the input.
What makes our approach unique is our focus on generating realistic test data that follows actual financial constraints. For example, we ensure that maximum transaction amounts always exceed average amounts. We later expanded this approach to other fields like cybersecurity and healthcare using open-source data.”
Combining applied research with an A* paper is unusual. How did you achieve that?
“The key was our collaborative relationship with BGL BNP Paribas. They gave us the freedom to explore the problem, without strict time pressures. We started by understanding BGL’s specific challenges, then researched similar problems in academic literature. Then, we followed an iterative approach – first testing our solutions on our research infrastructure and public data, then applying them in collaboration with our partner to banks’ use cases. This approach gave us the flexibility to publish while ensuring our research remained practically relevant.”
‟ This approach gave us the flexibility to publish while ensuring our research remained practically relevant”
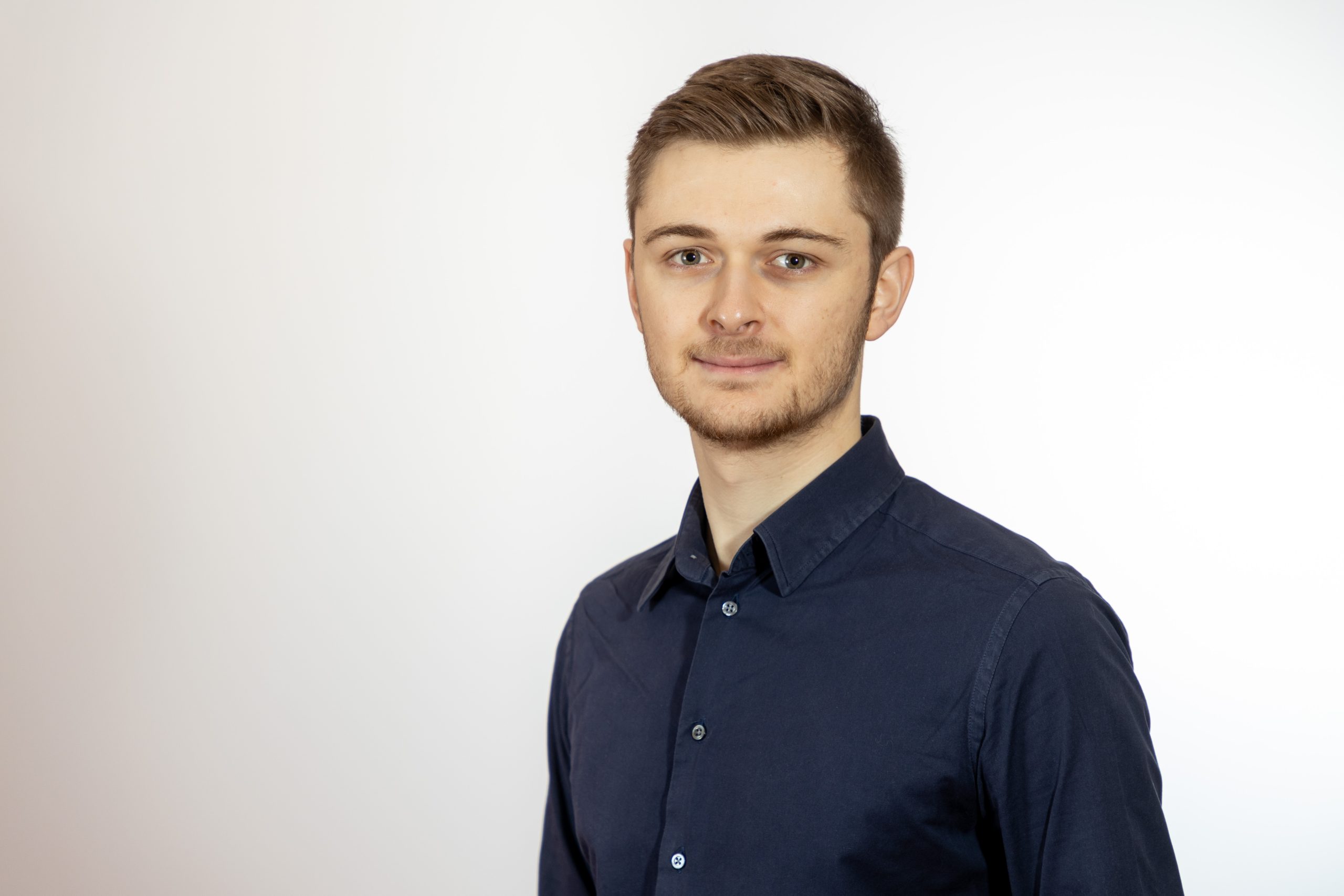
Postdoctoral researcher
How did you bridge the gap between theoretical and practical aspects?
“The fundamental problem we worked on had mostly been studied in computer vision, using theoretical frameworks and assumptions that don’t hold up in the real world. We focused on developing realistic evaluation scenarios for AI models, then developed novel methods that incorporate real-world constraints.”
What role did interdisciplinarity play in your research work?
“Our priority was to create realistic test data, and this required deep domain expertise. In the financial sector, we worked closely with experts at BGL BNP Paribas to define what constitutes realistic data for our experiments. Their insights were key in ensuring our tests reflected real-world scenarios.”
What advice would you give to doctoral students hoping to combine applied research with top-tier academic publications?
“First of all, don’t give up. Publishing research takes time, especially when working with industry partners. You need to develop a project that develops innovative scientific solution to address practically relevant problems. This means convincing the academic community that your industry-inspired problem is significant and broadly relevant – not just for your partner but for the entire field. In AI specifically, this often means demonstrating your solutions across various open-source datasets in different domains.
At the same time, you must show your industrial partner how your scientific approach applies to their specific needs and can deliver practical benefits. The upside is that when you succeed, your research has immediate real-world impact – it’s guaranteed to be used in industry from day one.”
About Thibault Simonetto
Thibault Simonetto is a postdoctoral researcher in the Security Design and Validation (SerVal) research group. His research interests are in machine learning security and reliability, focusing on the continuous delivery of robust and trustworthy machine learning-based systems. He completed his PhD in collaboration with BGL BNP Paribas, defending his thesis, “Enhancing Machine Learning Robustness for Critical Industrial Systems: Constrained Adversarial Attacks and Distribution Drift Solutions,” in October 2024. His work aims to bridge the gap between academic research and industrial applications, ensuring the secure deployment of ML technologies in critical systems.