NETCOM: Using non-equilibrium thermodynamics to optimize the energy demand of computation
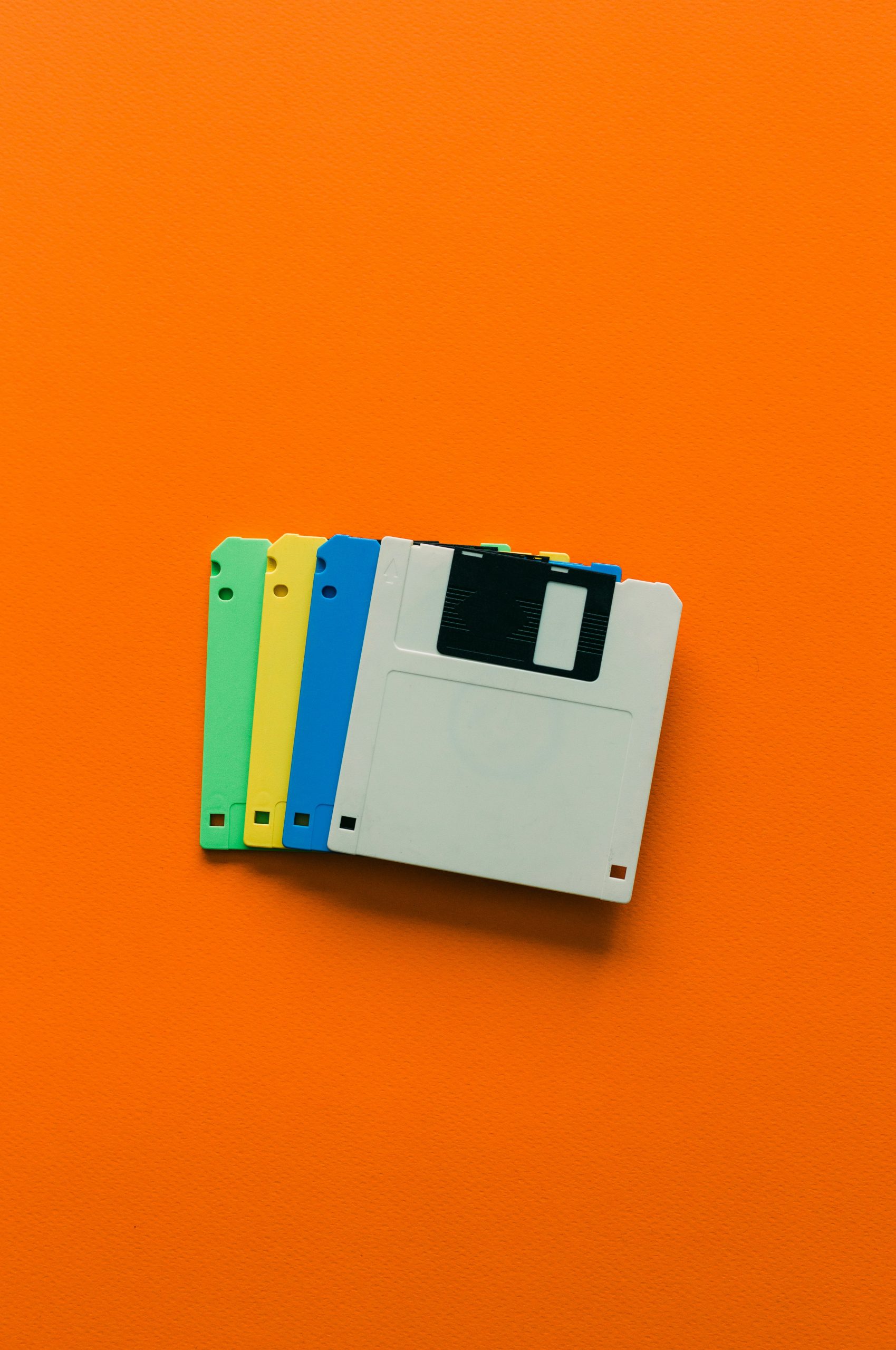
The project at a glance
About
The digital world is reported to consume 3-4% of the world power consumption, and increasing (9% per year), of which 20% is due to data centres [Fer18]. Data centres play a vital role in today’s cloud computing workflow and saving energy is one contribution to reduce climate change. This project aims to analyse and optimize the power demand of data centres through power and efficiency metrics derived from non-equilibrium thermodynamics. Abstraction of a data centres and even software into a network with different nodes will allow stochastic simulations of different workloads at a packet level analogous to chemical reaction networks and single-electron devices, allowing optimization of the energy. We intend to create power and efficiency metrics for several machine learning algorithms at different stages by modelling the spreading and usage of information as a thermodynamics process. The focus on particle flow of non-equilibrium thermodynamics, prompts an analogy with the communicating processes of a distributed system. Using the information-energy equivalence in stochastic thermodynamics, we can look at improved metrics for power and efficiency. In addition to energy-efficiency, the theoretical implications can also lead to program redesign, by simplifying the program’s complexity and data requirements. The gained insights can be beneficial to the data centres (by better allocating resources to computation), but also to the programs themselves, when modelled as physical systems. Closely connected with the optimization of machine learning efficiency is the significance of data context. For humans, context allows them to dismiss data and unlearn behavior and for proper evaluation of the context in machine learning the role of dismissing data and unlearning is needed. Lastly, I will propose simple code and workshops for the communication and acceptance among the general public of machine learning algorithms and their context.
Organisation and Partners
Faculty of Science, Technology and Medicine (FSTM)
Institute for Advanced Studies (IAS)