Taking advantage of a long-standing collaboration with the Wuhan University of Science and Technology, researchers from the Luxembourg Centre for Systems Biomedicine (LCSB) at the University of Luxembourg conducted a study based on blood samples from Chinese COVID-19 patients. They developed machine learning tools to identify crucial biomarkers of disease severity. Their results, recently published in the scientific journal Nature Machine Intelligence, highlight three parameters that can be used to predict the survival of individual patients with more than 90% accuracy: the levels of lactic dehydrogenase, high-sensitivity C-reactive protein and lymphocytes present in the blood.
The number of COVID-19 cases is putting a high pressure on healthcare services worldwide, making accurate and early clinical assessment of the disease severity vital. Unfortunately, there is currently no available biomarker to distinguish patients who require immediate medical attention. To support decision making and logistical planning in hospitals, LCSB researchers and collaborators from Huazhong University of Science and Technology, Wuhan University of Science and Technology and HUST-Wuxi Research Institute focused on developing a simple formula to predict patients at the highest risk, allowing them to quickly benefit from emergency care and potentially reducing the mortality rate.
This study leveraged a database of blood samples collected from 485 patients of the Tongji Hospital that admitted most severe COVID-19 cases in Wuhan. The clinical outcomes of these patients were followed up to 24 February: 298 recovered from the virus, while the remaining 187 died. “We retrospectively analysed the multiple blood samples taken throughout their stay at the hospital to identify and validate robust and meaningful markers of mortality risk,” explain Prof. Jorge Goncalves, head of the Systems Control group at the LCSB and co-author of the publication.
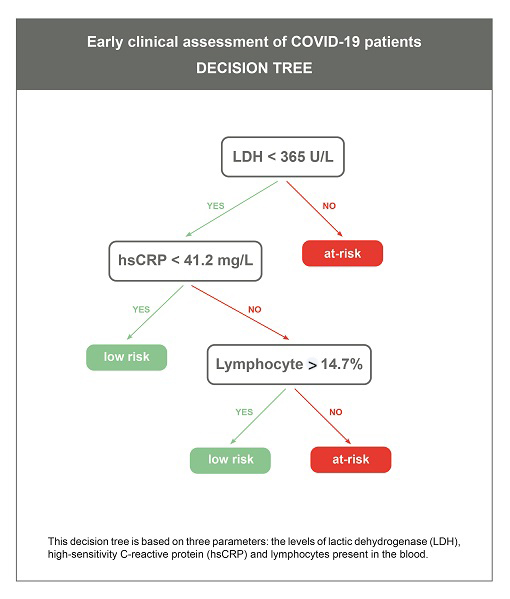
The researchers used a mathematical modelling approach based on state-of-the-art machine learning algorithms. Out of 84 clinical measurements, their model selected three key features. First, the concentration of lactic dehydrogenase (LDH): an increase in this enzyme is regarded as a common sign of tissue damage. The second parameter is a higher level of the high-sensitivity C-reactive protein (hs-CRP), which reflects a persistent state of inflammation. A low count of lymphocytes, one of the main types of immune cells, constitutes the third biomarker. For each of them, the researchers defined specific thresholds above or under which patients are at risk.
“The next step was to construct a simplified and clinically operable tool,” details Dr Laurent Mombaerts, a LCSB researcher who was involved in the study. “We created a three steps decision tree, validated it using the data collected in Wuhan and showed that this simple model can accurately predict the outcome of patients, regardless of their original diagnosis upon hospital admission.” On average, the model could predict the outcome of patients about 10 days in advance with a 90% accuracy.
As they had to strike a balance in order to keep the model practical and easy to interpret, the researchers are looking forward to testing it on larger datasets in order to increase its accuracy. Nevertheless, the study identified predictive and potentially life-saving biomarkers of disease severity. The three key features – LDH, hs-CRP and lymphocytes – can easily be collected and, in crowded hospitals with shortage of medical resources, this simple decision tree can help to quickly classify patients. In China, a website using this model is already available: doctors can simply enter the values measured for the three parameters and get the predicted outcome for the patient concerned. “It goes beyond identifying high-risk factors. It provides a simple and intuitive clinical test to recognise high-risk patients before irreversible damages occur,” emphasises Prof. Jorge Goncalves. This work also paves the way for using machine learning methods to help triage during large scale explosive epidemic such as this one.
Reference: Yan, L., Zhang, H., Goncalves, J. et al. An interpretable mortality prediction model for COVID-19 patients. Nat Mach Intell (2020). https://doi.org/10.1038/s42256-020-0180-7