Every five minutes, somewhere in the world, someone creates a new deepfake. As this AI-powered threat grows, researchers are developing equally powerful tools to fight back.
In 2024, a deepfake attempt occurred every five minutes, according to a report by the Entrust Cybersecurity Institute. The report shows a worrying trend. Fraudsters mix new and old techniques to beat new defense strategies. Criminals use stolen personally identifiable information (PII) from data breaches or phishing. Then, they create hyper-realistic synthetic identities.
Deepfakes use artificial intelligence to create convincing but fake videos, images, or audio. They can make people appear to say or do things they never did, from celebrity impersonations to fake news videos featuring political figures. The technology has advanced so quickly that spotting fakes has become increasingly difficult for the human eye.
The good news is that researchers are using powerful AI models to respond to these AI threats. Researchers at the University of Luxembourg’s Interdisciplinary Centre for Security, Reliability and Trust (SnT) have been developing advanced AI models to detect deepfakes since 2022.
Their goal is clear: make deepfake detection faster, more accurate, and more resilient against evolving threats.
The Role of SnT in Deepfake Detection
In 2021, scientists partnered up with Post Luxembourg to develop an algorithm to detect face-swap deepfakes that was more robust and effective than the solutions available on the market at the time. Building on this success, the research was expanded under the Luxembourg National Research Fund (FNR) BRIDGES programme, followed by an industrial fellowship with Post Luxembourg, concluding in March 2025. These initiatives have helped refine deepfake detection methods, allowing researchers to tackle increasingly sophisticated AI-generated content.
How AI Detects Deepfakes
To identify deepfakes, deepfake AI tools (or AI models) must be trained to detect inconsistencies in photos and videos – subtle details that bear the mark of AI-generated artifacts. For instance, generative AI models often struggle with fine details like hands and fingers, making these areas prime indicators of forgery.
AI models are usually trained through a method known as binary classification, where data is categorised into two groups: real or fake. For instance, researchers present the model with a series of images, each labelled as either real or fake. Through repeated exposure, it gradually learns to recognise patterns that distinguish authentic images from manipulated ones. However, this approach has a drawback: during training, the model tends to also learn patterns in the data that are not important.
“For example, if a model is trained on images that mostly have a white background, it might fail when trying to analyse photos with black backgrounds,” explains Dr. Enjie Ghorbel, a researcher in the Computer Vision, Imaging, and Machine Intelligence Research Group (CVI2). This issue, known in deep learning as a generalisation problem, limits the model’s ability to adapt to new contexts.
“Our research found that, instead of training models to separate fake from real, we could focus on teaching them to look for real data only,” says Ghorbel. “If the data examined doesn’t align with the patterns of real data, it means that it’s fake. This approach makes the model more robust and agnostic to various types of deepfakes. We explored this research direction first with images, then with videos,” she adds.
In their research, the CVI2 researchers – Prof. Djamila Aouada (PI), Dr. Enjie Ghorbel, Dr. Anis Kacem, PhD students Van Dat Nguyen and Nesryne Mejri, as well as postdoc researchers Dr. Marcella Astrid and Dr. Niki Foteinopoulou – had to develop ever more effective logical arguments to counter the increasingly sophisticated methods used to create deepfakes. These included training the AI to focus on vulnerable areas in images and checking the synchronisation between audio and video tracks.
“By adding other learning objectives, we have achieved a higher level of generalisation in our models,” explains Ghorbel.
The Challenge of Fully Synthetic Data
As deepfake AI advances, a new challenge has emerged: fully AI-generated images and videos. Unlike traditional deepfakes that modify existing media, these are entirely synthetic. This shift makes detection even more difficult. The photo below shows two individuals. But only one really exists, the other is AI-generated. Can you guess which one is real?
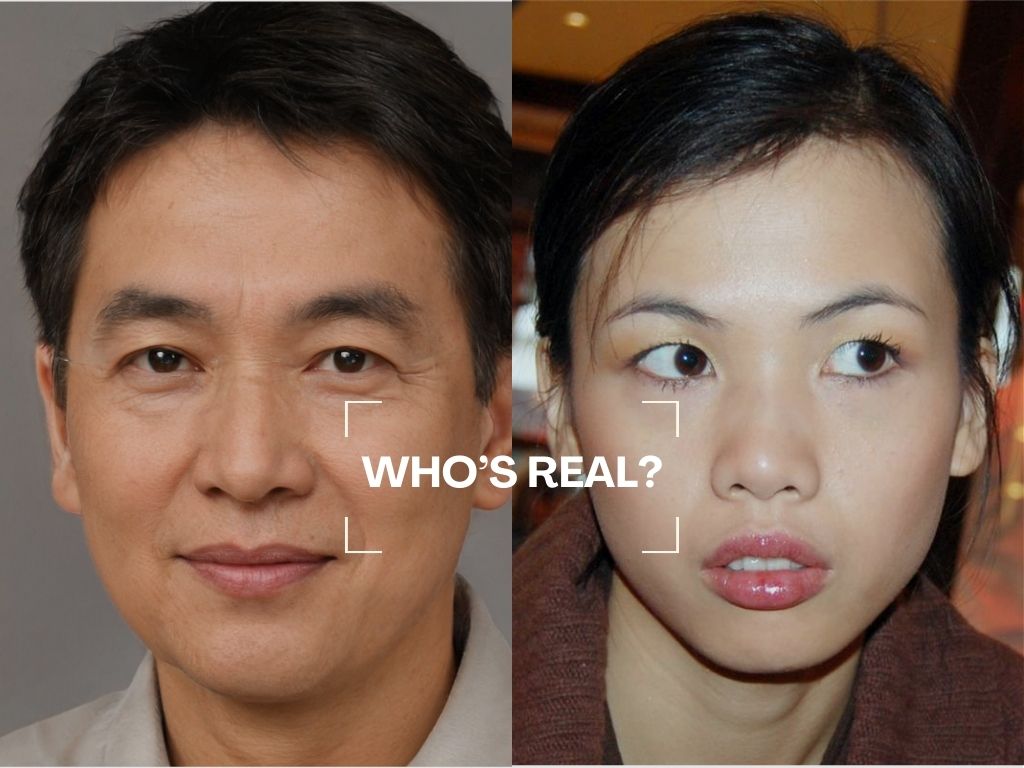
“Most deepfake detectors today work well on face-swapping and face reenactment,” says Ghorbel, “but they don’t work at all on fully synthetic data. This is the level of generalisation that we would like to reach,” she explains.
To tackle this issue, the CVI2 team turned to multitask learning – a method that trains AI to evaluate multiple aspects of an image or video simultaneously. Instead of just classifying content as simply real or fake, the model examines multiple aspects of the image, such as identifying the location of forgeries,” providing additional context to evaluate their authenticity.
The Real-World Impact of Deepfake Detection
Deepfake detection has numerous applications, with bank fraud and fake news representing some of the greatest risks to society. Fake images are also used for blackmail, coercion, and insurance fraud. As digitalisation grows, these criminal activities could slow down many sectors. The impact could be significant.
As AI grows stronger, fraudsters get better tools. This makes strong detection methods, and these research projects, crucial to protect businesses and people.
Conclusion: Staying Ahead in the AI Arms Race
The battle against deepfakes is a race against time and against rapidly evolving technology. Every breakthrough in generative AI demands an equally innovative defence strategy. “As AI evolves, so must our defences,” says Ghorbel. “Research like ours is essential to ensure the safety and trustworthiness of our digital world.”
By pushing the boundaries of AI-driven detection, researchers help build a future where digital deception no longer poses an unchecked threat. Their work helps us tell fact from fiction in our digital world. This keeps trust at the heart of our tech future.
—
Computer Vision, Imaging and Machine Intelligence (CVI2) conducts research in real-world applications of computer vision, image analysis, and machine intelligence, with extensive development of AI approaches. The expertise of CVI2 spans all stages of computer vision, including acquisition, processing, analysis, and decision.
Who’s real? Did you figure out which photograph, left or right, is a deepfake? It’s the one on the left!