Researchers at SnT developed a dual-camera system combining visual and thermal imaging to improve autonomous satellite rendezvous in space.
Satellites must connect in space to repair craft and remove debris. Using a dual-camera system and AI, researchers at SnT have found a way to make these orbital rendezvous safer and more autonomous. Their research not only solved a key challenge for the space industry but also led to the creation of a new company that makes space system testing more accessible and affordable.
The challenge of autonomous satellite rendezvous
Space is getting crowded. Satellites need to meet up to perform maintenance and repairs, and there is an increasing need to clean up debris. All this demands exact navigation. That’s why spacecraft are equipped with cameras that guide them to their targets, which start as distant points of light. As satellites move closer, tracking becomes difficult: the sun’s position changes quickly in orbit, creating extreme shifts between light and shadow that affect visibility.
The International Space Station demonstrates these challenges through its regular spacecraft visits. It receives about 8-10 spacecraft per year for crew rotations and supplies. Yet missions to repair satellites in orbit remain rare. Each approach requires detailed programming specific to that target satellite. This makes operations slow and limits what we can do in space. Automated systems would make these operations more efficient.
A multi-modal sensing solution: visual cameras and thermal imaging
In the MEET-A project, researchers at SnT partnered up with LMO, a company based in Luxembourg that developed space observation technology. This successful collaboration paved the way for numerous follow-up projects.
Mohamed Ali, Vincent Gaudillière, and Arun Rathinam developed a solution using two types of cameras. Standard visual cameras work in normal lighting, while thermal cameras can track objects in low lighting conditions. Their multimodal system fuses data from both sources for better navigation.
The project achieved three key objectives. First, they enhanced spacecraft recognition. Before calculating position, the sensing system must identify the target accurately to ensure successful rendezvous. The team developed new detection algorithms that perform better than traditional methods at recognizing different types of spacecraft and debris.
Second, they improved tracking accuracy. The system uses knowledge about orbital paths to predict movement. Since spacecraft follow specific trajectories, past positions help predict future locations. This temporal knowledge makes tracking more reliable than systems that treat each image independently.
‟ Using both thermal and standard cameras gave us a significant advantage in tracking spacecraft”
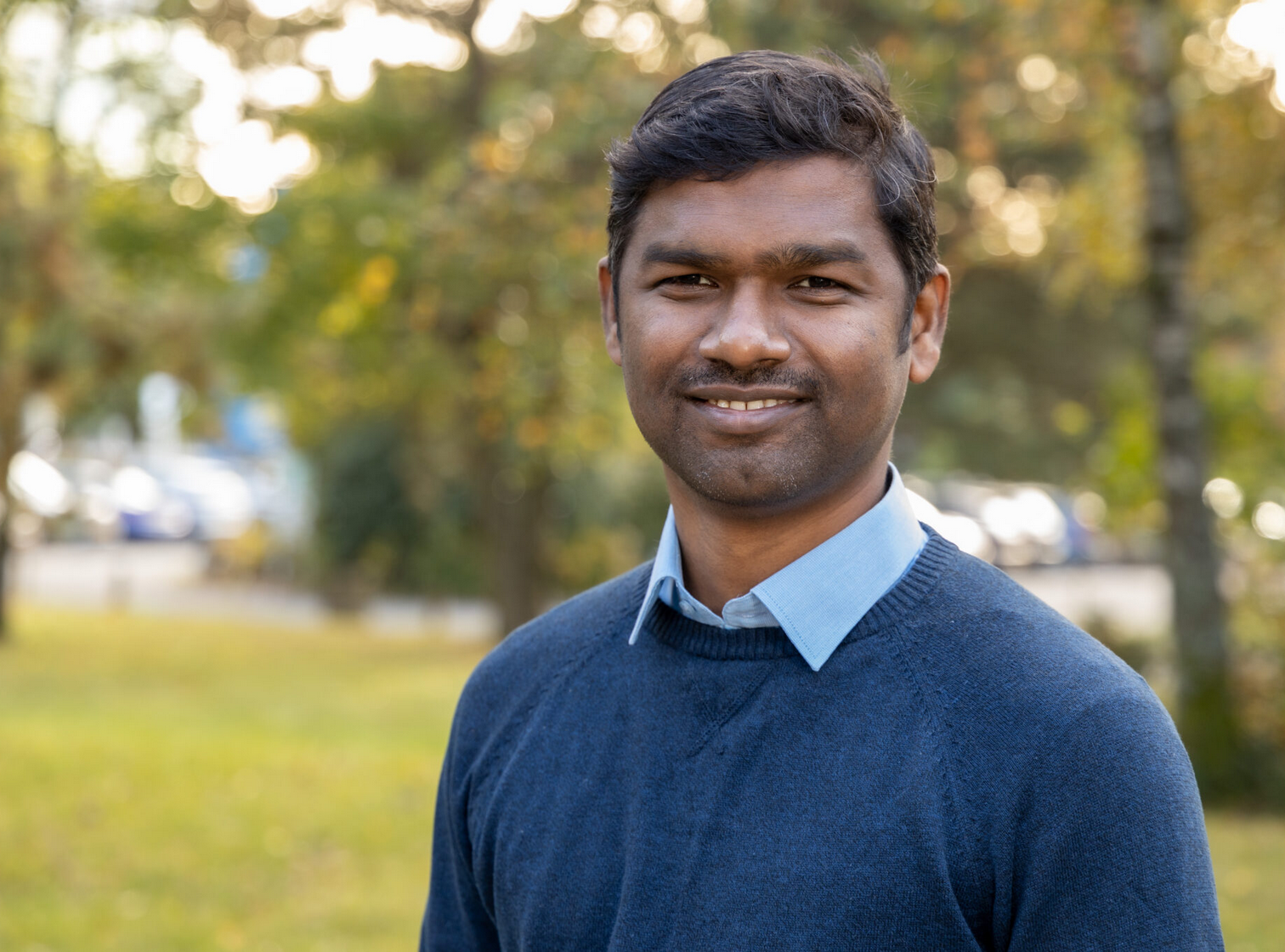
Postdoctoral researcher
Third, they improved the feature extraction and data fusion from both camera types: the multimodal system combines the best data from each source. “Using both thermal and standard cameras gave us a significant advantage in tracking spacecraft,” notes Rathinam. “While standard cameras work well in bright conditions, thermal imaging allows us to maintain tracking even in low-lighting conditions which are regularly encountered in orbit.”
Building space solutions with synthetic data
Testing space technology presents unique challenges. Getting real data from satellites thousands of kilometers away is difficult and expensive. Even when real data exists, it needs manual labeling – marking the exact position and details of objects in each image (picture number 1 below).
The team solved this by creating synthetic data – computer-generated information that mimics real conditions (picture number 2 below). Their simulator creates detailed space scenes, complete with accurate lighting and movement. The key advantage is that this data comes pre-labeled with exact positions and orientations, saving countless hours of manual work.
“Synthetic data has the advantage that you can generate as much as you want,” says Vincent Gaudillière. The metadata is generated at the same time as the digital files, making the process more efficient.
Deep learning systems need large amounts of labeled data to work well. Since most target satellites are already in orbit, it’s hard (and expensive) for researchers to collect enough real photos to train their algorithms. The simulator helps fill this gap. It creates endless variations of space scenarios, all automatically labeled.
The system trains on these simulated images before testing in SnT’s zero-gravity lab. This lab recreates space conditions, letting researchers verify their methods work with real objects. The synthetic data includes important details like position and orientation, making it valuable for training.
Taking AI research from lab to market
Research group leader Djamila Aouada created a worldwide challenge that puts research teams in competition to improve the performance of their systems and make them more robust. Teams work to figure out the exact distance between satellites and track their movement patterns. Each team receives computer-generated data to train their systems before testing on data collected in real laboratory conditions at SnT. The challenge has now reached its third year, drawing participation from scientists across the globe.
‟ ”
The team turned their research findings into a new spin-off company called WedjatAI. Their platform helps speed up the development of self-operating systems in harsh environments like space and mining sites.
“We’re using AI and coding tools to create detailed simulations that make testing safer and more affordable. Companies can now try out their systems repeatedly in simulated environments instead of learning through actual space missions,” explains Mohamed Ali who leads the spin-off.
The company offers enterprise-grade simulation tools that were once available only to major tech firms. By providing ready-to-use development environments, WedjatAI helps businesses of all sizes build autonomous systems faster and more efficiently.
This approach saves both time and resources, since companies no longer need to rely on expensive real-world launches for testing.
The MEET-A project made meaningful progress in both space research and industry work. It shows how combining research knowledge with real industry needs can lead to new business opportunities. The advances in space monitoring tools can benefit scientists worldwide while providing practical value to LMO and creating wider economic benefits for society.
About the partnership with LMO
LMO first joined forces with SnT in 2020, starting the MEET-A project in 2021 for three years. In 2022, SnT researchers and LMO began the DIOSSA project. This collaboration looks at deploying artificial intelligence-powered servicing capabilities in orbit with funding from the European Space Agency (ESA).
This research was conducted by a collaborative team. In addition to the researchers mentioned in this article, the project team included Djamila Aouada, Enjie Ghorbel, Miguel Ortiz del Castillo, and Leo Pauly. This research was supported by the Luxembourg National Research Fund.
About the CVI2 research group
The Computer Vision, Imaging and Machine Intelligence Research Group (CVI2) conducts research in real-world applications of computer vision, image analysis, and machine intelligence, with extensive development of AI approaches. The research group gathers close to 30 researchers.