IBIOMO: Image Informed Biomechanical Brain Tumor Forecast Modelling
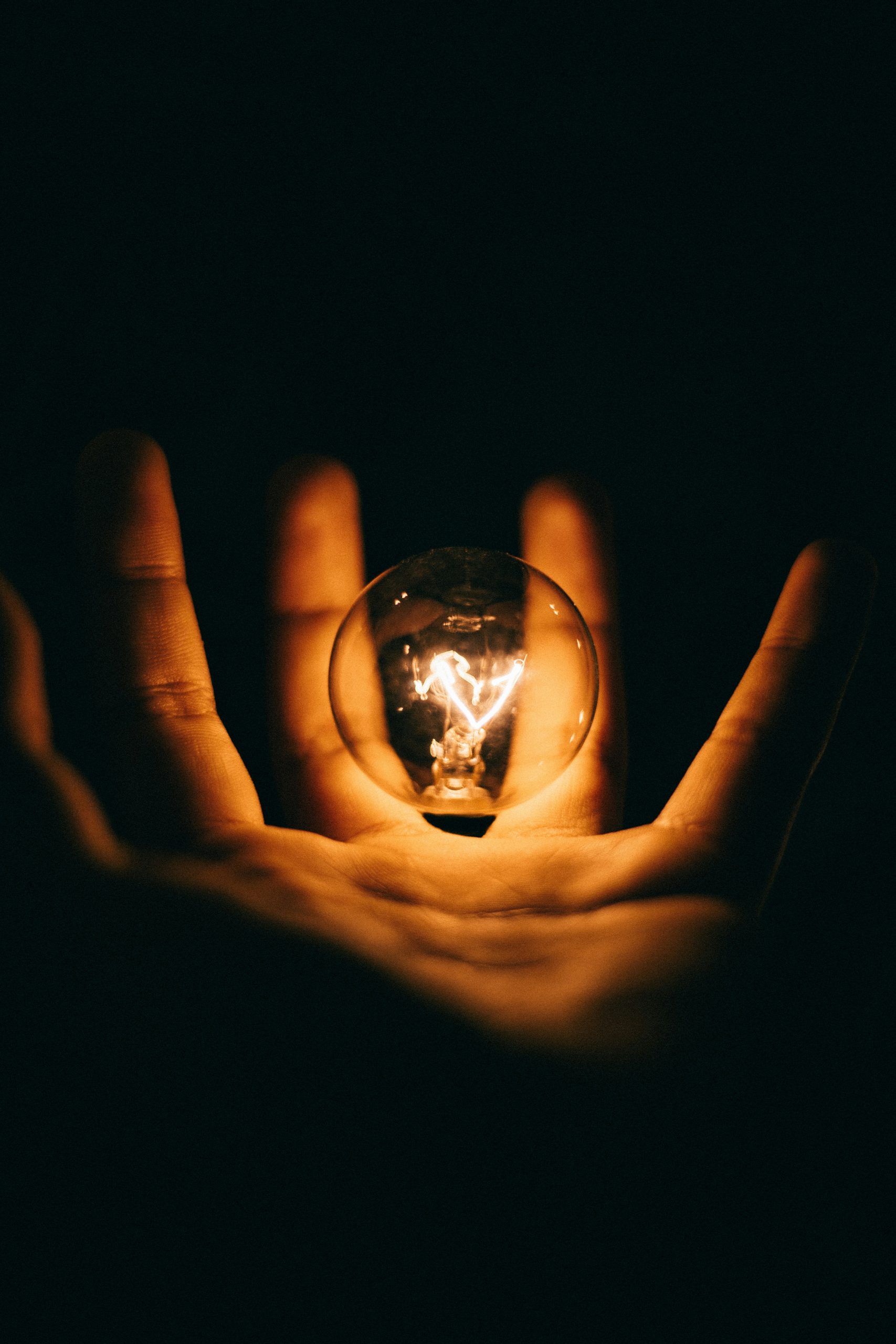
The project at a glance
About
Severe brain cancers, in particular glioblastoma, have a 50% fatality rate within 15-18 months and a 5-years survival of 5% [1]. Since 2005, the only standard of care is maximal resection surgery and concomitant radio-chemotherapy [2]. A new research area has developed, which consists in modelling the actual biophysical processes taking place within a cancerous tumour and its surrounding. This research direction is at the crossroads between clinics, mechanics, biology and computer science. Researchers develop mathematical models which they have to tailor to the patient at hand. Setting up these models has remained challenging and costly, because no generic abstraction has emerged as capable of reproducing the specific behaviour of the general patient. What is more, a (large) number of parameters is required for those models to be practically useful, yet, limited measurement modalities have been available to identify the value of those parameters, which also evolve depending on age, medication and pathologies. For those reasons, existing modelling paradigms cannot be translated from one set of patients to another, and, thus, are severely limited in terms of their practical use. Today, a dynamic young biomechanics researcher proposes to work with two world-leading teams at the interface between the clinic (in four countries and two continents), computer science, data science and computational sciences as well as biomechanics. The team has established close collaboration with the neurosurgical department and will develop image-informed mathematical modelling based on: deep-learning image segmentation and real-time simulation, multi-scale biophysical modelling of tumour growth with uncertainty quantification, inverse modelling and error control. The main deliverable will be the first stochastic, adaptive and error controlled open-source, open-data, open-protocol modelling and simulation approach to personalised brain cancer treatment, demonstrated in the case of meningioma. The adaptation of models to an individual patient’s morphology incorporating medical imaging data and the transportability of this modelling paradigm is our main aim. This transportability will be achieved by cross-validating models using data obtained independently of the patient set used to train the models.
Organisation and Partners
Faculty of Science, Technology and Medicine (FSTM)
Luxembourg Centre for Systems Biomedicine (LCSB)
Institute for Advanced Studies (IAS)