A journal publication has just been released based on AUDACITY’s PIONEER project (PIs Prof. Norman Teferle and Prof. Ariane König).
By Abdul A M Nurunnabi, Researcher, Geodesy and Geospatial Engineering (GGE), Department of Engineering, FSTM, University of Luxembourg.
We and our colleagues from GGE, Department of Engineering, Institute for Advanced Studies (IAS) Luxembourg; University of Luxembourg, The University of Tokyo, New York University and University of Waterloo have developed a method for detection and segmentation of Pole Like Objects (PLOs) in mobile laser scanning point clouds. Here is the open-access peer-reviewed paper that was presented in the ISPRS Geospatial Week 2023 held in Cairo, Egypt, and published later in the ISPRS-Archives.
Pole-like object (PLO) detection and segmentation are important in many applications, such as 3D city modelling, urban planning, road assets monitoring, intelligent transportation, road safety, and forest monitoring. Arguably, vehicle-based mobile lasers canning (MLS) is the best on-road data acquisition system, because it is fast, precise and non-invasive. As part of that, laser scanning georeferenced data (i.e., point clouds) provide detailed structural morphology of the scanned objects. However, point clouds are not free from outliers and noise. Critically, many of the object extraction methods that depend on local saliency features (e.g., normals)-based segmentation use principal component analysis (PCA). PCA can provide the local features but struggle to produce robust results in the presence of outliers and noise (see ScienceDirect publication).
To reduce the influence of outliers for saliency features estimation and in segmentation, this paper employs robust distance-based Diagnostic PCA (RD-PCA, see ScienceDirect publication) coupled with the well-known DBSCAN clustering algorithm. This study contributes to a better understanding of object detection and segmentation by (i) exploring the problems of local saliency features estimation in the presence of outliers and noise; (ii) understanding problems with PCA and why RDPCA is important; and (iii) introducing a novel method for PLOs detection and segmentation following a robust segmentation approach (e.g., publication on ieeexplore.ieee.org). The performance of the new algorithm is demonstrated through MLS point cloud data as it shows in the figure.
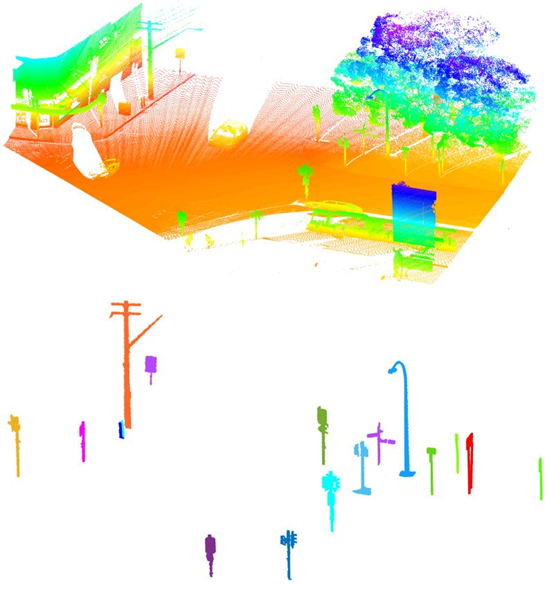
If you want to know more about the project, do not hesitate to go to the paragraph dedicated to the project PIONEER in the Audacity – University of Luxembourg I Uni.lu (Audacity 2022).