Approaching Quantum Mechanical Accuracy for Drug-Protein Binding with Machine Learning (AQMA)
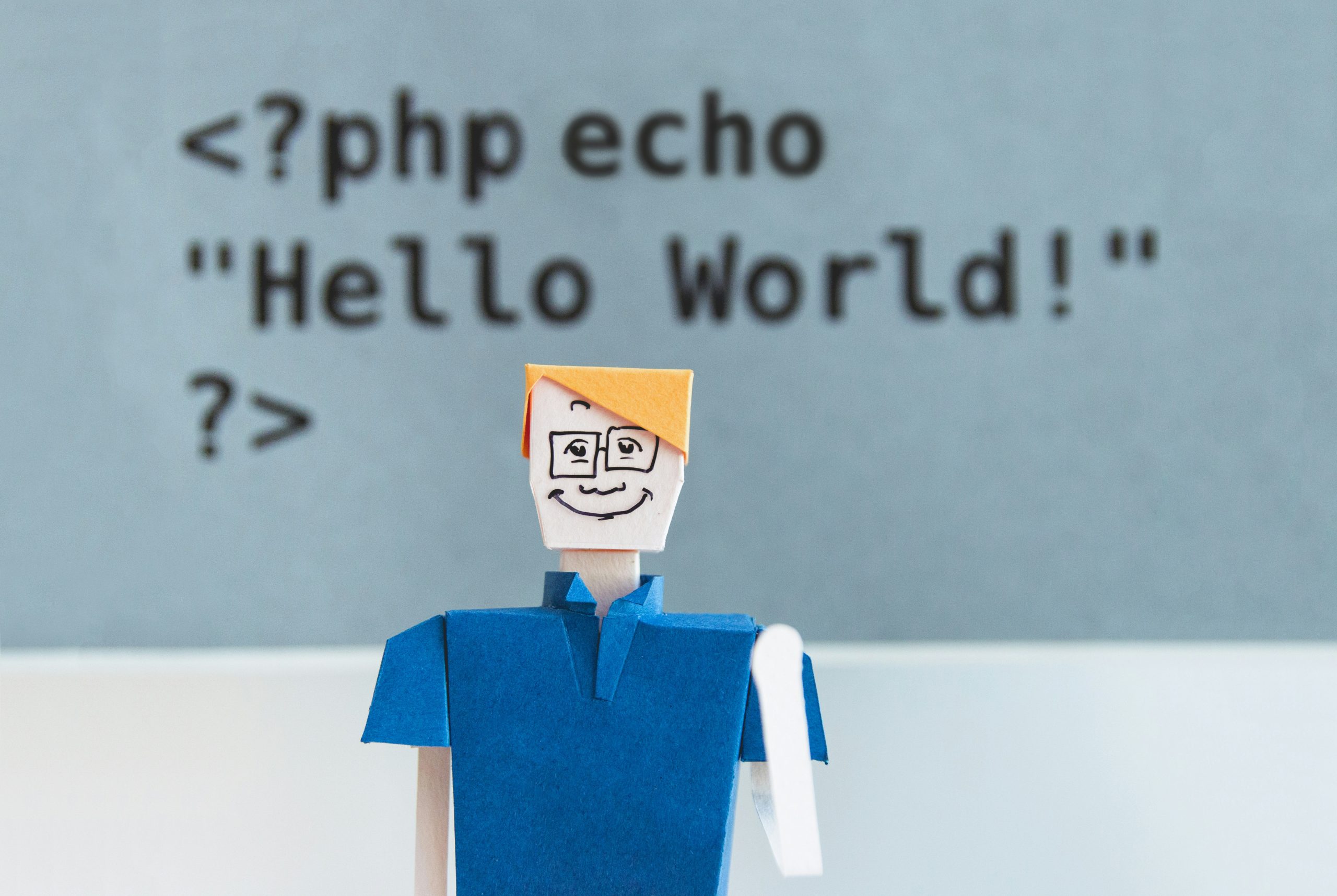
The project at a glance
About
Computational modelling of drug-protein binding is of vital importance in the modern drug discovery pipeline as it mitigates the cost, time, and resources required to screen novel candidates for biological targets, genetic studies, and gene technology. The challenge in these investigations is two-fold: (1) even slight inaccuracies of 1 kcal/mol in the prediction of energetics in biological systems can lead to erroneous conclusions, (2) the large size of drug-protein systems means that the use of highly accurate quantum-mechanical (QM) methods is not viable. Hence, it is a grand challenge to develop efficient biomolecular force fields that can achieve and exceed stringent accuracy requirements while being efficient enough for modeling drug-protein binding in atomistic detail. The recent combination of machine learning (ML) to accelerate QM calculations has already led to breakthroughs in our ability to obtain dynamical insights into small molecules. However, large realistic molecules remain out of reach of QM/ML approaches. In this context, the present PhD project will combine quantum mechanics, machine learning, and the theory of intermolecular interactions to develop QM/ML approaches applicable to large biomolecular systems, focusing on drug-protein binding. The main goal will be to develop a hybrid QM/ML model for capturing ubiquitous non-covalent interactions that largely determine biomolecular processes and functions. The developed model will then be combined with existing efficient ML force fields for local chemical bonding and applied to study folding of small proteins and the interactions between drugs and their protein targets in free-energy simulations. An appropriate benchmark dataset of representative organic molecules to examine our model performance will also be generated. The aim is to reach an applicability range in system size of, e.g., the medically significant DJ-1 protein, for which our results will be compared to further calculations and experimental data from the Cell Signaling Group at the LCSB/Uni.lu.
Organisation and Partners
Faculty of Science, Technology and Medicine (FSTM)
Institute for Advanced Studies (IAS)
Project team
Assoc. Prof Alexander SKUPIN
Associate professor/Chief scientist 2 in Modelling of Biomedical Data