Programme
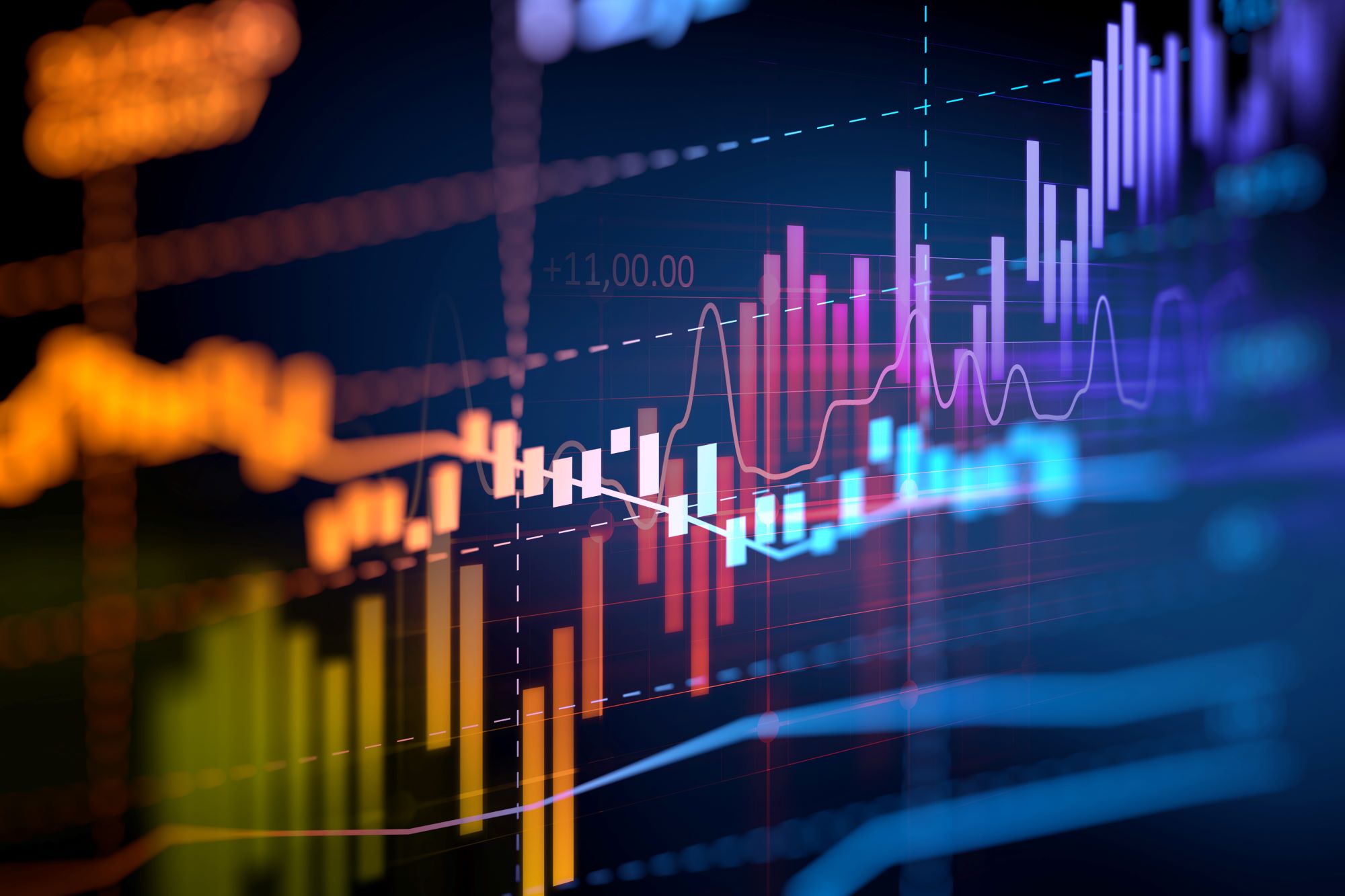
The didactical underpinning leverages on problem-based learning, enabling you to build the state-of the art scientific context around real-life cases, provided by residing industry representatives. You will also take part in an industry strength Hackathon, where you have the chance to come up with a financial service and/or with a financial product on your own ! More information below!
Attendance at second year courses is compulsory.
Academic contents
The courses of the first year are part of the main common programme and not track specific.
-
Master in Finance and Economics
Course offer for Digital Transformation in Finance, Semestre 3 (2024-2025 Winter)
-
Details
- Course title: 3.DTF1 Advanced Data Analysis
- Number of ECTS: 5
- Course code: MSFE_2_DIGTRANS-1
- Module(s): Module 3.DTF: Specialisation – Digital Transformation in Finance Track
- Language: EN
- Mandatory: Yes
-
Objectives
On completion of the course unit successful students will be able to :
Exhibit a comprehensive and multi-dimensional understanding of data, encompassing its diverse applications and significance in various contexts
Employ basic algorithmisation and scripting techniques to connect financial challenges with technology-driven solutions in professional environments
Utilise automated toolsets for data handling and management, enabling effective decision-making in tackling intricate problem sets with incomplete or noisy data -
Description
This course equips the students with the essential tools and expertise to thrive in the modern data-driven era. Its comprehensive program is divided into three building blocks, each focusing on critical aspects of data analysis. They enable the students to master Python programming, machine learning techniques, and database management with SQL.The “Python” block helps students to foster their programming skills. From basic syntax to advanced data manipulation, the students explore how Python serves as the backbone of data analysis. The block dives into libraries like NumPy and Pandas to efficiently process and visualize data, before moving to more advanced analytical tasks.The “Machine Learning” block provides a comprehensive introduction to machine learning techniques, among which different text analysis tools like ChatGPT. This block illustrates with concrete examples how these techniques are relevant from both financial and computer science perspectives.The “SQL” block takes a chapter from the Data Science course in the Master in Mathematics (specialising in Financial Mathematics) to enable students to manipulate big databases. The SQL management system is particularly useful in handling and retrieve information from structured data. -
Assessment
80% group presentation
20% individual assignment -
Note
Literature will be provided during the lecture.
-
Details
- Course title: 3.DTF2 Blockchain and Cryptocurrency Markets
- Number of ECTS: 5
- Course code: MSFE_2_DIGTRANS-2
- Module(s): Module 3.DTF: Specialisation – Digital Transformation in Finance Track
- Language: EN
- Mandatory: Yes
-
Objectives
On completion of the course unit successful students will be able to :
Understand the fundamentals of crypto-assets and blockchain technology
Improve the analysis of digital transformation across different domains and viewpoints
Steer the feasible digital transformation of the finance industry
Analyse valuation, opportunities and possible risks of a new asset class based on blockchain technology
Recognise misconceptions about technical properties of blockchain and decentralised digital identities
Identify appropriate use cases for both technologies and the corresponding role of digital wallets
Analyse blockchain technology’s influence on investment banks applying smart contracts for traditional investments -
Course learning outcomes
-
Description
This course is designed to provide participants with a comprehensive understanding of crypto-assets as a new asset class. Decentralisation technologies have garnered significant attention as a disruptive force that could challenge traditional structures and business models, particularly in the financial sector. In response to this transformative landscape, financial professionals must comprehend the potential, applications, and challenges these technologies pose. This course equips students with a critical perspective, essential to navigate the rapidly evolving financial landscape with confidence and make informed decisions in their professional pursuits. The first part of the course focuses on the fundamentals of crypto-assets and blockchain technology, from a financial markets perspective. Participants explore the core concepts of cryptocurrencies, blockchain, and distributed ledger technology, understanding the mechanics of hash functions, hash pointers, and consensus mechanisms to understand how to assess crypto-asset value and risks in crypto markets. By introducing a socio-technical perspective, the second part of the course delves deeper into decentralized finance and information security, thereby equipping financial professional with a holistic understanding of the interplay of the technical system as well as the social system. More concretely, students will learn how to reason about technology not only from a technical perspective (e.g., the building blocks of blockchain) but also from a social perspective (e.g., considering regulatory requirements). Additionally, the students will deepen their knowledge on cybersecurity management in decentralized finance, overviewing the corresponding role of artificial intelligence and privacy-enhancing technologies. They will further explore how blockchain can aid or hamper financial crime and integrity. Finally, they will be introduced to interdisciplinary design of central bank digital currencies, including the role of digital wallets. The purpose is to look beyond the blockchain hype by conducting an in-depth analysis of the factors influencing risks in decentralization technologies. This provides a balanced picture of the impact of blockchain-based technology stacks on end-users and other stakeholders.The third part of the course focuses on the institutional adoption of blockchain technology. Students explore the potential of smart contracts for investment banks in managing traditional investments. By studying a practical case, they will convert traditional financial products into smart-contract instruments. -
Assessment
35% written exam : Multiple choice and open questions on first part (25%) and second part (10%)
25% group presentation on first part
25% group presentation on second part
15% group prensentation on third part -
Note
Literature will be provided during the lecture.
-
Details
- Course title: 3.DTF3 Financial Institutions and Fintech
- Number of ECTS: 5
- Course code: MSFE_2_DIGTRANS-3
- Module(s): Module 3.DTF: Specialisation – Digital Transformation in Finance Track
- Language: EN
- Mandatory: Yes
-
Objectives
On completion of the course unit successful students will be able to :Understand the intricate relationship between financial institutions and fintech innovationAnticipate and navigate the collaborative potential and transformative consequences of fintech advancements in the financial sectorEvaluate the evolving landscape of digital currencies and their potential impact on the financial systemUnderstand fintech’s role in reshaping payments, open banking, and retail savings and investmentsHave a broader understanding about how emergent financial technologies are changing the standard financial architecture and its implications for financial institutionsHave a better understanding of how emergent fintech developments will change the financial products availableAssess credit scoring and digital lending within the context of financial innovation
-
Course learning outcomes
-
Description
The intricate interplay between financial institutions and fintech innovation has become essential in shaping the future of the financial sector. This course offers a comprehensive analysis of this relationship, highlighting the collaborative potential and far-reaching consequences. Students explore the evolving concept of currency and central bank digital currencies, the historical evolution of fintech and its role in reshaping payments and open banking, fintech’s potential to revolutionise retail savings and investments, the way banking is likely to adapt with new operating models, credit scoring, and digital lending.Students analyse the fundamental concept of money and gain a profound understanding of its evolving nature and use for financial transactions. They learn about central bank digital currencies, unravelling their precise definitions and evaluating the potential repercussions for the financial system. Moreover, participants examine the European Central Bank’s (ECB) reports, extensive studies, and cutting-edge advancements, gaining insights into the current state of affairs.This course also introduces the concept of fintech, its historical evolution, and its impact on the payments system. Participants study the main business models and their evolution and analyse open banking and API applications. Finally, an expert from the industry provides an insider’s perspective on how fintech could revolutionise retail savings and investments.Moreover, the course provides insights over the transformation that the banking system will be experiencing over the next decades. It classifies the main drivers of transformation and singles out major digitalization challenges. Students will examine the use cases of the most promising technologies like Decentralized Ledger Technologies or Generative AI. Finally, the course adopts an academic approach to credit scoring and digital lending. In this part, students explore the credit allocation to SMEs and default rates, and they investigate whether financial institutions will change their approach following the adoption of financial innovations. -
Assessment
30% written exam: Money and digital currencies30% oral exam: The future operating models in banking30% individual problem set: Credit scoring and digital lending10% individual problem set: API -
Note
Literature will be provided during the lecture.
-
Details
- Course title: 3.DTF4 Digital Finance Law, Regulation and Implications
- Number of ECTS: 5
- Course code: MSFE_2_DIGTRANS-4
- Module(s): Module 3.DTF: Specialisation – Digital Transformation in Finance Track
- Language: EN
- Mandatory: Yes
-
Course learning outcomes
By following this course, students shall be able to:Demonstrate up-to date, applicable knowledge regarding the impact and implications of the changing regulatory landscape on financial markets, instruments and institutionsApply the study content to address, explore and analyse both socio-economic and compliance implications of digital transformation initiatives in the workplace -
Description
The Course “Digital Finance Law, Regulation and Implications” is inspired by the constantly changing regulatory landscape of the European Financial Market. The more sensitive and inclusive regulation is becoming toward digitalisation; the more important it is for financial professionals to comprehend important regulatory requirements and to position digitalisation optimally in the workplace. The course therefore operates with the following learning objectives:What is special about Fintech law and regulation? Regulatory implications of digitalisation. Changing regulatory landscape. Implications of regulatory changes on an individual/institutional level.EU Digital Finance package.Regulatory approaches to innovation (such as regulatory sandboxes, innovation hubs, piloting schemes).Technology as regulatory risk.Digital Identiy, Know-your-customer, eKYC.Regulation of crypto-assets.Regulation of (Digital) Payment Systems (including Digital Currencies, CBDCs).BigTech in Finance.Funds & FinTech.RegTech & e-compliance. -
Assessment
100% written exam : computer-based, in-class, at the end of the last session
-
Details
- Course title: 3.DTF5 Financial Services: Applications
- Number of ECTS: 5
- Course code: MSFE_2_DIGTRANS-7
- Module(s): Module 3.DTF: Specialisation – Digital Transformation in Finance Track
- Language: EN
- Mandatory: Yes
-
Objectives
On completion of the course unit successful students will be able to: Apply design tools to assess the feasibility of innovative service ideas before implementationPresent business ideas with all the related operational, legal, and technical aspectsGet familiar with the concepts around data and the role of data monetisation for a successful transformation journey Obtain a realistic view of the opportunities, risks, and limitations of the machine-cognitive technologyUnderstand Artificial Intelligence technologies and their practical utilisation in financial servicesExercise on a hands-on Fraud Detection and Prevention paradigm derived from real market request
-
Description
Financial services institutions are going through a massive digital transformation exploiting the potentials that Big Data and decentralisation technologies bring along. In response to this transformative landscape, financial professionals must comprehend the potential, applications, and challenges these technologies pose. This course is structured into two part. The first part emphasises service design, enabling students to leverage emerging technologies to address user needs in real-world scenarios. The second part focuses on the business, legal, operations, and technology aspects of digital transformation in financial services.The first part of the course will equip students with knowledge and skills in service design. They will learn how to identify user needs, map user journeys, and design intuitive and user-friendly interfaces. Service design principles will be explored to ensure the technology solutions align with the end-user requirements and expectations, enhancing user adoption and overall user experience. Moreover, students will have the unique opportunity to participate in a Hackathon project with a global financial service institution. During this hands-on experience, students will collaborate in teams to ideate, design, and prototype innovative solutions to address real-world financial challenges. Under the mentorship of industry experts, students will gain practical insights into overcoming challenges, refining their ideas, and presenting their proposals effectively.The second part starts by stressing the potentials that data may bring to organizations. It touches upon data monetization strategies and exemplifies novel data-driven business models in a rapidly increasing data economy. As Big Data and Artificial Intelligence (AI) technologies introduce new risks and challenges for traditional financial players, third-party dependency risks for technology integration, data protection risks, and increasing regulatory requirements set the scene. Another inherent matter of AI is its idiosyncratic nature that makes it heavily data-driven, limiting its scalability and reusability. Students will open up the black-box of AI, discussing the technology’s genesis and following evolution while highlighting the fundamentals. The opportunities, challenges, limitations, and vulnerabilities will be scrutinized accordingly, together with the regulatory challenges and ethical implications. The lectures will raise considerations and provide examples in which modern technology compromised privacy on anonymized data and provide suggestions to mitigate such risks. Finally, students will have the chance to design a Fraud Detector simulating a realistic request from a bank. They will go through a design thinking process (understand, define, ideate, prototype, and test), deep diving into a fraudster’s behavior, deal with the data, or the lack of it as well as the integration and operating model of a Fraud Detector in a bank. -
Assessment
40% group presentation: Hackathon project in the first part
10% group report: Report with summary of hackathon in the first part
30% group project in the second part
20% individual m
aterial on the second part -
Note
Literature for the first part will be provided during the lecture.
Literature for the second part:
S. Gupta, Driving Digital Strategy, Harvard Business Review Press, 2018
T. Davenport, J. Harris, Competing on Analytics, Harvard Business Review Press, 2017
Artificial Intelligence: Opportunities, Risks and Recommendations for the Financial Sector
, CSSF, 2018
Report on Big Data and Advanced Analytics
, EBA, 2020
DAMA-DMBOK: Data Management Body of Knowledge
(2nd Edition), 2017
V. Mayer- Schönberger, Big Data: A Revolution That Will Transform How We Live, Work, and Think, 2014
-
Details
- Course title: 3.E1.Behavioural Finance
- Number of ECTS: 5
- Course code: MScFE_BK-6
- Module(s): Module 3.E: Special Topics in Finance & Economics – Electives II
- Language: EN
- Mandatory: No
-
Objectives
On completion of the course unit successful students will be able to :Know anomalies in finance and economics and understand theoretical explanationsKnow the foundations of behavioural finance and behavioural economicsUnderstand human behaviour
-
Description
The course: introduces to the literature on the anomalies in financial markets and the theories of behavioral economics and finance. Departing from the standard paradigm in financial economics, expected utility and the capital asset pricing model, we pinpoint anomalies that show up in the data from the real world and the laboratory.includes models of prospect theory, noise trader risks, psychological game theory, bounded rationality that help to understand the divergence between observed behavior and the standard paradigm of financial economics. -
Assessment
Written exam (2 hours) -
Note
Literature: Barberis and Thaler 2003. “A survey of behavioral finance.” Handbook of the Economics of Finance, 1, 1053-1128Dhami, Sanjit, 2016, Foundations of behavioral economic analysis. Oxford University PressGigerenzer and Selten (Eds.) 2002. Bounded rationality: The adaptive toolbox. MIT pressHens, Thorsten and Kremena Bachmann, 2009, Behavioral Finance for Private Banking, WileyKahneman and Tversky 1979 “Prospect Theory: An Analysis of Decision under Risk” EconometricaShleifer Andrei, 2001, Inefficient markets – An introduction to behavioral finance. Calderon Lectures in EconomicsThaler 1985 “Mental accounting and consumer choice”. Marketing science, 4(3), 199-214Thaler 1999 “Mental accounting matters”. Journal of Behavioral decision making, 12(3), 183-206Thaler and Johnson 1990 “Gambling with the house money and trying to break even”. Management science 36, 643-660Tversky and Kahneman 1992 “Advances in prospect theory”. Journal of Risk and uncertainty, 5(4), 297-323Further readings will be communicated during the lectures.
-
Details
- Course title: 3.E2.Household Finance and Real Estate
- Number of ECTS: 5
- Course code: MScFE_BK-7
- Module(s): Module 3.E: Special Topics in Finance & Economics – Electives II
- Language: EN
- Mandatory: No
-
Objectives
On completion of the course unit successful students will be able to :Carry out their original empirical work, do the critical reading of pertinent articles related to the question and know how to handle complex survey data.Carry out their original empirical work, do the critical reading of pertinent articles related to the question and know how to handle complex survey data.Critically assess the individual and social benefits of important financial products accessible to consumers.Approach consumer financial markets with more empathy towards potential customers and design better functioning products and markets.Orient themselves in the real world of household financial savings, debt and investments, up to current developments.
-
Description
The objective of the course is to understand how households use financial instruments to attain their objectives. We first review the empirical facts on household wealth and inequality as well as the underlying rational and behavioral aspects of consumer financial decision making. We study current household financial products and the competitive landscape in credit, investment, and advising markets. We also cover consumer financial product innovations and the regulation of household finance, and provides an overview of recent research on real estate markets. The course includes a group project, where students will apply the concepts seen in class and make a presentation. We introduce various data sources on household finance, their advantages and disadvantages. We guide students in how to prepare their dataset for their own project in several dimensions (e.g. weighting and imputation) and in how to apply basic estimation techniques on household surveys with a complex sample design. -
Assessment
Oral exam (40%)Presentation (40%)Seminar paper (20%) -
Note
A comprehensive reading list of recent academic publications and working papers is available from the instructors.
-
Details
- Course title: 3.E3.Financial Engineering
- Number of ECTS: 5
- Course code: MScFE_BK-8
- Module(s): Module 3.E: Special Topics in Finance & Economics – Electives II
- Language: EN
- Mandatory: No
-
Objectives
On completion of the course unit successful students will be able to :Show proficiency in probability and statistics, calculus, programming and use these tools to model markets and drive decision makingUnderstand risk and analyze financial dataDesign and implement complex financial models that allow financial firms to price and trade securitiesUnderstand the current academic and practitioner literature on financial engineeringGet exposed to some of the most applicable machine learning techniques in finance considering that machine learning and Artificial Intelligence (AI) play a significant role in the creation of models
-
Description
Understanding risk and analysing data to drive policy and decision making is the name of the game in institutions like i.e. banks, insurance companies, hedge funds, and governments.Financial Engineering is the study of applying math, statistics, computer science, economic theory, and other quantitative methods to analysing and modelling financial markets. Financial engineers work at the intersection between data science and finance. The first financial engineers were Fischer Black, Robert Merton, and Myron Scholes, infamous for their options pricing model known as the Black-Scholes Model. This model won the Nobel prize in economics and is the foundation for the explosion in derivative markets. -
Assessment
The final grade of the course will be derived from the grade for participation (25%), the presentation (25%) and the grade for the research paper (50%). -
Note
Literature: “Statistics and Data Analysis for Financial Engineering”, 2nd edition by David Ruppert and David S. Matteson, Springer, ISBN 978-1-4939-2614-5 (eBook) Research papers
-
Details
- Course title: 3.E4.Professional seminars
- Number of ECTS: 5
- Course code: MScFE_BK-9
- Module(s): Module 3.E: Special Topics in Finance & Economics – Electives II
- Language: EN
- Mandatory: No
-
Objectives
Fund Channel SA (professor: Olivier MARCY): In full autonomy, be able to select the reliable information sources, to analyze the information accuracy. Get the right information at the right moment, it is key and critical in finance.Alternative liquid investments (professor: Edoardo ANCORA): At the end of the course the students will have a complete overview of the valuation techniques applied in Luxembourg for private equity, real estate and private debt. The students will be able to become familiar with the practical aspects to perform and assess a valuation of an alternative and illiquid investment.Let’s set up an asset management business (professor: Nicolas DELDIME): Understand the strategic concerns of entrepreneurs who move to Luxembourg to do asset management. Have a holistic understanding of a regulated organizational model.
-
Description
BCEE (professors: Yves BODSON, Philippe HENNES, Yves DOMINICY)Risk Management: The first part of the lecture is an introduction to risk management answering the questions what risk is and what is risk management. The different types of risk in a bank will be introduced, as well as the Banking Union and the three lines of defense. In the second part of the lecture, we will concentrate on the credit risk, and we will be talking about credit scoring models.Asset Classes and securitization: Presentation of the primary financial asset classes (key legal and economical definitions, interaction between economic and financial cycles, valuation criteria applicable to fixed income instruments and to equities, risk categories and key measurement tools) and of the securitization market (key concepts with references to the primary financial asset classes and evolving legal and economic landscape)Corporate Banking: The presentation will address the management of the bank’s commercial relationship with corporate/professional clients, with the main theme of business financing.Fund Channel SA (professor: Olivier MARCY)Information Hierarchy (Duality between, media objectives and public targeted, versus, financial resources)Part I:Brief history of the messages and the information channels and approach of the Media theoriesNews-papers – Information hierarchyPart II:Impact of the digitalizationWeb platforms and social media – information hierarchySocial Media information hierarchy – (student presentations – 2/4 per groups) Alternative liquid investments (professor: Edoardo ANCORA)In a time of low-interest, low inflation and high turbulence in Stock Exchanges, investment managers are engaged in the hunt of higher yields like never before, starting to explore the alternative and illiquid investment space. In this context, a key skill required is the capability to properly value alternative and illiquid assets. During the course we will explore the main alternative investments (private equity, real estate, and private debt) and the typical valuation techniques applied by investment managers in Luxembourg. Let’s set up an asset management business (professor: Nicolas DELDIME)1. Asset management is a regulated profession. What does it mean to practice a regulated profession?2. Build an organizational model (governance, staffing, insourcing / outsourcing, different stakeholders)3. Build a business plan4. Review of a practical case -
Assessment
BCEE (professors: Yves BODSON, Philippe HENNES, Yves DOMINICY): 3 hours written examFund Channel SA (professor: Olivier MARCY): 20mn presentation during course periodAlternative liquid investments (professor: Edoardo ANCORA) :1 hour written examLet’s set up an asset management business (professor: Nicolas DELDIME): 1 hour written examThe final grade is the aggregation of the 4 exams’ grade (weighted / Teaching Units) -
Note
LiteratureInformation Hierarchy – Fund Channel SA (professor: Olivier MARCY):Digital Platforms :Fundchannel.comBloomberg.comMorningstar.comFundinfo.comFundsquare.netSwissFundData.chsix-group.comBooks :Media et Société (Francis Balle – Montchrestien – 7ème Edition – 2019)Culture Numérique (Dominque Cardon – Les presses de Sciences Po, Coll. « Les petites humanités » 2019)Histoire des Médias (Jacques Attali Fayard – 2021)Deux Mille Mots pour dire le Monde ( Henriette Walter – Bouquins – 2021)Histoire politique de la roue (Raphaël Meltz – Librairie Vuibert – 2020)Histoire de la Rue de l’Antiquité à nos Jours ( Danielle Tartakowsky – Tallandier 2022)Les Algorithmes font la loi (Aurélie Jean – Livre de poche – 2023)Histoire Mondiale des Impôts de l’Antiquité à nos jours (Eric Anceau, Jean-Luc Bordon Passés / Composés – 2023)No Crypto ( Nastasia Hadjadji – 2023)Technopolitique (Asma Mhalla – Seuil – 2024)Newspapers & MultiMedia Platforms :Financial Times (UK & World Wide)The New YorkerBBCThe EconomistSouth China Morning PostJeune AfriqueLes Echos (FR)L’Echo & De Tijd (BE)Handelsblatt (DE)Beaux Arts Magazine (FR)Le courrier InternationalLet’s set up an asset management business (professor: Nicolas DELDIME):Circular Commission de Surveillance du Secteur Financier 18/698
-
Details
- Course title: 3.E5 Financial Econometrics
- Number of ECTS: 5
- Course code: MScFE_BK-22
- Module(s): Module 3.E: Special Topics in Finance & Economics – Electives II
- Language: EN
- Mandatory: No
-
Objectives
Upon successful completion of this course students will be able to:Model the time varying conditional variance of time series dataApply state-of-the-art risk measurement and risk management techniquesAssess the performance of the econometric models in describing the time varying VaRCritically appraise risk management systems
-
Course learning outcomes
-
Description
Part 1 – BackgroundRisk Management and Financial ReturnsHistorical Simulations, Value-at-Risk and Expected ShortfallA Primer on Financial Time Series Analysis Part 2 – Univariate Risk ModelsVolatility Modeling Using Daily DataVolatility Modeling Using Intraday DataNonnormal Distributions Part 3 – Multivariate Risk ModelsCovariance and Correlation ModelsSimulating the Term Structure of RiskDistributions and Copulas for Integrated Risk Management Part 4 – Backtesting and Stress Testing -
Assessment
100% written exam -
Note
The reference books for this course are:Elements of Financial Risk Management, Elsevier, ed. 2, Peter F. Christoffersen, 2012Risk Management and Financial Institutions, Wiley, ed. 3, John Hull, 2012 (optional)Financial Risk Manager Handbook, Wiley, ed. 5, Philippe Jorion, 2009 (optional)
Course offer for Digital Transformation in Finance, Semestre 4 (2024-2025 Summer)
-
Details
- Course title: 4.E1.Design Execution and Evaluation of Research in Finance and Economics
- Number of ECTS: 5
- Course code: MScFE_BK-12
- Module(s): Module 4.E Special Topics in Finance and Economics – Electives III
- Language: EN
- Mandatory: No
-
Objectives
On completion of the course unit successful students will be able to:Execute an academic research project: identify a research question, position it in the context of current literature, formulate hypotheses and apply appropriate methodology to test them, present results and discuss the implications of the analyses that have been carried out; critically assess academic research and the work of peers.The course is intended to equip students with the necessary knowledge and tools to carry out independent research and prepare them for the master thesis – either academic or the research section of the applied thesis.
-
Description
The course aims at developing and strengthening students’ academic skills in identifying and analyzing research questions, applying research methods in finance and economics to approach these questions, and interpreting results relative to the current state of the art.The course is centered around a set of research projects in Finance and Economics that students carry out in small research teams. Each team focuses on an individual research project and carries it out along all its stages, from identifying a research question and motivating its relevance to choosing appropriate methodology to address it, executing the analysis, and presenting and interpreting the results.The course is structured as follows:1. Introductory session on a selected set of research topics, providing guidance on identifying each respective research question, on the appropriate research methodology and empirical strategy, the respective data to use for the empirical analysis, and related literature.2. Execution stage during which research teams plan and conduct their chosen research projects.3. Student presentation sessions at each stage of the execution of the research projects:3.1. Research idea/question, motivation behind it and current state of the art.3.2. Research methodology, empirical setup, development of hypotheses, overview of the data needed to address the research question.Execution and analysis of results, potential further areas of inquiry, conclusion. -
Assessment
60% Seminar paper30% Presentation10% Discussion -
Note
Academic articles that represent the key references for the set of research projects distributed ahead of the course.
-
Details
- Course title: 4.E2 Testing of Economic Model – Sports Data
- Number of ECTS: 5
- Course code: MScFE_BK-15
- Module(s): Module 4.E Special Topics in Finance and Economics – Electives III
- Language: EN
- Mandatory: No
-
Course learning outcomes
On completion of the course unit successful students will be able to:Formulate research questions.Use regulation/rules as a source of exogenous variation to estimate (economic) models.Test economic theory using sports data.Analyze sports data using economics principles. -
Description
Nowadays, a wealth of precise data is being collected about performances in sports. While statistical methods are designed to “summarize” the information contained in performance data, these methods do not properly take into account the fact that performances in sports are the result of rational agents (athletes, teams, coaches,…etc.) taking decisions under a set of incentives (rewards, prize money, payoffs) and constraints (rules of the game).This course introduces and applies the economic approach to human behavior on performance data. The course shows how this approach can provide not only deep insights into the analysis of performance in sports but also robust tests for economic models. Indeed, since by the very nature of sports, athletes perform in a “controlled” environment, (changes in) the “rules of the game” offer unique opportunities, natural experiments, to test predictions of economic models.The course provides a set of examples that are used as an illustration of the method. These examples relate to the following questions:Why do goalkeepers not always choose to jump to the same side at penalty kicks? Why did performance inequality increase so much in the last 5 decades in the peloton of the Tour de France?Why did the gender performance gap in triple jump, pole vault and marathon followed a S-shape evolution over a period of 25 years plateauing thereafter? Why do some tennis players and sumo wrestlers cheat? -
Assessment
2 hours written exam during the course period -
Note
PALACIOS-HUERTE, (2014), BEAUTIFUL GAME THEORY: HOW SOCCER CAN HELP ECONOMICS, PRINCETON UNIVERSITY PRESS.CANDELON, B. AND DUPUY, A. (2015): HIERARCHICAL ORGANIZATION AND PERFORMANCE INEQUALITY: EVIDENCE FROM PROFESSIONAL CYCLING, THEINTERNATIONAL ECONOMIC REVIEW, VOL. 56 (4), PP. 1207-1236.DUPUY, A. (2012): AN ECONOMIC MODEL OF THE EVOLUTION OF THE GENDER PERFORMANCE RATIO IN INDIVIDUAL SPORTS. THE INTERNATIONAL JOURNAL OF PERFORMANCE ANALYSIS IN SPORT, VOL. 12 (1), PP. 224-245.JETTER, M. AND WALKER, J. (2017): GOOD GIRL, BAD BOY: CORRUPT BEHAVIOR IN PROFESSIONAL TENNIS, SOUTHERN ECONOMIC JOURNAL, 2017, 84(1): 155-180DUGGAN, MARK, AND STEVEN D. LEVITT. (2002). “WINNING ISN’T EVERYTHING: CORRUPTION IN SUMO WRESTLING .” AMERICAN ECONOMIC REVIEW, 92 (5): 1594-1605.
-
Details
- Course title: 4.E3 Statistics in risk management using R – French
- Number of ECTS: 5
- Course code: MScFE_BK-16
- Module(s): Module 4.E Special Topics in Finance and Economics – Electives III
- Language: FR
- Mandatory: No
-
Objectives
Objectifs:Savoir manipuler le logiciel R pour des analyses de donnéesComprendre la notion de risque financier et son estimation en utilisant les fonctionnalités du logiciel RSavoir faire une analyse économétrique de séries temporelles en utilisant le logiciel R
-
Description
Le premier chapitre du cours consiste à apprendre à manipuler le logiciel R, notamment afin d’avoir accès à des données financières et de les analyser. Nous étudierons en particulier comment créer des séries temporelles, utiliser des indicateurs statistiques, faire une analyse technique et graphique à l’aide du logiciel R. Le deuxième chapitre s’intéresse au concept de risque financier d’un actif et d’un portefeuille d’actifs au travers de la notion de rendement, de volatilité, de calcul d’indicateurs de performance ajustés au risque et d’optimisation de portefeuille. Nous développerons la notion de risque au travers d’indicateurs statistiques tenant compte des risques extrêmes. Dans tous ces chapitres, les séries temporelles sont étudiées en faisant une analyse économétrique des données financières. Les applications qui illustrent le cours sont entièrement menées à partir du logiciel R. Les étudiants doivent avoir téléchargé le logiciel R avant de venir à la première séance du cours en utilisant le lien suivant : https://cran.r-project.org/bin/windows/base/ -
Assessment
Examen écrit de 2 heures pendant la période de cours -
Note
Bilbiographie :Analyse des séries temporelles, 5e édition, de R. Bourbonnais et V. Terraza, chez Dunod 2022Analyse Statistique pour la gestion bancaire et financière, applications avec R, de V. Terraza et C. Toque, chez De Boeck, 2013Modélisations de la Value at Risk – Une évaluation de l’approche Riskmetrics de V. Terraza Editions Universitaires Européennes – 2010 Le livre de R, apprentissage et référence, de B. Desgraupes, chez Vuibert, 2013Séries temporelles avec R, de Y. Aragon, chez Springer, 2011
-
Details
- Course title: 4.E4. Incubator Course: Cases of Modern Finance
- Number of ECTS: 5
- Course code: MScFE_BK-26
- Module(s): Module 4.E Special Topics in Finance and Economics – Electives III
- Language: EN
- Mandatory: No
-
Objectives
On completion of the module unit successful students will be able to:Have a broader understanding about how emergent financial technologies are changing the standard Financial Architecture and its implications for financial institutionsHave a better understanding of how emergent FinTech developments will change the financial products available
-
Description
This incubator course aims to Educate students about the disruptive effects of advanced technologies in the financial sector, with a leading focus on asset management, instruments and advanced data analytics.Raise awareness on both the socio-economic and the performance implications of innovation diffusion.Provide both academic and industry-strength underpinning for the future of finance.The incubator course consists of two parts. Each part includes both lectures lectures and practical case work in groups. The practical case works, as well as the lectures are offered by a residing institutional / industry partner of this course. Students are asked to provide solutions to the presented case studies, based on a problem-based discovery. The first block is concentrating on the notion “Financial architecture” in the context of asset management and administration. It discusses the disruptive effects of peer2peer technologies, such as blockchains, through industry-strength case studies. Below, the skeleton of this block is provided as follows:General IntroductionIntroduction to the Financial ArchitectureBusiness Models of BanksBusiness Models of the Asset Management IndustryTrading Platforms and their Market MicrostructureIntroduction to Blockchain Peer-to-peer platformsThe Blockchain Market Microstructure Business Models of FinTech Credit PlatformsRecent developments about Financial Products on Peer-to-peer platformsWorkshop: Liquidity pools and automated market makers (instrumentalising flash swaps and loans)Conclusion: A perspective on the changing Financial Architecture in light of recent technological developments.The second block is concentrating on the notion “advanced data analytics” in the context of asset management and administration. In an ever-changing environment, Investment Banks face many challenges: regulatory compliance, business model review and increased competition. At the same time, the financial sector is witnessing a huge digital transformation, particularly with the advent of new technologies. Artificial intelligence, Distributed Ledger Technology, API and Big data, how can new tools bring solutions to financial institutions?This block would kick-off with a lecture on investment banking. Through its organizational structure and its businesses, themes common to the world of banking and investment will be discussed. Case studies are introduced and discussed in order to propose a digital solution in response to the case problem at hand. Below, the skeleton of this block is provided as follows:A practical application of Performance and Risk management measures in finance Measures of returnThe complexity of defining and measuring riskHow to find the best returns while minimizing the risk (measures of risk-adjusted return and its limitations)?Can we use new technologies and Machine Learning for predicting stock price? How to observe the factors affecting assets?Multifactor Models (Fama French, Carhart four-factor model): a linear approachAn Introduction to Decision trees (and random forest): a nonlinear approachWorkshop #1: Implementation of a basic strategy with alternative data: does ESG (Environmental, Social, and Governance) investment bring better performance?Workshop #2: Compare linear and non-linear approaches in price prediction and understand the limitationsConclusion -
Assessment
The final grade will be an oral presentation (100%) -
Note
Literature:Ayadi, R., Cucinelli, D. and De Groen P. (2019) « Banking Business Models Monitor : Europe »Halaburda, H., Haeringer, G., Gans, J. and Gandal, N. (2022) “The Microeconomics of Cryptocurrencies”, Journal of Economic Perspectives forthcoming. Lehar, A. and Parlour, C. (2021) “Decentralized Exchanges”, SSRN Working Paper.Liberti, J.M. and Petersen, M.A. (2019) “Information: Hard and Soft”, The Review of Corporate Finance Studies, 8(1), 1-41.Valleee, B. and Zheng, Y. (2019) “Marketplace lending: A new banking paradigm?”, The Review of Financial Studies, 32(5), 1939-1982.Verlaine, M. (2020) “Behavioral Finance and the Architecture of the Asset Management Industry”.Stefan Jansen. (2020). Machine Learning for Algorithmic Trading: Predictive models to extract signals from market and alternative data for systematic trading strategies with Python, 2nd Edition.Chloé-Agathe Azencott. (2018). Introduction au Machine Learning.Joachim Häcker, Dietmar Ernst. (2017). Financial Modeling: An Introductory Guide to Excel and VBA Applications in Finance.Jean Dermine. (2009). Bank Valuation and Value-Based Management: Deposit and Loan Pricing, Performance Evaluation, and Risk Management.John C. Hull. (2018). Risk Management and Financial Institutions.
-
Details
- Course title: 4.E5 Topics in Well-being Research
- Number of ECTS: 5
- Course code: MScFE_BK-28
- Module(s): Module 4.E Special Topics in Finance and Economics – Electives III
- Language: EN
- Mandatory: No
-
Objectives
On completion of the course unit successful students will be able to:Analyse different datasets with the STATA softwareHave knowledge of a number of the empirical methods used to tackle theoretical questionsHave acquired advanced interdisciplinary knowledge.
-
Course learning outcomes
-
Description
In this course, students will learn about the different theoretical and conceptual approaches used in the social sciences to analyze well-being and its determinants. After an introduction to the various methods proposed for the measurement of well-being, we will focus on its determinants and explore the relationship with income (including the Easterlin paradox), social position and mobility, as well as the role of adaptation and expectations. We will then move on to discuss societal well-being and the recent proposals to go beyond GDP as a measure of progress. Last, we will show how measures of individual well-being can be used to shed new light on important economic concepts such as income inequality, gender disparities and policy evaluation. -
Assessment
Seminar Paper (95%)Active participation (5%) -
Note
Recommended literature:Balestra, C., Boarini, R. & Ruiz, N. (2018).Going beyond GDP: empirical findings, in: C. D’Ambrosio (ed.), Handbook of Research on Economic and Social Well-Being, chapter 2, pages 52-103, Edward Elgar Publishing.Blanchflower, D. G., & Oswald, A. J. (2004). Well-being over time in Britain and the USA. Journal of Public Economics, 88, 1359-1386.Blanchflower, D. G., & Oswald, A. J. (2008). Is well-being U-shaped over the life cycle? Social Science & Medicine, 66, 1733-1749.Card, D., Mas, A., Moretti, E., & Saez, E. (2012). Inequality at work: The effect of peer salaries on job satisfaction. American Economic Review, 102, 2981-3003.Clark, A. E., & Oswald, A. J. (1996). Satisfaction and comparison income. Journal of Public Economics, 61(3), 359-381.Clark, A. E., Frijters, P., & Shields, M. A. (2008). Relative income, happiness, and utility: An explanation for the Easterlin paradox and other puzzles. Journal of Economic Literature, 46, 95-144.Clark, A. E., & Lepinteur, A. (2019). The causes and consequences of early-adult unemployment: Evidence from cohort data. Journal of Economic Behavior & Organization, 166, 107-124.Clark, A. E. (2018). Four decades of the economics of happiness: Where next? Review of Income and Wealth, 64, 245-269.Di Tella, R., MacCulloch, R. J., & Oswald, A. J. (2001). Preferences over inflation and unemployment: Evidence from surveys of happiness. American Economic Review, 91, 335-341.Easterlin, R. A. (1974). Does economic growth improve the human lot? Some empirical evidence. In Nations and Households in Economic Growth. Academic Press.Flèche, S. (2021). The welfare consequences of centralization: evidence from a quasi-natural experiment in Switzerland. Review of Economics and Statistics, 103, 621-635.Giovannini, E., & Rondinella, T. (2018). Going beyond GDP: theoretical approaches, in: C. D’Ambrosio (ed.), Handbook of Research on Economic and Social Well-Being, chapter 1, pages 1-51, Edward Elgar Publishing.Guio, A.-C. (2018). Multidimensional poverty and material deprivation: empirical findings, in: C. D’Ambrosio (ed.), Handbook of Research on Economic and Social Well-Being, chapter 6, pages 171-193, Edward Elgar Publishing.Layard, R. (2006). Happiness and public policy: A challenge to the profession. Economic Journal, 116, C24-C33.Lepinteur, A. (2019). The shorter workweek and worker wellbeing: Evidence from Portugal and France. Labour Economics, 58, 204-220.Luttmer, E. F. (2005). Neighbors as negatives: Relative earnings and well-being. Quarterly Journal of Economics, 120, 963-1002.Oswald, A. J., Proto, E., & Sgroi, D. (2015). Happiness and productivity. Journal of Labor Economics, 33, 789-822.
-
Details
- Course title: 4.Applied Master Thesis (including Internship)
- Number of ECTS: 20
- Course code: MScFE_BK-19
- Module(s): Module 4.DTF.MTI Applied Master thesis & Internship
- Language: EN
- Mandatory: No
-
Details
- Course title: 4.Academic Master Thesis
- Number of ECTS: 20
- Course code: MScFE_BK-20
- Module(s): Module 4.DTF.MTI Applied Master thesis & Internship
- Language: EN
- Mandatory: No